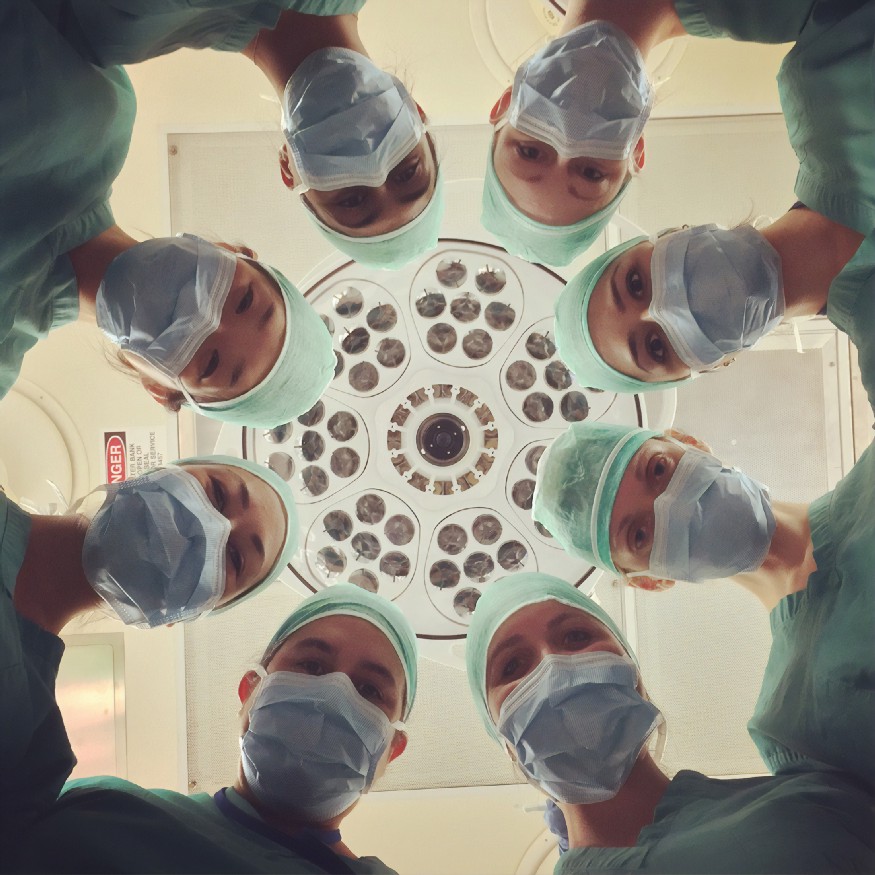
Three years ago on a warm April morning I shuffled into my first thermodynamics exam. It was notoriously hard. A whopping 64% wouldn’t get a passing grade. But I was sure I would, because I had a secret weapon. In my bag was a TI-84 Plus calculator packed to the brim with old exam questions and cryptically typed out algorithms detailing how to solve similar assignments.
All I had to do when given a question, was find the one in my calculator that most resembled it and adjust the parameters. Or so I thought.
That first time I got a 2 out of 10. Right, reality check. Turns out there’s a lot more to thermodynamics than following a series of algorithms or laws.
I failed because I hadn’t learned anything about why I was using one law for this system and another for that system. I was only looking for patterns in my training dataset (the old exams) repeated in the exam before me.
Incidentally, this kind of decision making is exactly what the field of artificial intelligence struggles with most. Connecting a body of theoretical knowledge to new, complex, real-world scenario’s. Abstracting knowledge from one field, to serve in another.
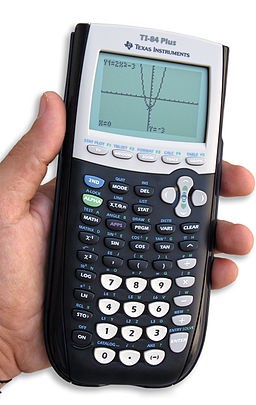
Narrow AI is beating specialists at their game
With the astronomic rise of computing power and big data, something called narrow AI has flourished. Presented with enough data of similar cases a narrow AI algorithm can learn to make decisions for other similar cases. This is what I tried to do on my thermodynamics exam.
An amazingly useful example of this at my university (TU/e) is the detection of bowel cancer on a colonoscopy video. In research lead by Fons van der Sommen they were able to live detect early-stage cancerous tissue correctly 90% of the time, where doctors diagnosed it correctly 73%.
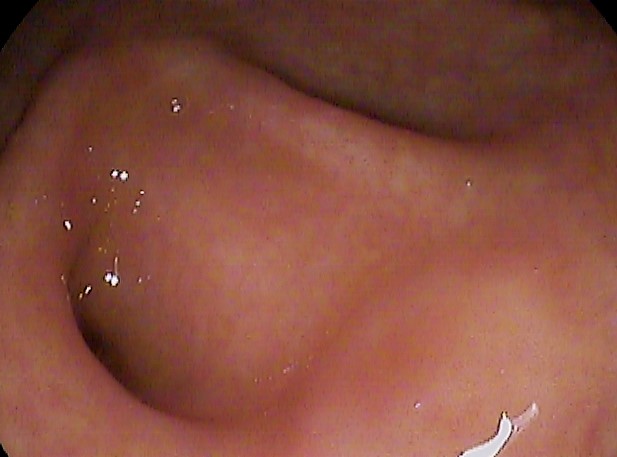
In The Netherlands where yearly 5200 people die from bowel cancer, these improved rates of early detection can result in 1331 people saved from an early death. So when I ran into Fons in the hallways of our university I had to learn more about his findings and what the next steps would be to get his algorithms to assist doctors in the field.
Then he told be something remarkable. The doctors he was working with had almost lost faith in the algorithm. Why? Because one of them had — by accident — taken a picture of the ceiling of the hospital room and the algorithm said it had, with 93% certainty, detected bowel cancer.
So what happened here? And are the doctors right to worry? First of all, doctors are always right to worry, they’re dealing with human lives. But in this case their worry was misguided. They evaluated the algorithm like them would have another doctor. Imagine the sheer stupidity of a doctor saying it has detected cancer not in your gut but on the wood-fibre panels of the hospital ceiling.
What really happened is that an algorithm that was only trained on images of bowels, some with and some without cancer, had been taken outside of its context.It might be better than doctors in detecting bowel cancer, but that’s all it can do. That’s why it’s called narrow AI. It can only do this one task.
General intelligence enables us to move knowledge from one context to another
Our human intelligence is called general intelligence. This enables us to understand abstract theories and models and apply them to new situations. It enables us face novel problems every day, without retraining.
When the answer is discrete and can be based on a wealth of data like in the case of bowel cancer, narrow AI is a great solution. When the situation requires the interpretation of a previously unknown problem, humans still triumph.
The truth is, we’re a long way from building anything like our brilliant minds. But if we want to stay relevant we do have to change the way we think about our minds and our ability to solve problems. Preferably without failing an exam in the process.
The time of specialists might come to an end, but then again, we were never really evolved for that in the first place. I say, let the computers do the boring work of finding more of what we’ve already seen and let us focus on new and interesting problems.
About this column
In a weekly column, alternately written by Buster Franken, Eveline van Zeeland, Jan Wouters, Katleen Gabriels, Mary Fiers, Tessie Hartjes, and Auke Hoekstra, Innovation Origins tries to find out what the future will look like. These columnists, occasionally supplemented with guest bloggers, are all working in their own way on solutions for the problems of our time. So tomorrow will be good. Here are all the previous articles.