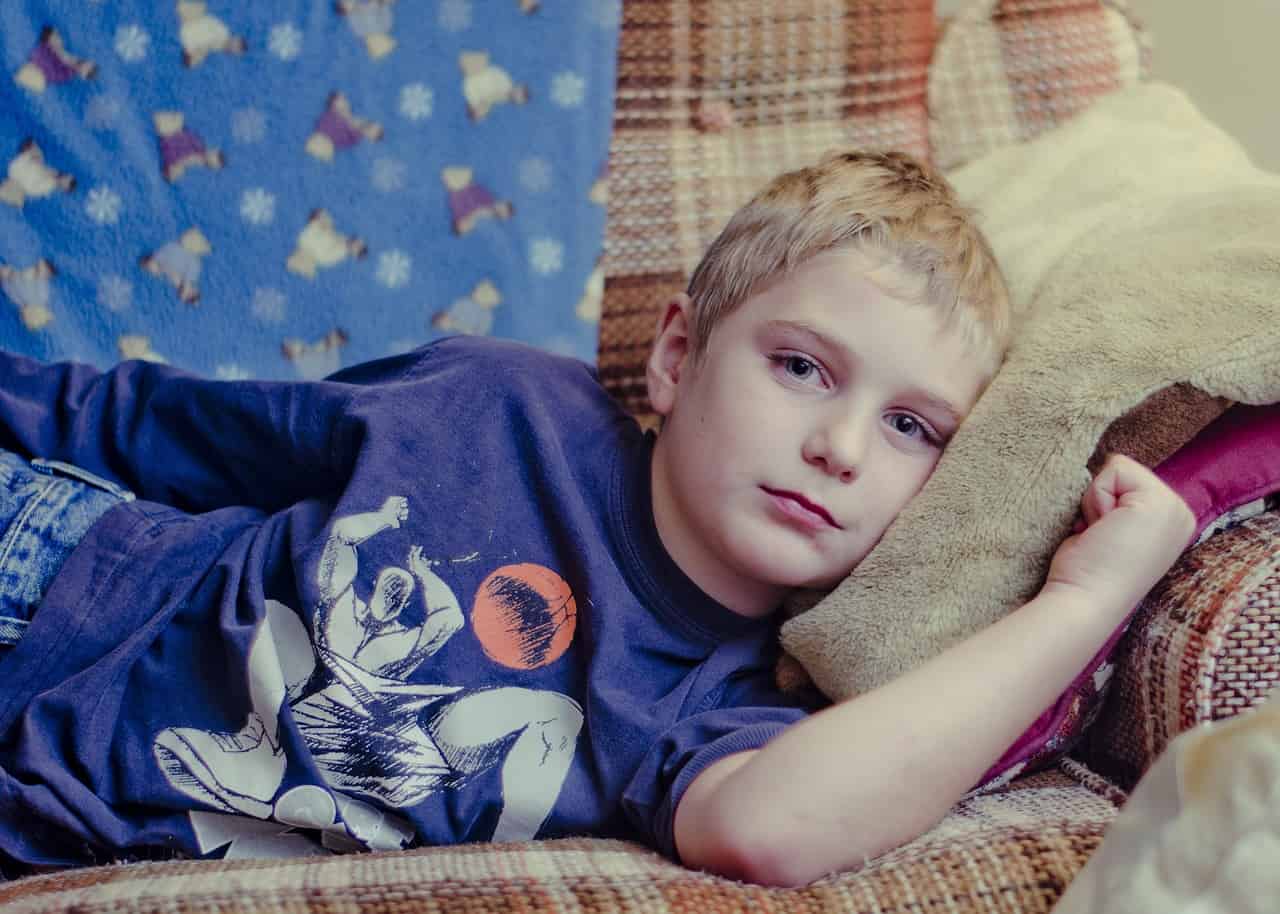
An international team, led by researchers at Imperial College London, has developed and validated a diagnostic approach capable of simultaneously detecting and distinguishing between 18 infectious or inflammatory diseases – including group B Streptococcus (GBS), respiratory syncytial virus (RSV), and tuberculosis – with the potential to provide a result in a fraction of the time of current diagnostic tests, says the university in a press release.
- A breakthrough blood test developed by an international team, can rapidly diagnose childhood illnesses by analyzing gene patterns.
- The test distinguishes between 18 diseases, including GBS, RSV, and tuberculosis, using a single blood sample, potentially providing results within an hour.
- While still in development, the test could drastically improve diagnoses, reduce delays, and impact healthcare, especially in developing regions.
Using a single sample of blood, the test could enable clinicians to diagnose the cause of fever based on the distinctive pattern of genes being ‘switched on or off’ by the body in response to specific illnesses. While current tests for some of the conditions can take several hours, days or even weeks, a test-based on this approach would be capable of providing a result in under 60 minutes.
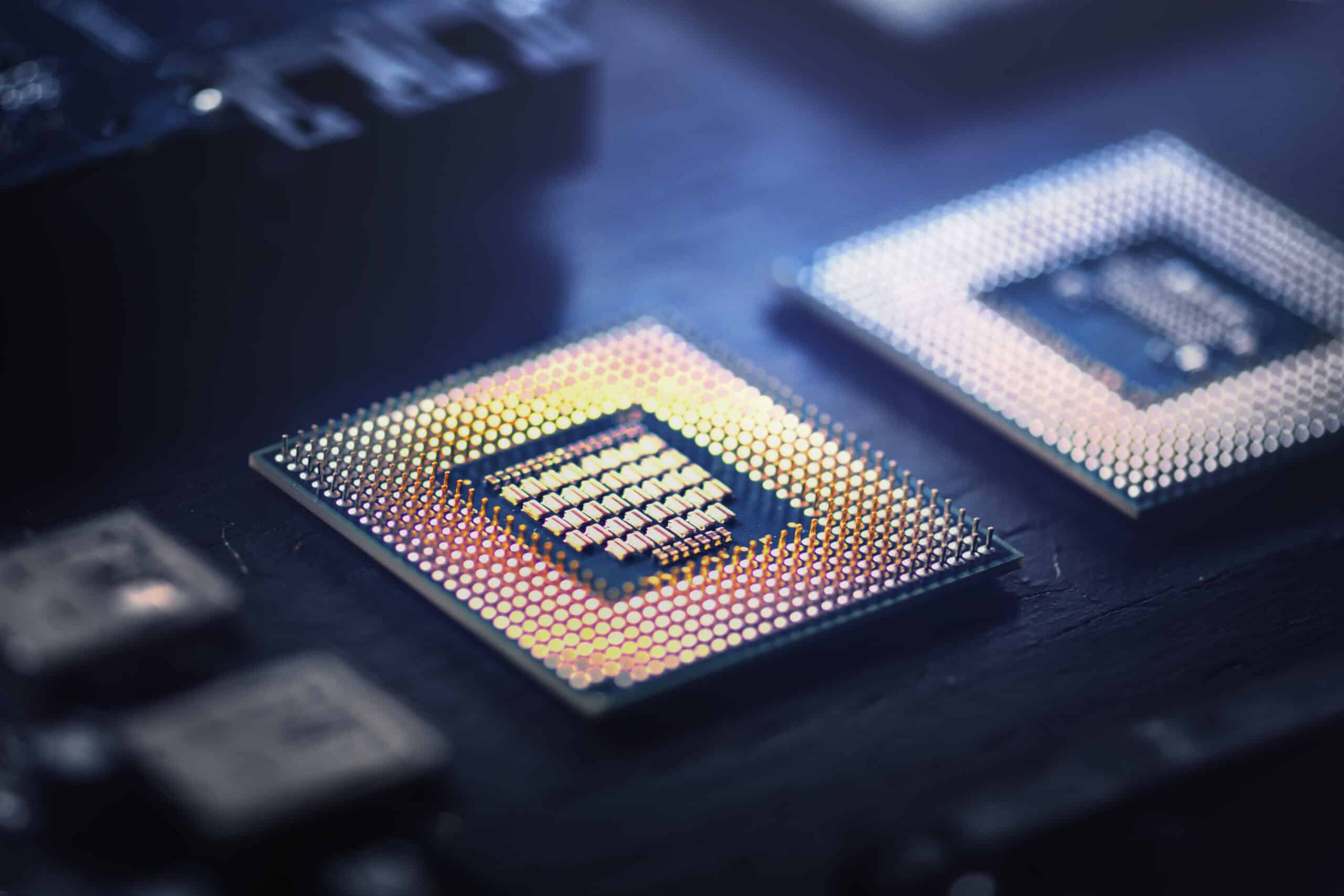
Diagnosing childhood diseases
Professor Michael Levin, Chair in Paediatrics & International Child Health within the Department of Infectious Disease at Imperial College London, and co-senior author of the paper, explains: “Despite huge strides forward in medical technology, when a child is brought into hospital with a fever, our initial approach is to treat based on the doctors’ ‘impression’ of the likely causes of the child’s illness.
“As clinicians, we need to make rapid decisions on treatment, often just based on the child’s symptoms, information from the parents, and our medical training and experience,” he adds, “but we may not know whether a fever is bacterial, viral, or something else until hours or days after a child has been admitted, when their test results come back. Such delays can stop patients getting the right treatment early on, so there is a clear and urgent need to improve diagnostics. Using this new approach, once it’s translated to near point of care devices, could be transformative for healthcare.”
In the latest study, researchers explored an approach focused on detecting the pattern of a patient’s gene expression in blood that occurs in response to specific infections and inflammatory conditions. Using data from thousands of patients (including more than 1000 children with 18 infectious or inflammatory diseases) the team was first able to identify which key genes were switched ‘on’ or ‘off’ in response to a range of illnesses – providing a molecular signature of disease.
Machine learning
Machine learning was then applied to identify which patterns of gene expression corresponded to the specific disease areas and pathogens – focusing in on a panel of 161 genes for 18 conditions. This panel was further validated in a cohort of 411 paediatric patients admitted to hospital with sepsis or severe infections (representing 13 of the 18 diseases), where gene expression was captured from blood analysis, and where diagnoses were made using current gold standard clinical methods.
New diagnostic tests cannot be tested in a clinical setting until they are approved – as some misdiagnoses could have severe consequences, such as failing to identify a life-threatening bacterial infection. Instead, the team used a ‘cost sensitive’ measure, based on consensus from a panel of five clinical experts, to show where the test could be used to avoid misdiagnosis, and where this would have the greatest consequence.
Dr Myrsini Kaforou, Senior Lecturer within Imperial’s Department of Infectious Disease and co-senior author of the paper, said: “This body of work has enabled us to identify the molecular signature of a wide range of diseases based on 161 genes, out of thousands of genes in the human genome. By distinguishing between many diseases at the same time within the same test, we have developed a more comprehensive and accurate model that aligns with the way clinicians think about diagnosis.
“With this initial proof-of-concept study, we’ve been able to show that our multi-disease machine-learning diagnostic approach works. This kind of advance is only possible through interdisciplinary collaboration and large research consortia, which bring together expertise from infectious disease, molecular science, and bioinformatics.
“There is still much work to be done to progress this test to the clinic, but we are working towards it. A future diagnostic test based on this approach could help provide the right treatment, to the right patient, at the right time, while optimising antibiotic use, and reducing lengthy time to diagnosis for inflammatory diseases.”
Next steps
The researchers stress that a functional test is not yet available for clinical practice and their RNA transcript panel would require further adaptation, testing and translation into a readily usable platform/device before it could be approved by regulators.
As part of the international, multi-partner DIAMONDS study, the next step is to trial the approach in thousands of patients in hospitals in Europe, Africa and Asia.