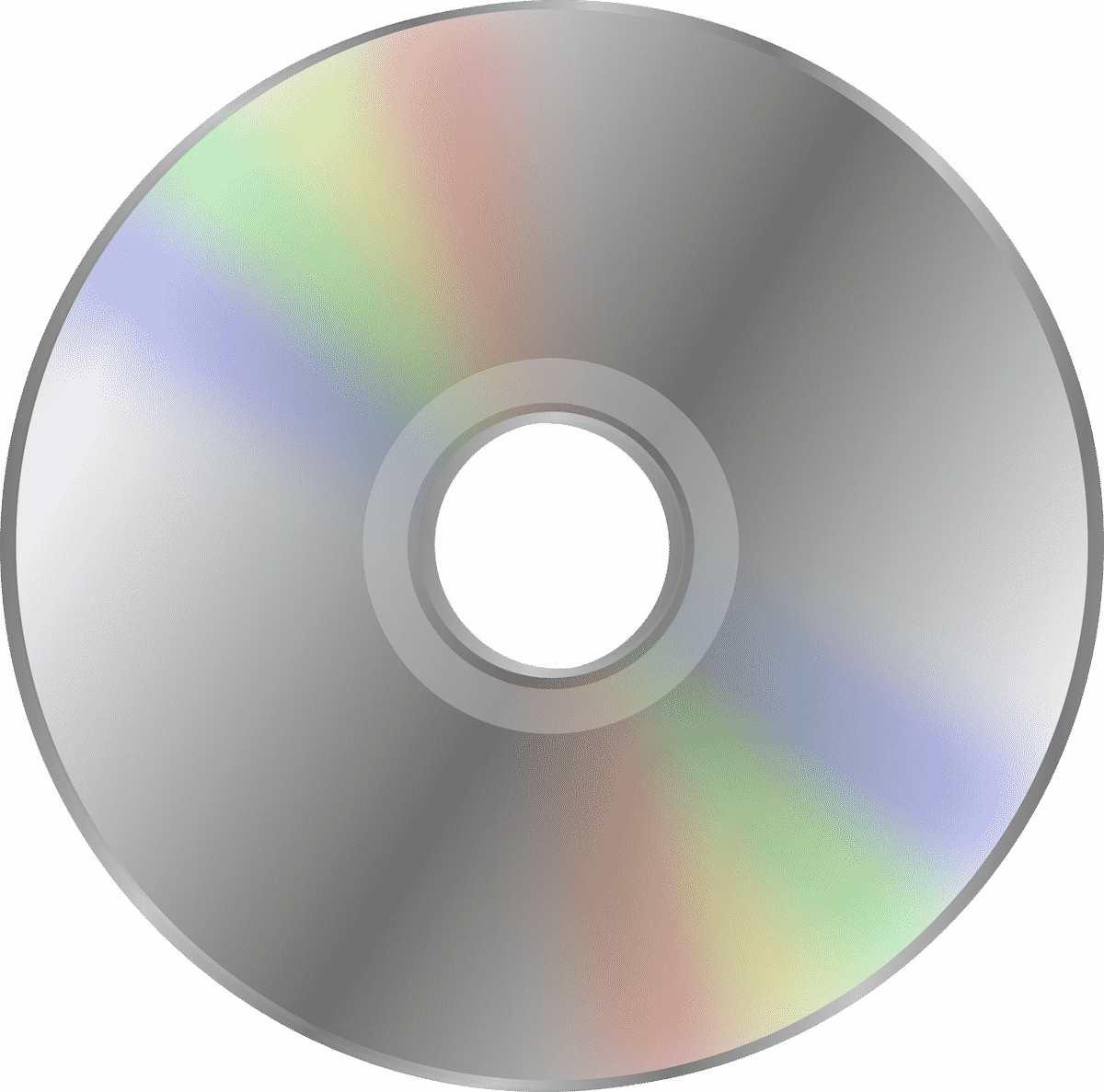
In a world where data is the new gold, we must ask ourselves what that means for our ‘pre-programmed’ knowledge. By measuring a lot, we collect data, and it seems as if we can do anything with it nowadays. The use of AI algorithms seems to be enabling us to find out everything about our behaviour, and to predict what we will be buying, where we are driving to, and whether or not our autonomous car should intervene. Does it still make sense to learn as a student? Can we download all the knowledge, and should education as well as engineering design be completely experience-based?
When I graduated in Systems and Control Engineering in 1984, I was trained to use models to analyse and understand the dynamic behaviour of systems. With these models, we could also design control systems based on models. With guarantees for optimality and robustness. Cool. I was sent out into the world to proclaim my faith: with models, it all gets better! My PhD research at KEMA in Arnhem reinforced this image. I was a researcher for the design of the first wind farm in the Netherlands, i.e. with several wind turbines together. I worked on the use of models to design automatic control of the speed and angle of the blades. All this through simulations.
Then I joined Philips and started researching the application of this new control theory for the first data application of CD players (CD-ROM). I soon learned that the product developers of Philips Optical Storage thought very differently. They used measurements from the CD scanning mechanisms to tune the controls. I thought I could do better with the modern model-based theory I had learned. And although that was a bit true, models are not perfect. The data you can measure if you can estimate the measurement errors sufficiently, is better. I learned to assess the value of experimentation, the use of data, of experimental validation. For example, by using the near-repetitive character of the tracking errors after each rotation of a CD. After all, the next track more or less resembles the previous track. When I left Philips for the university in 1999, I formulated one of my research challenges as ‘doing more with data’.
At the university that goal was converted into a research line ‘learning control’ with applications for wafer scanners, printers and other high-tech systems. The idea is that the controller learns from past mistakes. We did this by using models and data, and the better the model, the faster we could learn using the data. Sometimes with only two or three iterations.
This is exactly the core dilemma of the current hype around artificial intelligence. Namely: if you don’t use a model, and only collect data, then you need a lot of data and a lot of iterations to get a good result. While the use of a model accelerates that process very much. This insight was confirmed last year during the Holst lecture at the TU Eindhoven by one of the top people of Facebook.
The models used by the AI algorithms may well be learnt and based on data as well. In our field of systems and control, this is called system identification. Think of a person who takes driving lessons. After 50 lessons of one hour, you can probably drive more reliably than after the use of model-less trained data of a few million cars. This is because you already have some kind of model of the world around you in your head. I expect that in the coming decade data-based artificial intelligence will reap the rewards of our field of system and control technology, in order to come to a happy marriage of data and model use.
And I hope that the European headquarters of AI will be located here in Eindhoven. The combination of AI and our high-tech industry could prove to be a winner!
About this column:
In a weekly column, alternately written by Eveline van Zeeland, Jan Wouters, Katleen Gabriels, Maarten Steinbuch, Mary Fiers, Carlo van de Weijer, Lucien Engelen, Tessie Hartjes and Auke Hoekstra, Innovation Origins tries to find out what the future will look like. These columnists, occasionally supplemented with guest bloggers, are all working in their own way on solutions for the problems of our time. So tomorrow will be good. Here are all the previous episodes.