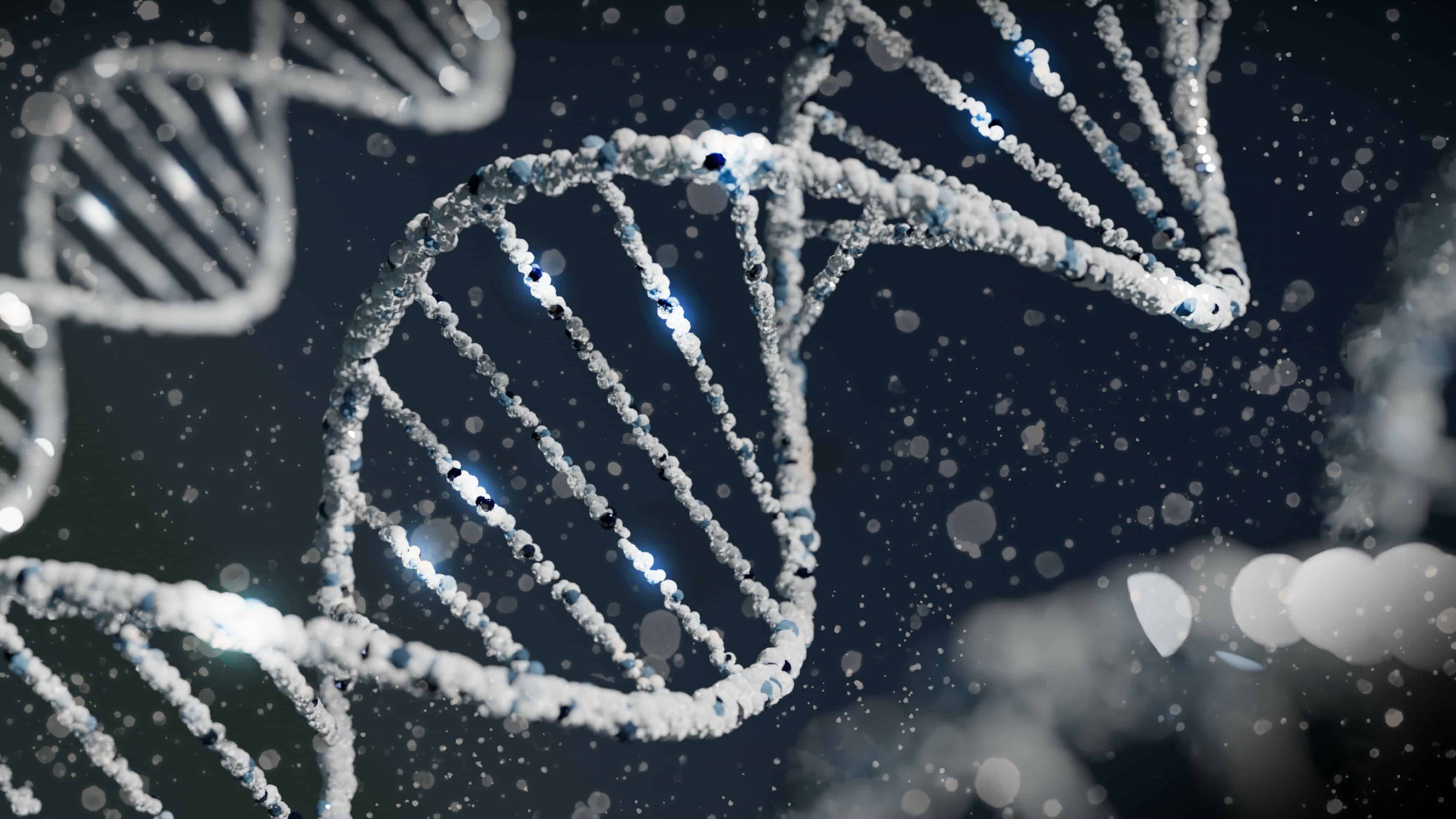
Google DeepMind’s new AI tool, AlphaMissense, predicts the impact of genetic mutations on human health, potentially revolutionizing disease diagnoses and treatment. Analyzing all 71 million possible single-letter DNA mutations, it categorizes 89 percent as likely harmless or pathogenic, outperforming human experts.
- Google DeepMind’s AI tool, AlphaMissense, classified the effects of 71 million DNA mutations.
- The AI tool classified 89 percent of the mutations as likely pathogenic or benign.
- However powerful, rigorous evaluation of the tool is needed.
While not intended for direct clinical use, AlphaMissense aids in diagnosing rare genetic disorders and discovering disease-causing genes. It outperforms existing ‘variant effect predictor’ programs, potentially identifying previously unknown harmful mutations. Despite concerns about the complexity and the need to verify its predictions, AlphaMissense could carry significant weight in future disease diagnoses if proven reliable.
The power of AlphaMissense
AlphaMissense, the new AI tool developed by Google DeepMind, is an adaptation of the company’s ground-breaking AlphaFold program. AlphaFold, originally designed to predict the 3D structure of human proteins from their chemical makeup, has now been retooled to identify potential health risks within our own genetic code. AlphaMissense leverages AlphaFold’s knowledge to pinpoint ‘missense’ mutations – genetic alterations that can disrupt the function of proteins and lead to disease.
Missense mutations occur when a single letter in our DNA code is misspelt, changing the amino acid sequence of a protein. They frequently play a role in genetic diseases such as cystic fibrosis, sickle-cell disease, and various cancers. Yet, of the 71 million possible missense mutations, human experts have only confirmed 0.1 percent as pathogenic or benign. AlphaMissense, however, has already categorized a staggering 89 percent of these mutations.
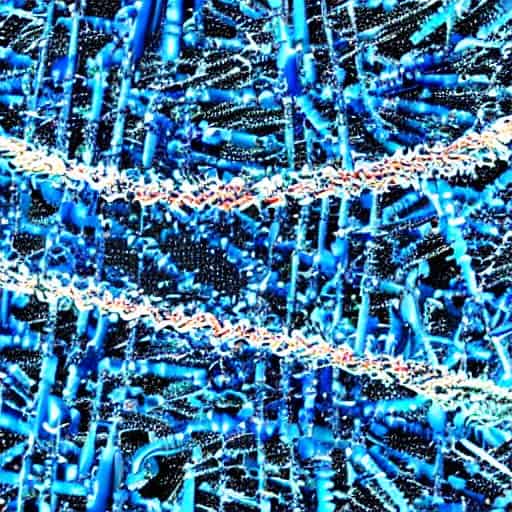
The training and validation of AlphaMissense
The training of AlphaMissense involved DNA data from humans and closely related primates. The AI familiarised itself with protein sequences to understand what a “healthy” protein looks like. It then learned to distinguish between benign and potentially harmful missense mutations.
Google DeepMind did not just rely on internal validation. The company collaborated with Genomics England to evaluate the accuracy and consistency of AlphaMissense’s predictions. The results confirmed that the tool’s predictions are not only accurate, but also consistent, further bolstering the tool’s credibility.
AlphaMissense in action: a catalogue of predictions
AlphaMissense’s predictions have been made freely available to researchers worldwide through the AlphaMissense catalogue. The catalogue, which assesses all 71 million single-letter mutations that could affect human proteins, is a valuable resource for geneticists, clinicians, and researchers. It provides data on which mutations are likely benign, likely pathogenic, and which need further investigation.
While not intended for direct clinical use, the catalogue can potentially guide doctors to better treatments and aid in diagnosing patients with rare disorders. It could also help identify previously unlinked mutations, shedding new light on the genetic basis of disease.
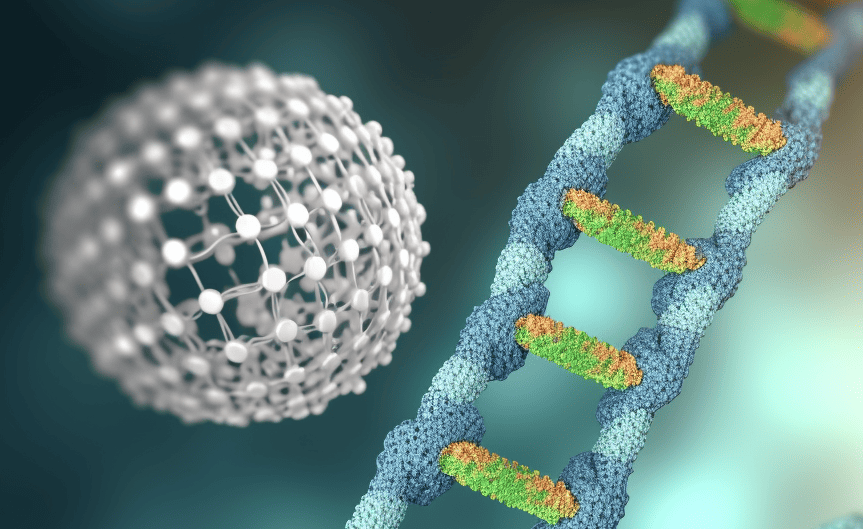
The potential and limitations of AlphaMissense
AlphaMissense represents a significant advance in our ability to predict the effects of genetic mutations. However, it’s worth noting that the model’s complexity and the need for further verification have raised some concerns among experts. The tool can identify broken genes, but understanding the underlying problem and how to fix it remains a challenge for AI.
Moreover, while AlphaMissense outperforms other computational tools in discerning disease-causing variants, it is not considered a significant leap forward like AlphaFold. Computational predictions currently have a minimal role in diagnosing genetic diseases, but as models like AlphaMissense improve, they may gain more acceptance. Rigorous evaluation of these tools, using performance metrics and benchmarking against experimental data, is crucial before their real-world application.