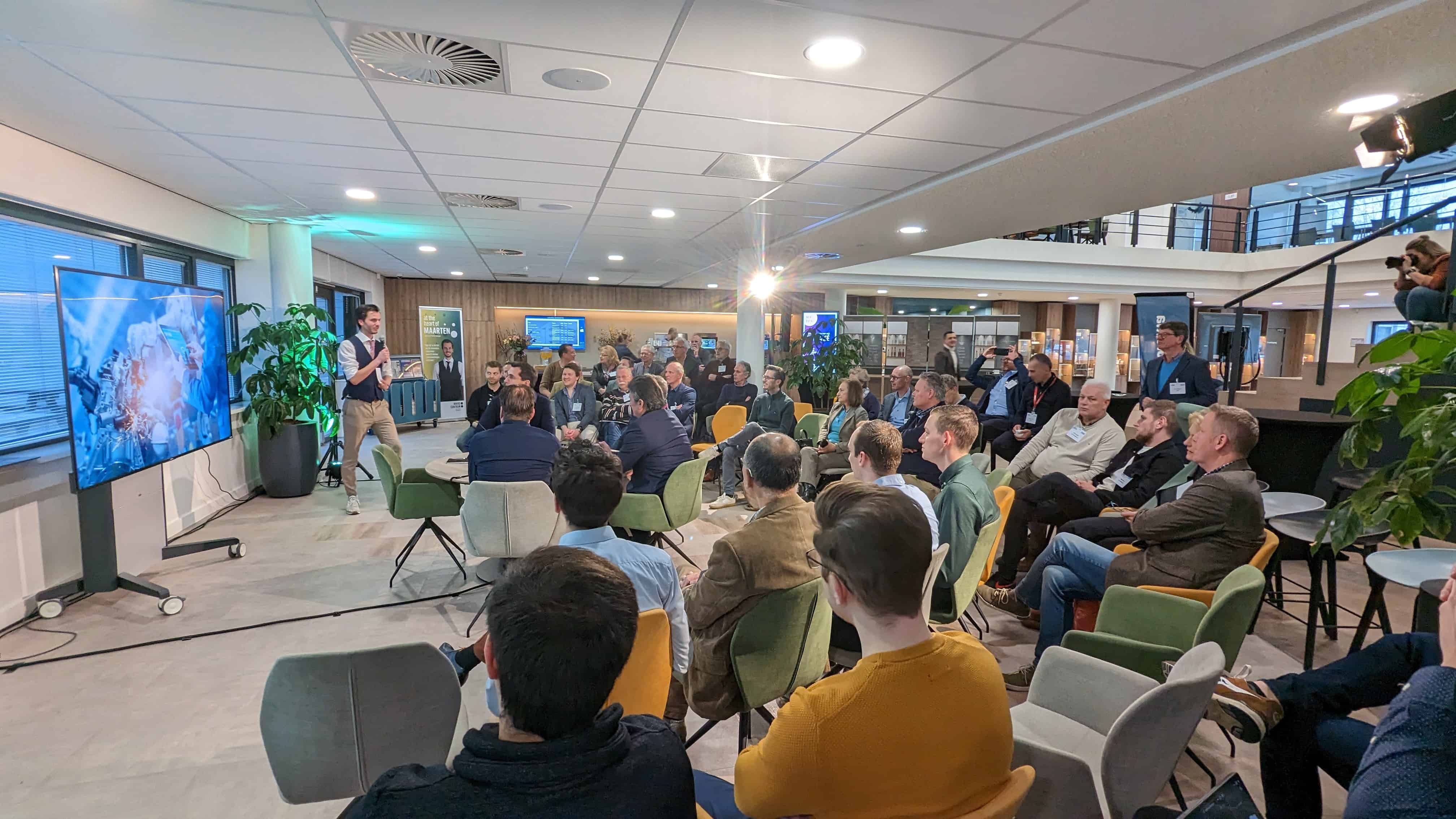
For many companies, it is already an absolute must-have: a strategy that allows you to predict the right maintenance timing of your machines and equipment. Instead of waiting for things to go wrong, use sensors, data analytics, and AI to determine when maintenance is needed accurately. It’s the way to keep your downtime low and optimize relationships with customers and suppliers.
This way of working is known by the term predictive maintenance. Because it is crucial for the high-tech manufacturing industry, Mikrocentrum and DSPE devoted a separate episode of their Techcafé sessions to it. Experts Marc Vissers (marketing and communications manager at Lenze), Willem Hofman (CEO of IXON), Arjen Ros (manager and consultant at Copernicos), and Mark Stappers (lecturer and researcher at Fontys Hogeschool Engineering) joined Maarten Roos at the table. Their conclusion: the Netherlands compares favorably with the rest of the world, but we are still in an intermediate phase. “We are still exploring when it comes to the deployment of AI, sensors, and data analytics.”
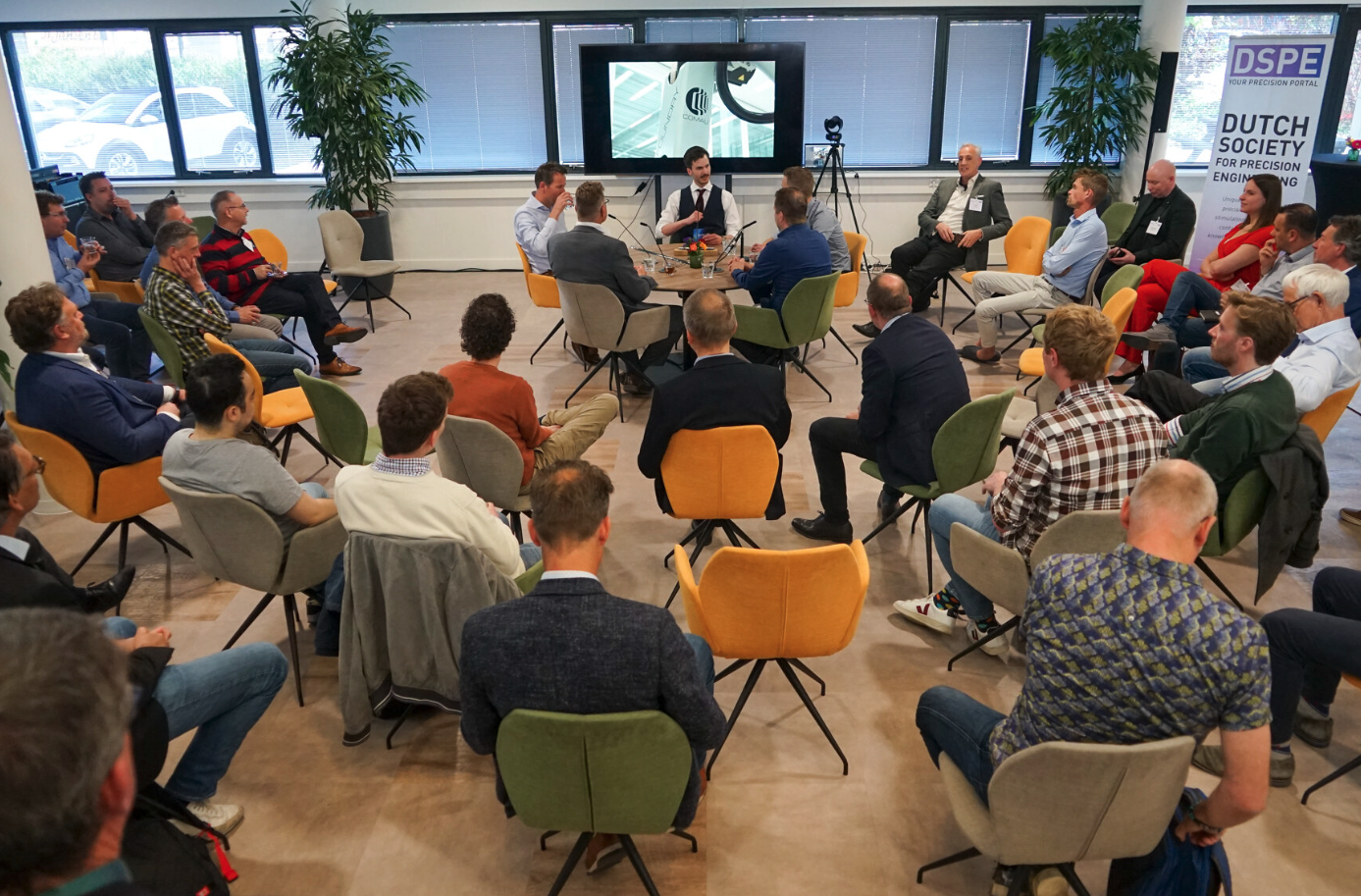
What is predictive maintenance all about? Marc Vissers answers instantly: “Making sure that when you design the machine, you already know what can happen during its lifetime. And setting up the processes and systems accordingly.” The goal, Vissers says, is to turn data into added value in terms of sustainability and of the business itself. “You want to prevent downtime,” Willem Hofman adds. “In doing so, it is important to connect the machine builder and the user.”
Scenarios from simulations
Arjen Ros mentions another important aspect of predictive maintenance: “You often hear that it doesn’t work without gigantic volumes of data. But by building models that can use simulations to create scenarios of a future situation, you also come a long way. Of course, with data, it all gets even better. But don’t wait for that; you can start early as an entrepreneur.” This is all the more true because much valuable data is already unconsciously present. “Look at your energy consumption, for example. If that suddenly increases inexplicably, that’s a signal to do some extra research.”
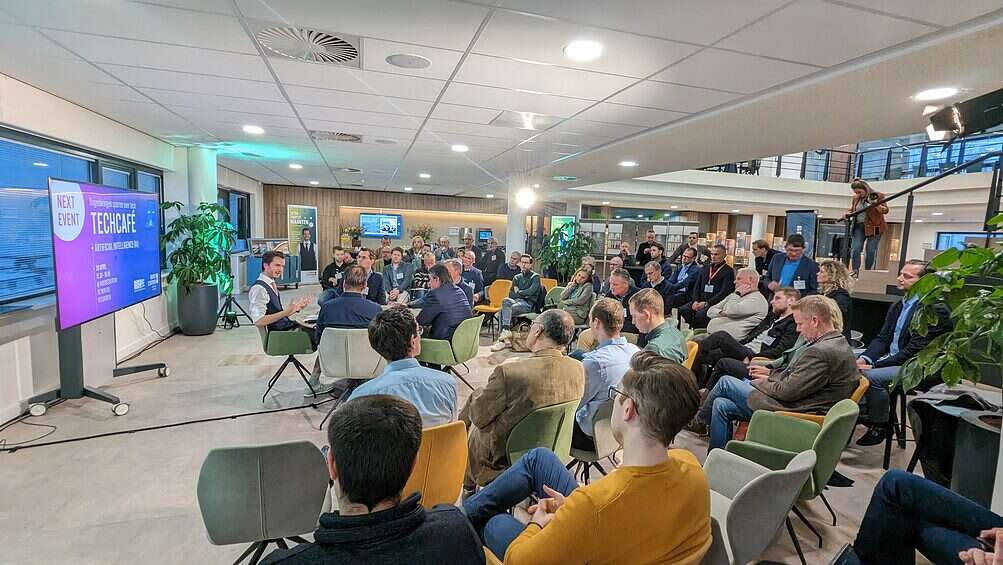
Mark Stappers also sees a lot of benefits in digital twinning. “In the applied research we set up with Fontys engineering and the industry, we make a lot of use of that.” Predictive Maintenance “is up in the air,” says Stappers. “SMEs are searching for solutions, just like the big machine builders. They are aware of the importance but are still looking for ways to do it in a business-wise manner. The same goes for education, by the way. For now, we still see it as part of our digital twinning activities, but at some point, this will also become a separate module. Until then, we will work with SMEs to find the right approach.”
Hofman also sees increasing recognition in the business community. “But at the same time, there is doubt about the how. We are still in that in-between phase: it is not yet quickly applicable, other priorities sometimes get in the way, and the real urgency is still missing. But I’m sure that won’t last long.”
New or refurbished
Vissers sees a difference between the application for new machines and refurbished models: “For the new, I see it already going quite well, but for refurbished, the brand and product orientation is stronger than the more conceptual questions. That’s not strange at all because with new, you can really start building it from the design phase.”
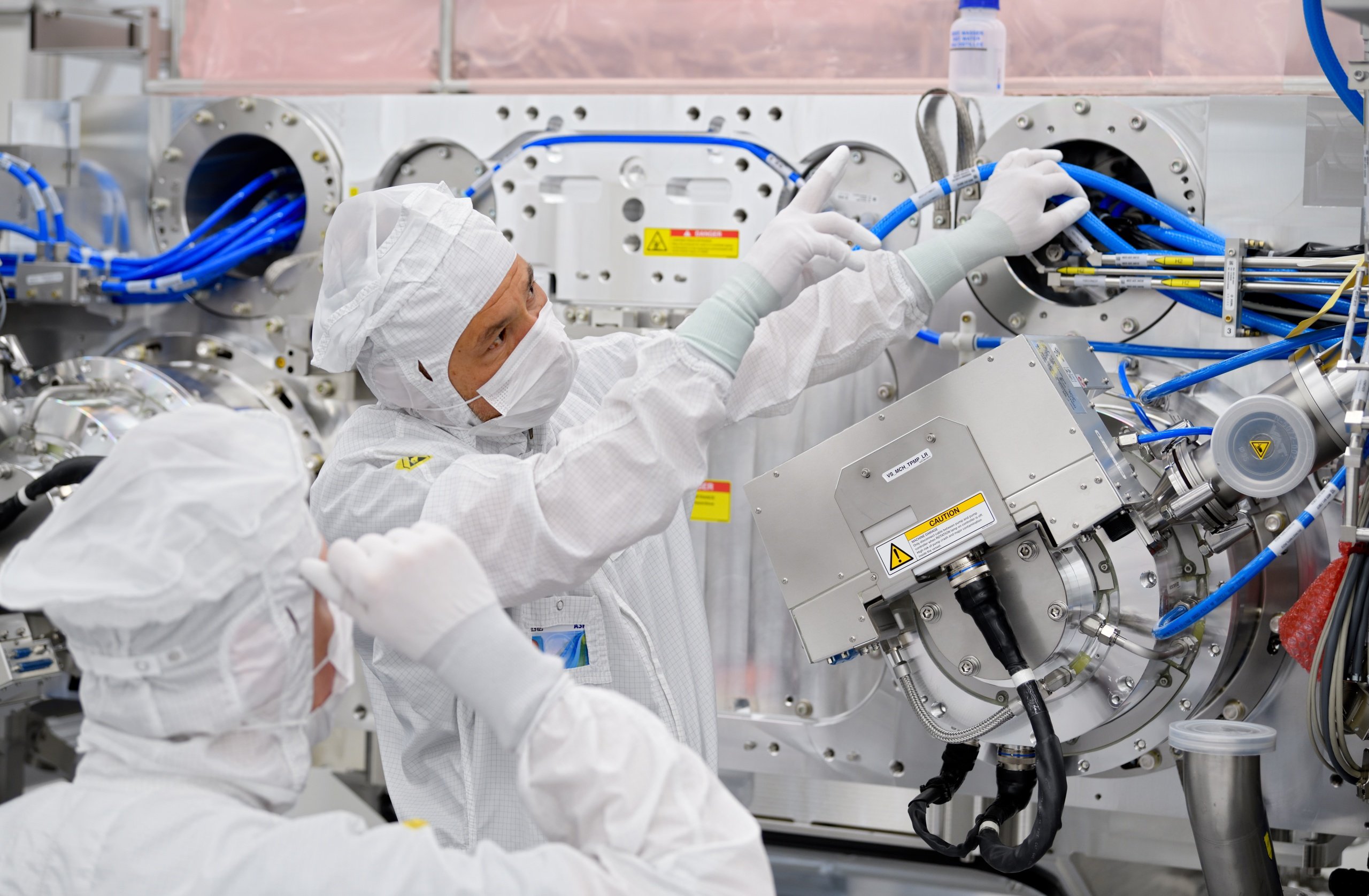
And then there is the problem that many end users have a fleet of machines that come from sometimes dozens of different suppliers. Even if all those machines are prepared for predictive maintenance, those systems have to be able to be linked together. Open Source solutions could be the answer that would greatly help end users, but are all machine builders ready for that? “In Europe, and especially in the Netherlands, maybe so,” says someone from the audience. “But outside of that, not yet. In Japan, for example, this is unthinkable.” Vissers acknowledges that, at this stage, it’s still a challenge. “But maybe a machine builder could use it as a selling point.”
Pitfalls
Pitfalls around predictive maintenance are also plentiful, the speakers collectively observe. “Not all data is equally useful,” says Stappers. “Sometimes you want to get information out of your machine that isn’t there, but often you also see that companies just start collecting and storing everything, while they can actually do very little with it.” And look not only at the machine itself but also at the environment, Ros adds. “A solar system in the desert operates differently than a naval vessel on the ocean.” Accessibility also plays a role: if people walk around and use the machines daily, that’s an essential source of information. “But that doesn’t apply to those solar systems in the desert,” Hofman says. “Fortunately, we can remotely monitor whether the batteries are still working and the panels haven’t become too dirty. We then tune the maintenance accordingly.”
Ros, too, still sees barriers. “Predictive maintenance requires people who understand the impact of AI; if you don’t understand that, then you have a problem. In addition: in a world that changes so quickly, you must recognize that not everything is predictable, even with the best AI. And perhaps most importantly: AI is not a replacement for humans. So whoever knows how to best combine the knowledge in people’s heads with artificial intelligence is on the right track. Always listen to your professionals!”
“It’s really going to be very nice!”
Willem Hofman
The Netherlands may be on the right track, but there is also a lot of room for improvement, it is noted. “But where will we be in, say, five years,” Maarten Roos wants to know. Roos sees it mainly in interconnectedness, moving from individual components to complete systems. Stappers hopes for a separate course with links to applied mathematics, ICT, and other studies. For Vissers, that’s when the machine builders are all involved, and thanks to the input of a wide range of new specialties, they can serve their end users even better. Hofman gets a broad smile as he tries to imagine the world five years from now. “Everyone will be working on it by then because we all see the value of predictive maintenance. It’s really going to be very nice!”