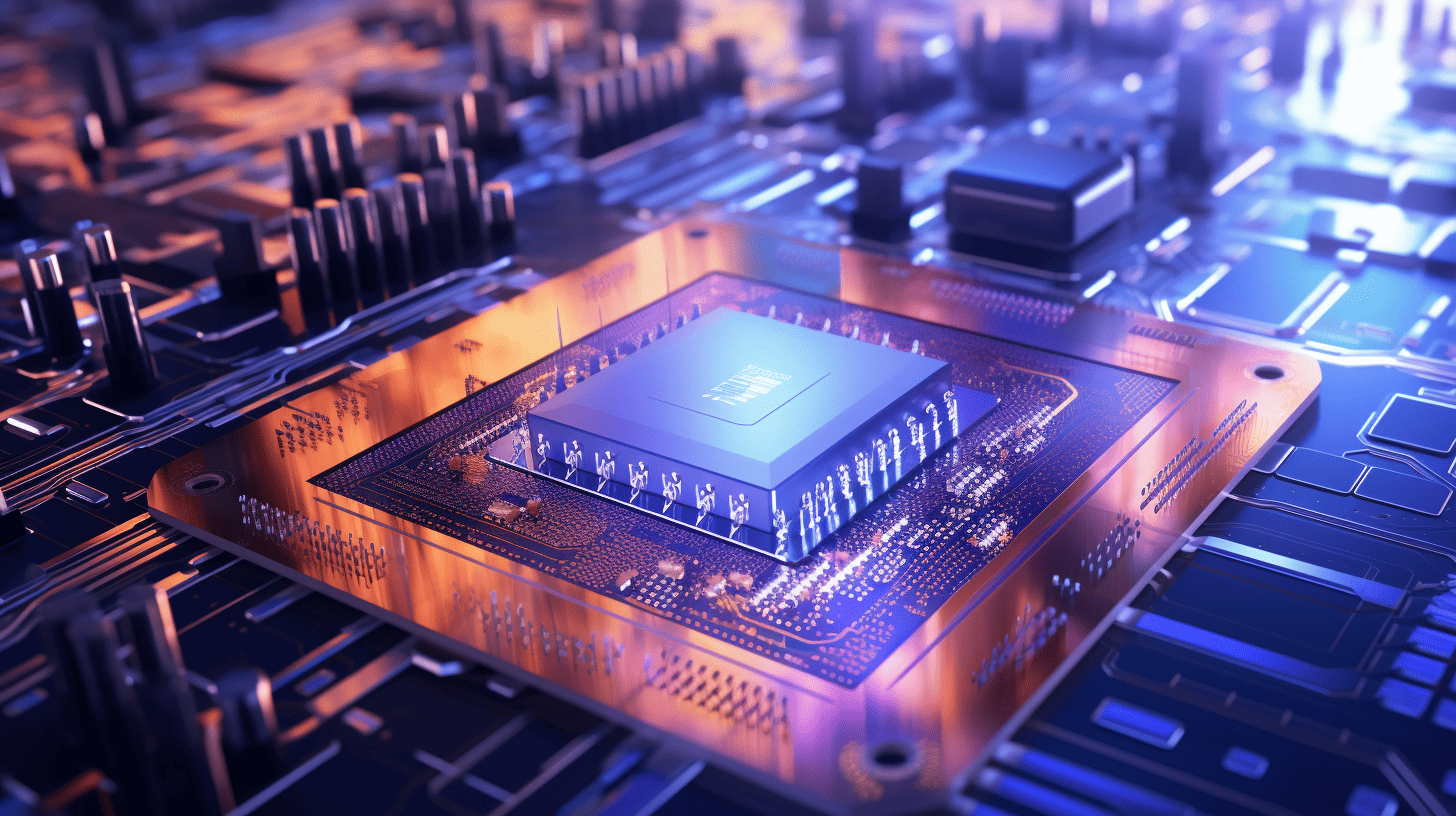
In the world of computing, speed and energy efficiency are often opposing forces. As machines learn to handle more complex tasks faster, their energy consumption increases. But what if there was a way to achieve faster computing speeds while reducing energy consumption? Enter ‘Lightning’, a photonic-electronic system developed by the Massachusetts Institute of Technology (MIT) researchers. This innovative system combines light and electrons, unlocking faster, greener computing potential.
A Leap Forward For Machine Learning
The system is designed to accelerate machine learning tasks. Lightning can speed up deep neural networks by utilizing photonic computing, which performs computations using photons instead of traditional transistors and wires. This enables real-time machine learning inference tasks such as image recognition and language generation. The successful development of Lightning marks a significant step forward in the field of photonic computing, offering a promising solution for faster and greener computing, MIT claims.
Integrated Photonics
The MIT findings touch upon the basics of ‘integrated photonics’, a semiconductor system developed by Dutch researchers and entrepreneurs in the fields of photonics and electronics. As Ewit Roos, director at PhotonDelta, said to Innovation Origins: “Light has different applications than electricity. Light will, therefore, never be able to replace electronic circuits altogether. That is why the semiconductor industry is interested in integrating photonic functions into or alongside electronics: it enriches and enables new applications.”
Photonics is similar to electronics. However, it uses photons (light) to transmit information instead of electrons. Photonic technology detects, generates, transports, and processes light. Current applications include solar cells, sensors, and fiber-optic networks. Photonic chips, called Photonic Integrated Circuits (PICs), integrate various photonic and often electronic functions into a microchip to make smaller, faster, and more energy-efficient devices. This is very much visible in, for example, data centers: by harnessing the power of light, PICs can process and transmit data more effectively than their electronic counterparts. As with traditional chips, the manufacturing process is via automated wafer-scale technology. This allows the chips to be mass-produced, thus reducing costs. Integrated photonics offers a sustainable solution to society’s energy consumption and technological challenges. The ultimate application range is broad, from energy-efficient data communication to sensors for medical applications and car battery management.
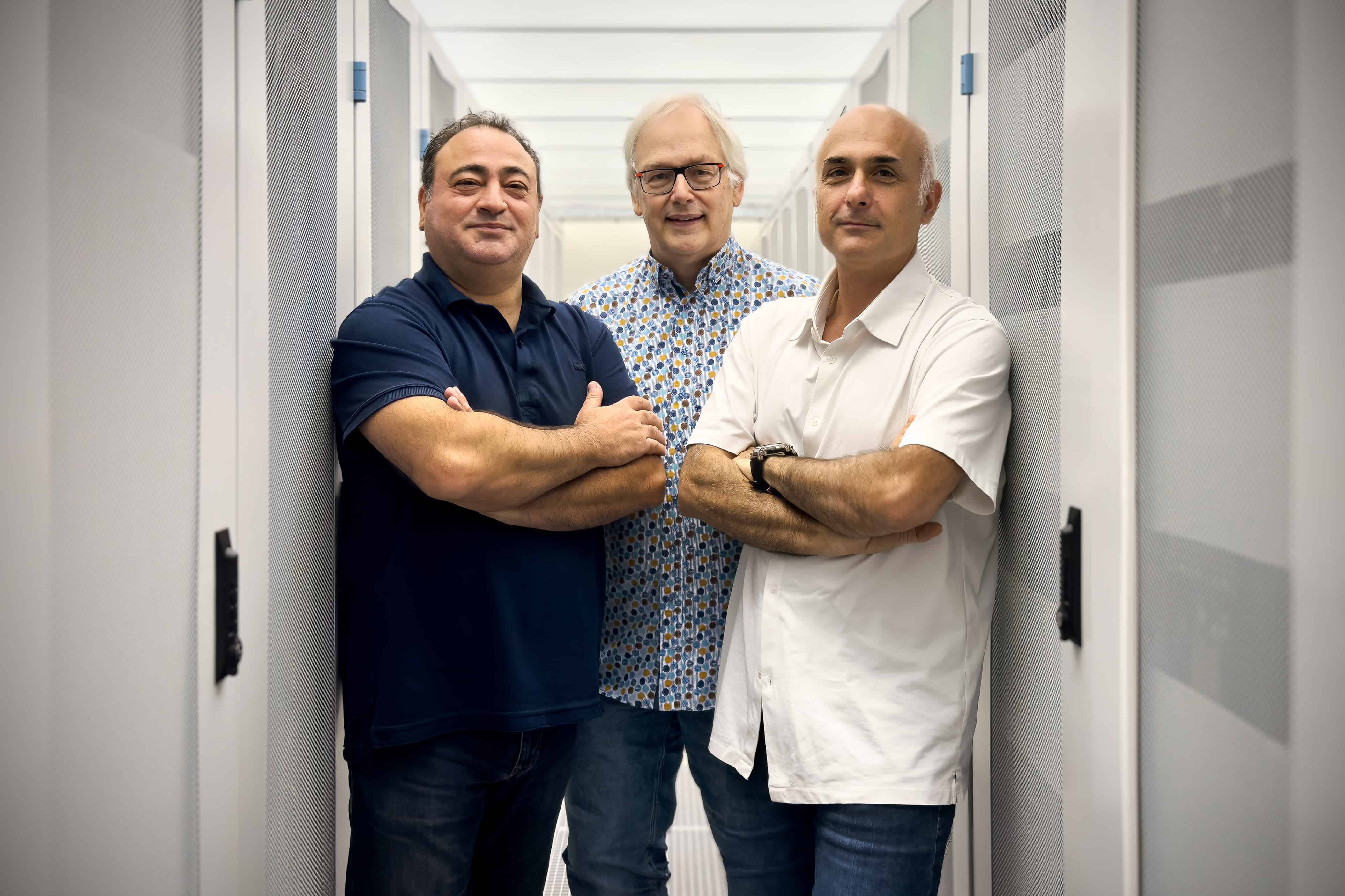
Overcoming the Memory Challenge
One of the main hurdles in implementing photonic computing devices is the lack of memory and instructions to control dataflows. The Lightning system leaps over this obstacle by seamlessly connecting photonics to electronics. The researchers developed a novel count-action programming abstraction that acts as a unified language between the two components, ensuring smooth data movement between them. This makes Lightning a computing system capable of serving real-time machine learning inference requests.
Environmental and economic benefits
Lightning doesn’t just offer computational advantages. Machine learning services, such as ChatGPT and BERT, consume substantial computing resources and emit large amounts of carbon dioxide. Lightning generates less heat and greater energy efficiency by utilizing photons, which move faster than electrons. This system reduces machine learning inference power consumption by orders of magnitude compared to traditional accelerators, making it a cost-effective and environmentally-friendly option for data centres.
Lightning vs. other systems
According to MIT, Lightning came out on top compared to standard graphics processing units, data processing units, SmartNICs, and other accelerators. The researchers found that Lightning outperformed these systems regarding energy efficiency when completing inference requests. The design shows potential as an upgrade for data centers, reducing the carbon footprint of machine learning models and improving the inference response time for users, MIT concludes.
Various organizations, including DARPA, ARPA-E, the United States Army Research Office, and the National Science Foundation, supported the research conducted by the MIT team. This month, the team will present their findings at the Association for Computing Machinery’s Special Interest Group on Data Communication (SIGCOMM).