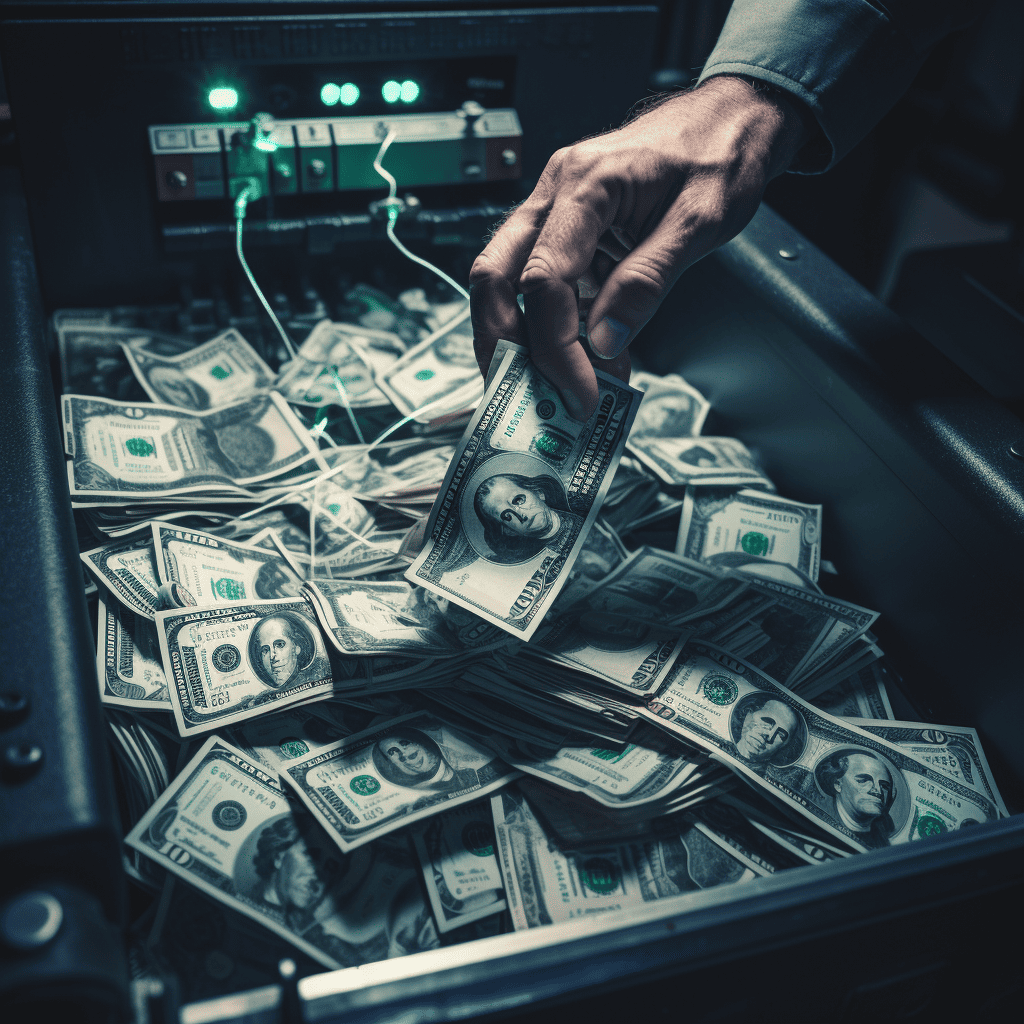
Computer scientists at King’s College London have developed a new tool that enables quicker and more accurate detection of money laundering, which can scan 50 million transactions in less than a second.
- A new software detects money laundering three times faster than conventional methods.
- The algorithms identify when criminals divide money into multiple small transactions.
- The software was tested with real data from a bank and could recognize suspicious patterns.
The researchers have designed an entirely novel approach to detecting money laundering, based on algorithms that rapidly identify when criminals divide a large sum of money into multiple smaller transactions between many bank accounts – a technique known as ‘smurfing’.
Connecting the dots
The algorithms run on data taken from several bank accounts, which are represented as nodes on a large, complex graph, and the software is programmed to focus on the part of the graph where it detects the most suspicious activity.
For example, if there is a deposit of one million pounds, the software can monitor where this exact sum of money is being transferred – it has the ability to identify all combinations of related transactions that take place, even if the money is split between different accounts and outgoings.
As detailed in a paper published in the Proceedings of the 2023 SIAM International Conference on Data Mining, lead researchers Dr. Huiping Chen and Dr. Grigorios Loukides, together with Dr. Robert Gwadera and Dr. Solon Pissis, say the new software is over three times more effective than current detection methods and can also analyse larger amounts of data.
The United Nations Office on Drugs and Crime (UNODC) estimates that between two percent and five percent of global GDP is laundered yearly, roughly between £632 billion and over £1.5 trillion.
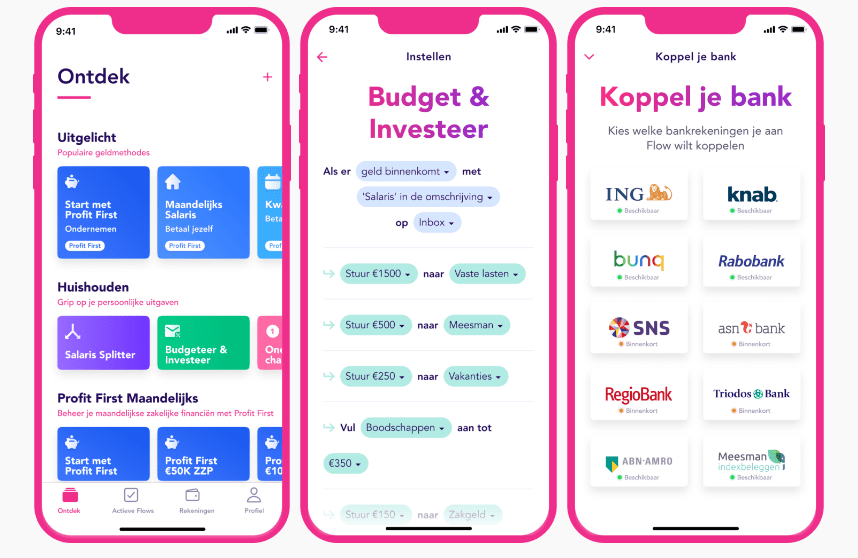
Open source software
Dr Loukides comments: “We have developed an optimal method that can find the best possible solution for detecting common classifications of smurfing attacks across millions of amounts of data, on average 3.2 times more effectively than the state-of-the-art methods currently used. We are now working to improve the tool further, to deliver a quicker speed than conventional approaches but with even higher accuracy.”
The software for the new tool is open source and freely available to access. Because it can be used with much larger amounts of data than conventional detection methods, researchers say it can analyse large amounts of data over long periods of time, filtering out and sounding an alarm to indicate to the bank when it detects suspicious activity.
Beyond financial activity
The approach has been tested using real data from an anonymous Czech bank and on fictional cases based on predictions of common patterns and activity present in cases of real money laundering. In both instances, the algorithms could detect all suspicious transaction patterns.
Researchers say the new tool also has potential use beyond the detection of suspicious financial activity, as it could optimize marketing campaigns by enabling retailers to find and detect the most profitable bundles of products, improving the accuracy of retail data at speed.