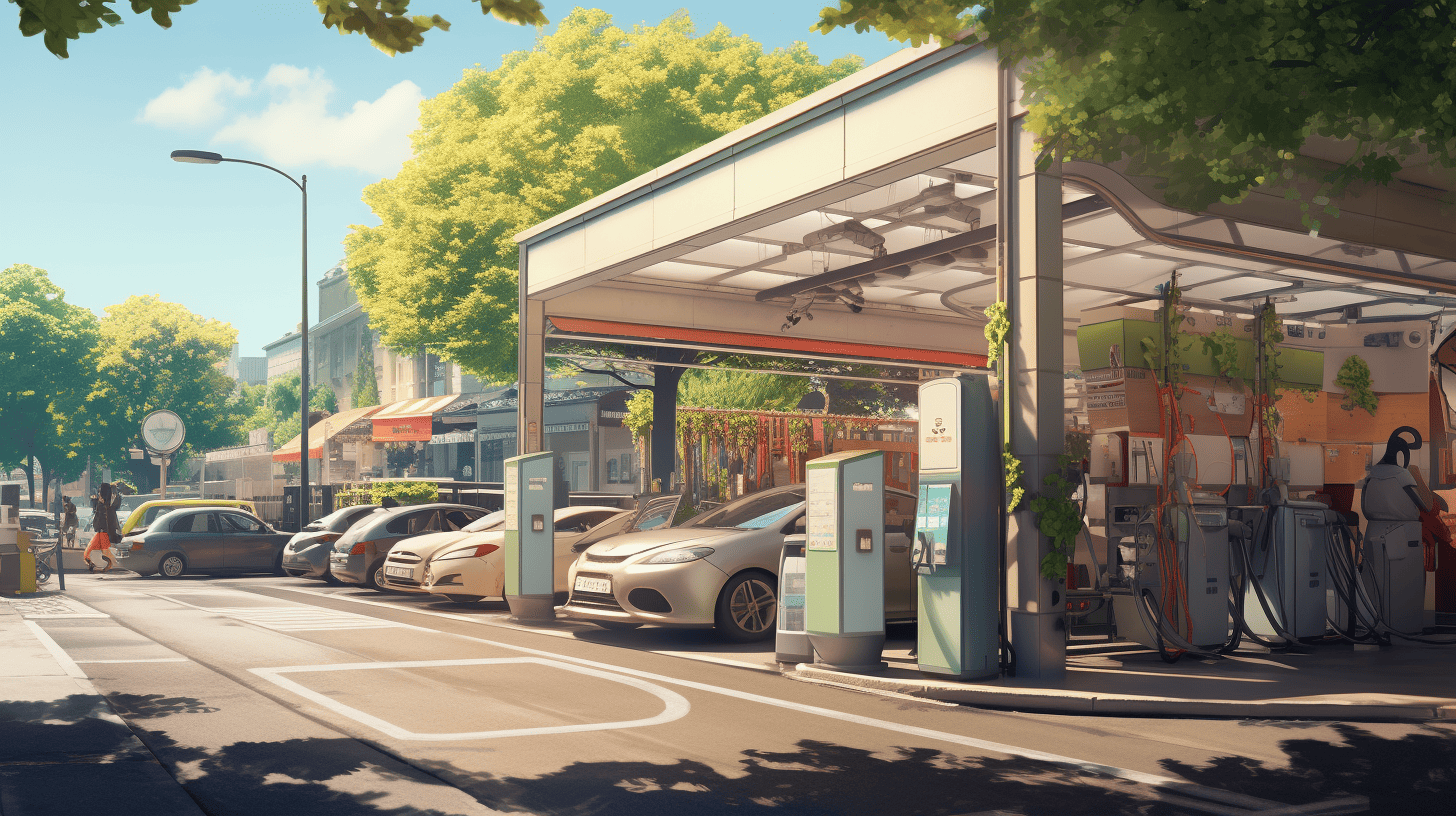
The increasing electrification in society leads to higher peak loads on the electricity grid. Some people are warning for an energy transition that is too fast. Others are hopeful for a fully electric future. In a report commissioned by the Dutch Topsector Energie program, more than 100 potential solutions are offered, all focusing on maximizing the capacity of the existing or upcoming electricity network to accelerate the energy transition. In a 12-part series, Innovation Origins spotlights the twelve most promising solutions. This is part 11: Swarm optimization in electric charging.
- The Dutch Topsector Energy collected 100 ideas to solve the issues around grid congestion.
- One of the twelve most powerful ideas is swarm optimization in electric charging.
- Swarm optimization of charging contributes to the more efficient use of available capacity, provided it doesn’t lead to a worse experience for individual users.
The electricity network can be used more efficiently by smartly charging and discharging electric vehicles (EVs) using algorithms, AI, and information about network congestion. The technology should optimize the collective interest without harming individual interests too much. A specific time and place might seem optimal for an individual EV to charge based on its destination or route but if that charging location is in a congestion area with limited capacity, collective optimization is better, as seen in projects like Flexpower. Collective optimization also helps individuals avoid reduced charging power and longer charging times, preventing local network overloading.
Inspiration can be drawn from route and charging planners for electric cars. For instance, to offer users optimal charging recommendations, Tesla uses AI with real-time data, including charger locations, current battery levels, charging speeds, and distance to the destination. Several apps exist to provide EV drivers with optimal convenience in planning charging and travel times. Similar software can be developed, taking into account available network capacity. The most optimal charging locations for the collective EV drivers can be found by smartly setting optimization parameters and algorithms.
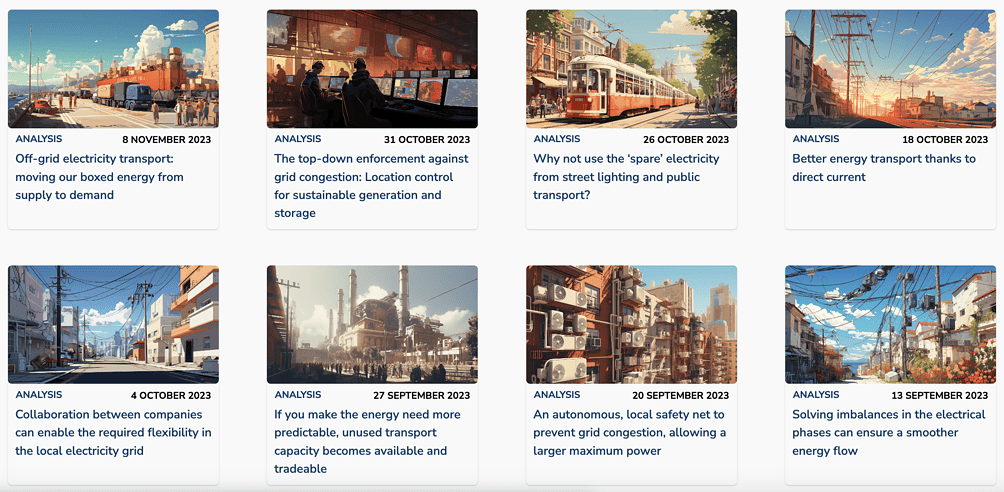
More efficient
Swarm optimization of charging contributes to the more efficient use of available capacity, provided it doesn’t lead to a worse experience for individual users. It’s the electricity variant of traffic information and dynamic route planning on the road network. The solution’s impact and applicability are greater for destination charging, where the vehicle remains stationary for several hours. For fast charging on the way to a destination, the impact on better utilization of the electricity network is limited, but choosing specific charging locations can alleviate local congestion.
Stakeholders include EV drivers and EV fleet managers who benefit from many available charging options and clarity about charging speeds. Part of the solution should involve tech companies developing apps or other digital tools to provide insight into charging capacities, prices, and the most favorable places to charge. Communication about capacity between the network manager and Charge Point Operator (CPO) is essential, as well as with the aforementioned apps or tools that communicate charging capacities to the user.
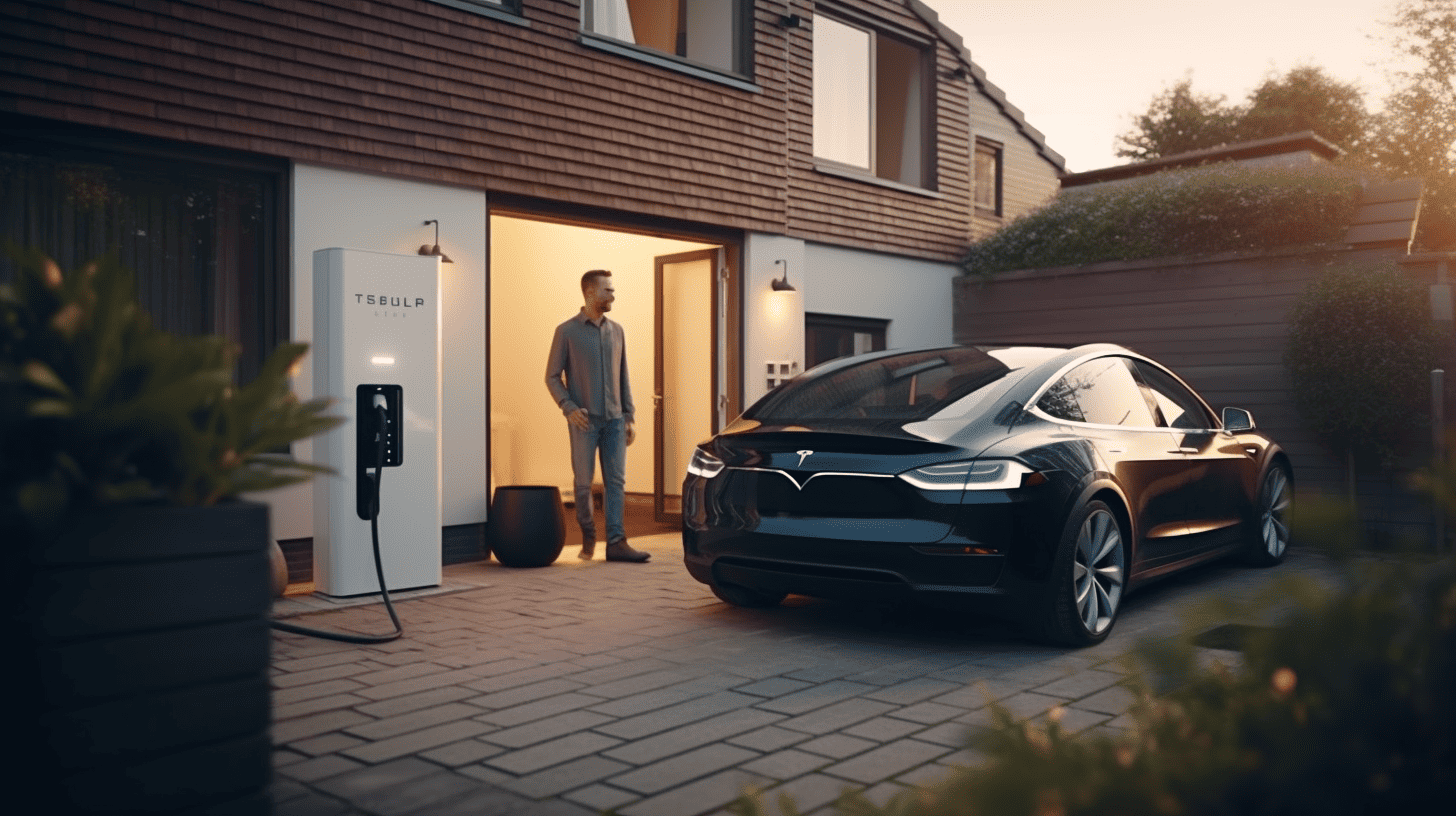
Data, apps, and platforms
Developing data exchange, apps, and platforms is crucial. Open-source development is encouraged to prevent dominance by commercial parties, which could lead to resistance, ambiguity, or perverse incentives. Care must also be taken with the use of AI and associated biases. To implement the solution, efforts and coordination from diverse stakeholders are needed. More (near-real-time) data is required from the electricity network and users. This data must be reliable and secure. Regulations, supervision, and communication protocols are necessary. Smart algorithms (with or without AI) need to be developed for large-scale EV use, processing vast amounts of data to optimally guide all electric vehicles in an area based on network load.
Pilots with electric vans in a specific municipality, for example, can help develop the technology, through development, testing and further development. This solution can be implemented for both passenger transport and in the logistics sector; for both long-distance and urban distribution. A combination with energy hubs at business parks is also obvious; transporters can make better use of collective capacity by coordinating loading times. Finally, participation of enough EV drivers is crucial for the success of this solution. A prerequisite is that the solution is easy to use and financially attractive for both individual EV drivers and transporters.