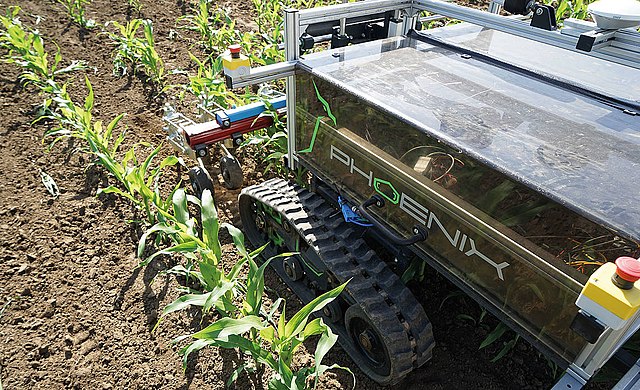
Recently I gave a presentation on digitalization in greenhouse horticulture. In a sidestep, I mentioned ‘the application of AI’ without elaborating any further.
There was a lively discussion at the end of the presentation about how far we have come in the application of digital technology and as to how the Netherlands can maintain its position as a leading horticultural country in this field. Later, one of the people present asked me to make sure that next time I would also ‘briefly’ explain what AI actually is. Yes, that is a rather difficult question, because what exactly is AI?
There are hundreds of definitions. Here are a few that I found among the hundreds of definitions:
“AI has become a catch-all term for applications that perform complex tasks that previously required human input, like online communication with customers or a game of chess.”
“AI is the ability of a machine to exhibit human-like skills – such as reasoning, learning, planning and creativity.”
The formal definition of AI by the European Commission is: “systems that display intelligent behavior by analyzing their environment and taking actions – with some degree of autonomy – to achieve specific goals.”
So I won’t dare to delve into that, nor whether there is a difference between statistics and AI. Or if many of the applications that claim to make use of AI actually do that…
What I do find interesting is the discussion about where we can apply AI. The possibilities are much more wide-ranging than initially thought when the term was coined. Moreover, the question of where we can apply AI is in fact still not that interesting. After all, it is not about the technology so much but about the value that the product or service we develop with it has for users!
Nevertheless, in order to get an idea of what applications you are able to develop, I think it helps to take a look at the seven patterns of AI.
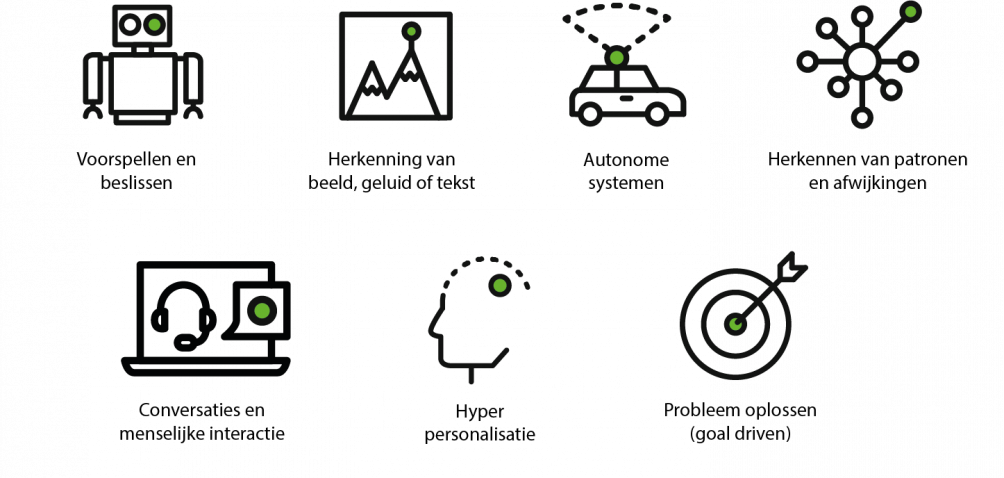
From left to right, top to bottom: Predicting and making decisions – Recognizing an image, sound or text – Autonomous systems – Recognizing patterns and anomalies – Conservation and human interaction – Hyperpersonalization – Problem-solving (goal driven)
Hyperpersonalization
Hyperpersonalization involves tailoring products or services to a specific person. The best-known example of this are personalized advertisements. An example from horticulture could be the cultivation of crops with specific ingredients tailored to the needs of a certain type of person. Think of a tomato with more lycopene aimed at a prostate cancer patient.
Autonomous systems
These systems can perform tasks without or with very minimal input from a human being. Autonomous cars such as a Tesla (although they are not yet allowed on the road without input from a person) are the best known example here. Currently in horticulture, there are several harvest robots in development that can harvest 24/7 without human intervention.
Autonomous systems are often thought of as ‘hardware’. However, software (bots) can also perform tasks autonomously.
Predicting and making decisions
This is a pattern that helps people make better decisions. Whereas autonomous systems are about making decisions with or without a minimum of input from people, this is about helping people make the right choice.
In horticulture, for example, we are seeing systems here that predict the harvest of broccoli or help the grower make choices about the optimal climate settings for a greenhouse.
Conversation and human interaction
This involves communication between machine and human in a manner that is comparable to communication between humans. An example of this are virtual assistants such as Siri or Alexa. In horticulture, the dutch company Koppert Cres started experimenting with Google assistant a few years ago to provide information on recipes and availability of their products.
Recognizing patterns and anomalies
This pattern involves looking for any anomalies. What is ‘normal’ and what is a deviation from the standard pattern. Take the detection of fraud, for one thing. Which financial transaction fits the pattern of a user and where does it deviate so much that fraud might be involved? A horticultural example is the early recognition of an abnormal patch in a crop. For instance, a harvesting assistant who drives through a rose field twice a day and can recognize from all the thousands of roses being inspected that the ripening process is always slightly slower at a particular spot. It is complicated even for a very experienced harvester to recognize these kinds of (small) deviations from such a huge amount of information.
Recognizing an image, sound or text
Uses of this pattern are perhaps the best known. Certain things are detected within a large amount of unstructured data. These can be e.g. photographs, videos, sound or (written) text.
Facial recognition when unlocking a smartphone, converting written text to a Word document and recognizing a music track are well-known examples.
Examples in horticulture include recognizing a (green) cucumber among all the green leaves. Another example is the ability to recognize products on Instagram. By combining the information from the image with the text from the accompanying message and the location where the photo was taken, the Dutch analytics consulting company Gibbs managed to make more information available about the growers’ end customers.
Problem-solving (goal driven)
This pattern revolves around finding the optimal solution by practicing trial and error. It concerns e.g. the planning issues in a factory but also how to win a game of ‘Go’. In horticulture, this pattern can help to use the best possible mix of resources such as temperature, lighting and water to get the highest yield when growing lettuce. One example is the Autonomous Greenhouse Challenge.
Multiple patterns in a product
Of course, things don’t work in real life as simply or as circumscribed as in the patterns mentioned above. Often a solution is made up of multiple patterns. First of all, for example, it is about recognizing patterns in an image which then requires examining what the patterns and anomalies are and then determining the best solution to the problem based on those anomalies.
Even with the use of patterns, explaining what AI is is still not that straightforward. The next time I am asked this question, I still don’t think I will be able to ‘briefly’ explain it!
I do hope that with these examples and the different patterns that it becomes a bit clearer as to what is possible with AI. Of course, it’s not about the technology of AI at all, but mainly about the value we can create for the end user. Technology is only a tool in that respect….
Would you like to know more about AI in the Agri and Food sector? Then take a look at the recently launched (Dutch Language) AI course for Agri and Food that I had the privilege to contribute to on the chapter about working with a robot.
About this column:
In a weekly column, alternately written by Bert Overlack, Eveline van Zeeland, Eugene Franken, Helen Kardan, Katleen Gabriels, Carina Weijma, Bernd Maier-Leppla and Colinda de Beer, Innovation Origins tries to figure out what the future will look like. These columnists, sometimes joined by guest bloggers, are all working in their own way to find solutions to the problems of our time. So tomorrow will be good. Here are all the previous articles.