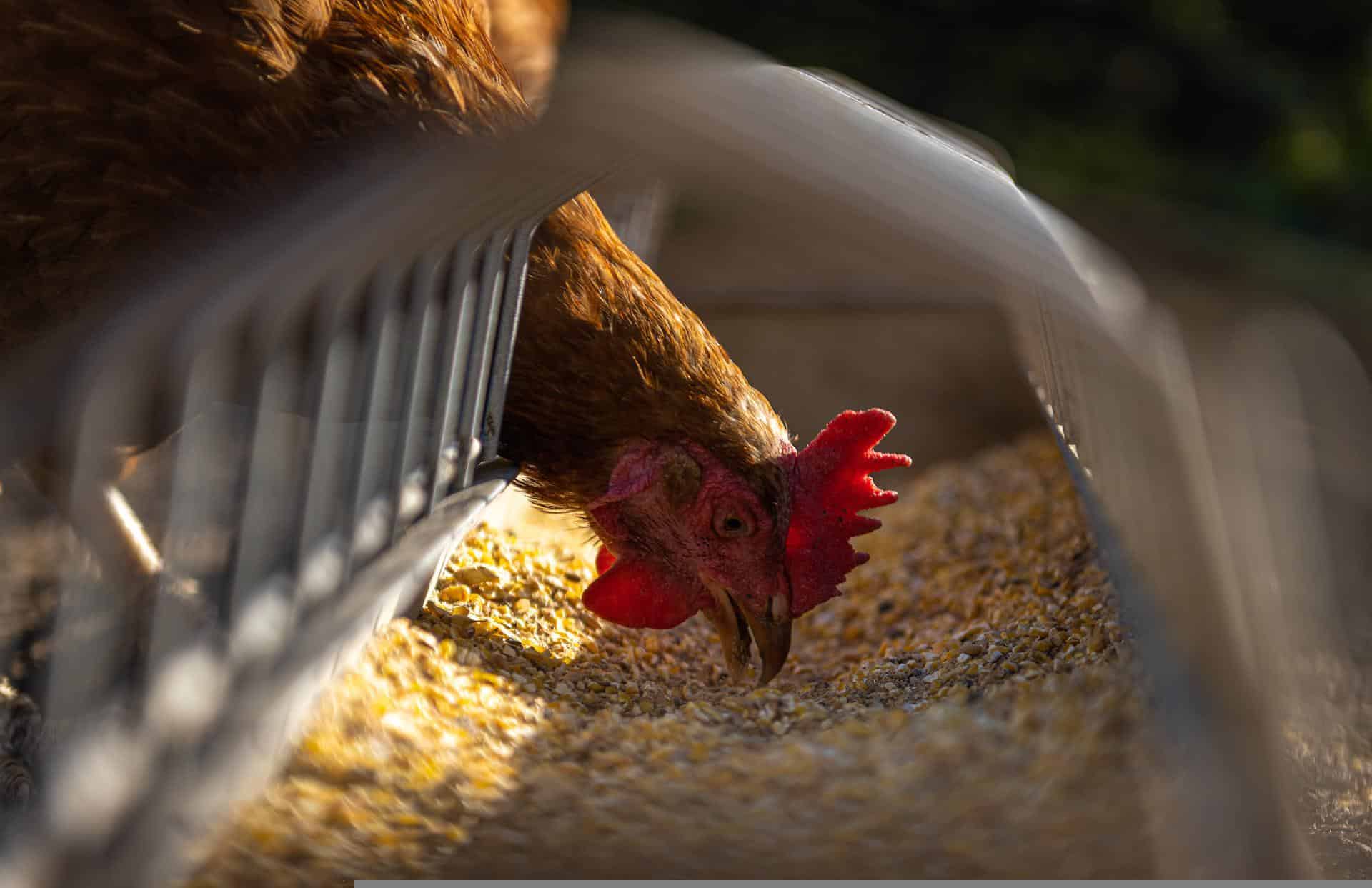
Scientists have used machine learning to find new ways to identify and pinpoint disease in poultry farms, which will help to reduce the need for antibiotic treatment, lowering the risk of antibiotic resistance transferring to human populations, says the University of Nottingham in a press release.
The study, published in Springer Nature, was led by Dr. Tania Dottorini at the University of Nottingham. The research is part of the FARMWATCH project, a £1.5m partnership between the University and the China National Center for Food Safety Risk Assessment.
Antibiotic resistance
With antibiotic resistance now one of the most threatening issues worldwide, effective and rapid diagnostics of bacterial infection in chicken farming can reduce the need for antibiotics, which will reduce the risk of epidemics and antibiotic resistance.
In this project, researchers in Nottingham collected samples from the animals, humans and environment in a Chinese farm and connected slaughterhouse. This complex ‘big’ data has now been analysed for new diagnostic that will predict and detect bacterial infection, insurgence of antimicrobial resistance (AMR), and transfer to humans. This data will then allow early intervention and treatment, reducing spread and the need for antibiotics.
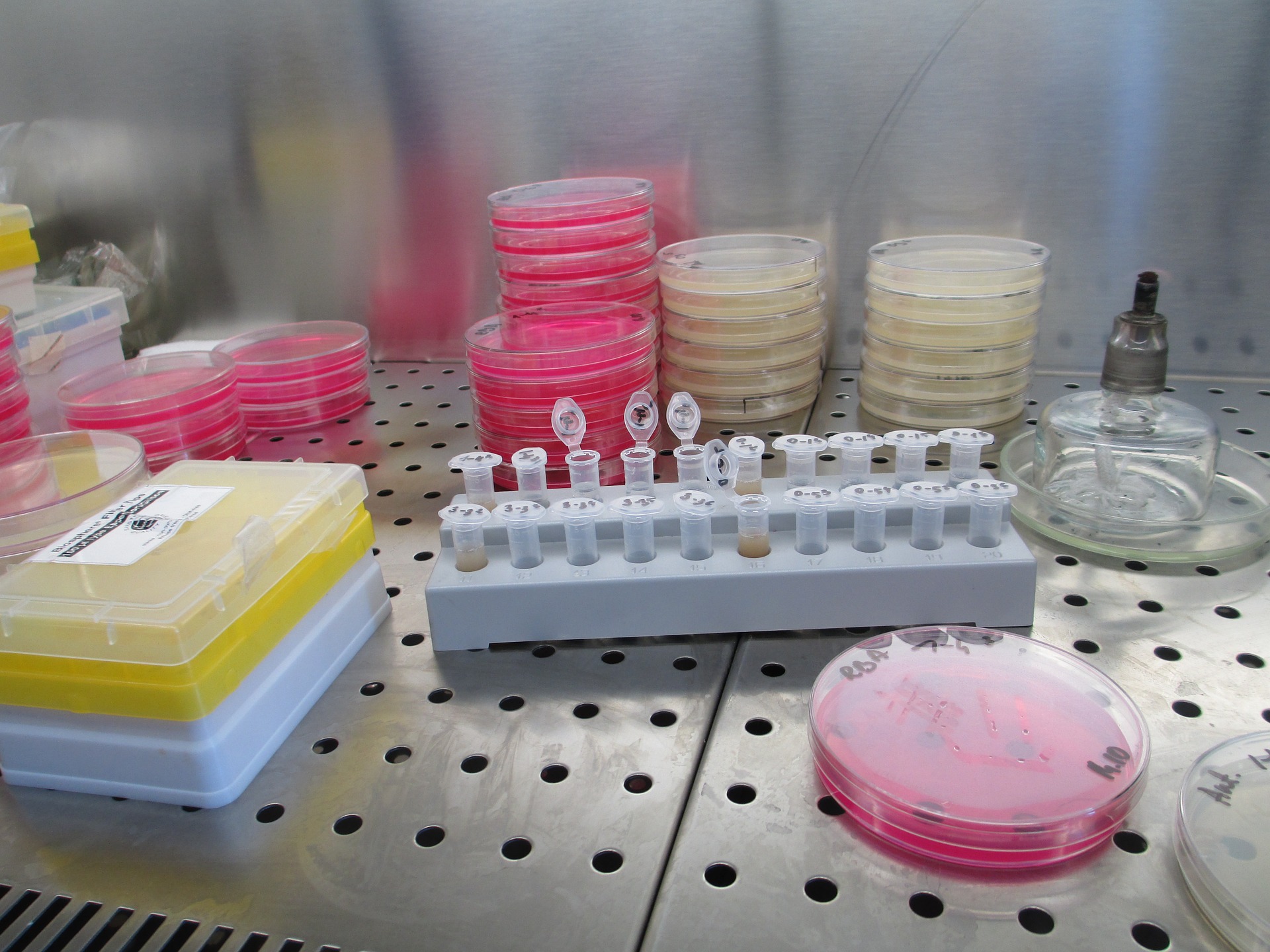
Machine learning
The study produced three key findings. Firstly, several similar clinically relevant antimicrobial resistance genes (ARGs) and associated mobile genetic elements (antibiotic resistance genes able to move within genomes and between bacteria), were found in both human and broiler chicken samples.
Secondly, by developing a machine learning-powered approach the team found the existence of a core chicken gut resistome that is correlated with the AMR circulating in the farms. Finally, using sensing technology and machine learning, the team uncovered that the AMR-related core resistome are themselves associated with various external factors such as temperature and humidity.
Dr Dottorini said: “The food production industry represents a major consumer of antibiotics, but the AMR risks within these environments are still not fully understood. It is therefore critical to set out studies and improved methods optimised to these environments where animals and humans may be in close contact. Precision farming, cost-effective DNA sequencing and the increased adoption of machine learning technologies offer the opportunity to develop methods giving a better understanding and quantification of AMR risks in farming environments.”
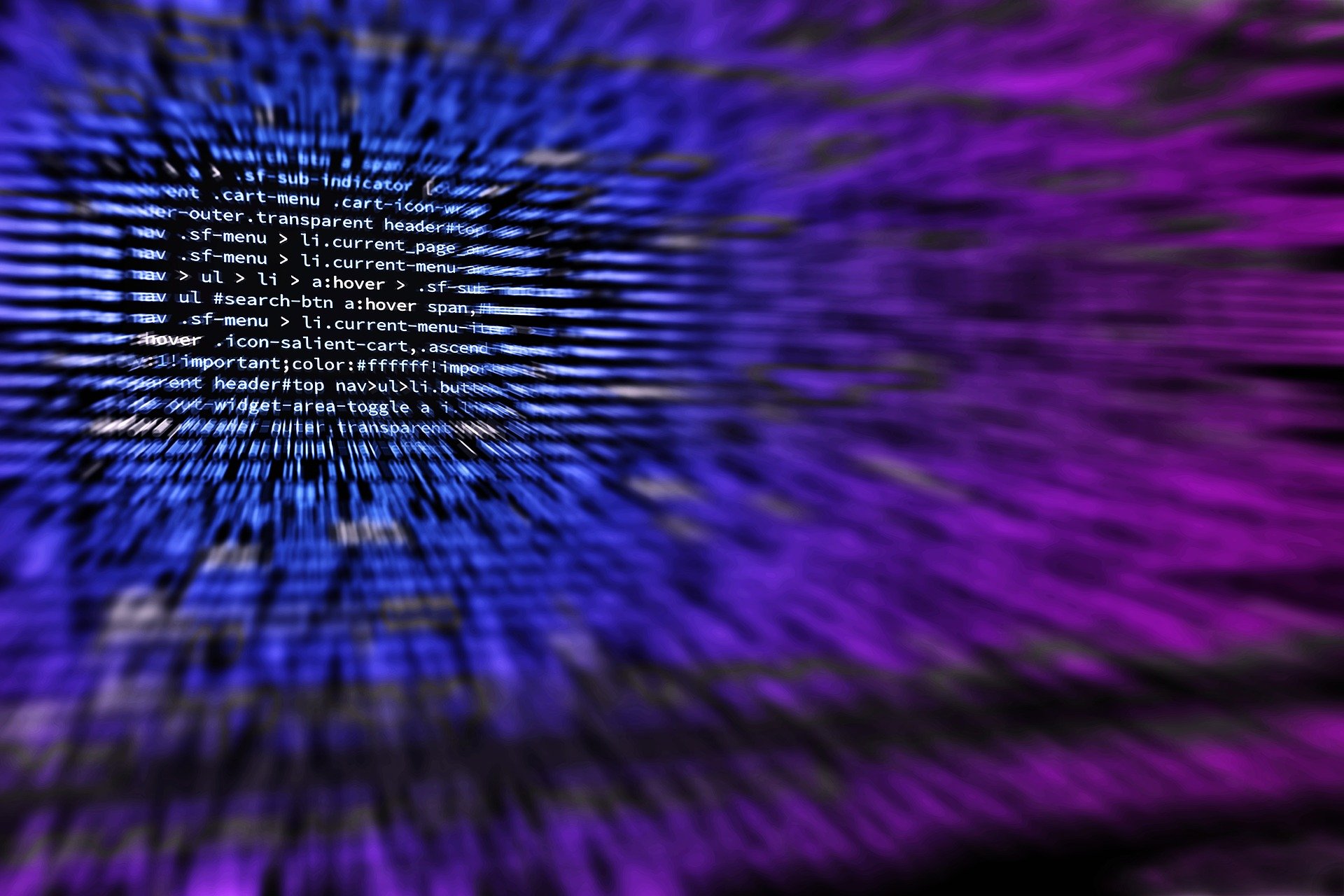
Selected for you!
Innovation Origins is the European platform for innovation news. In addition to the many reports from our own editors in 15 European countries, we select the most important press releases from reliable sources. This way you can stay up to date on what is happening in the world of innovation. Are you or do you know an organization that should not be missing from our list of selected sources? Then report to our editorial team.