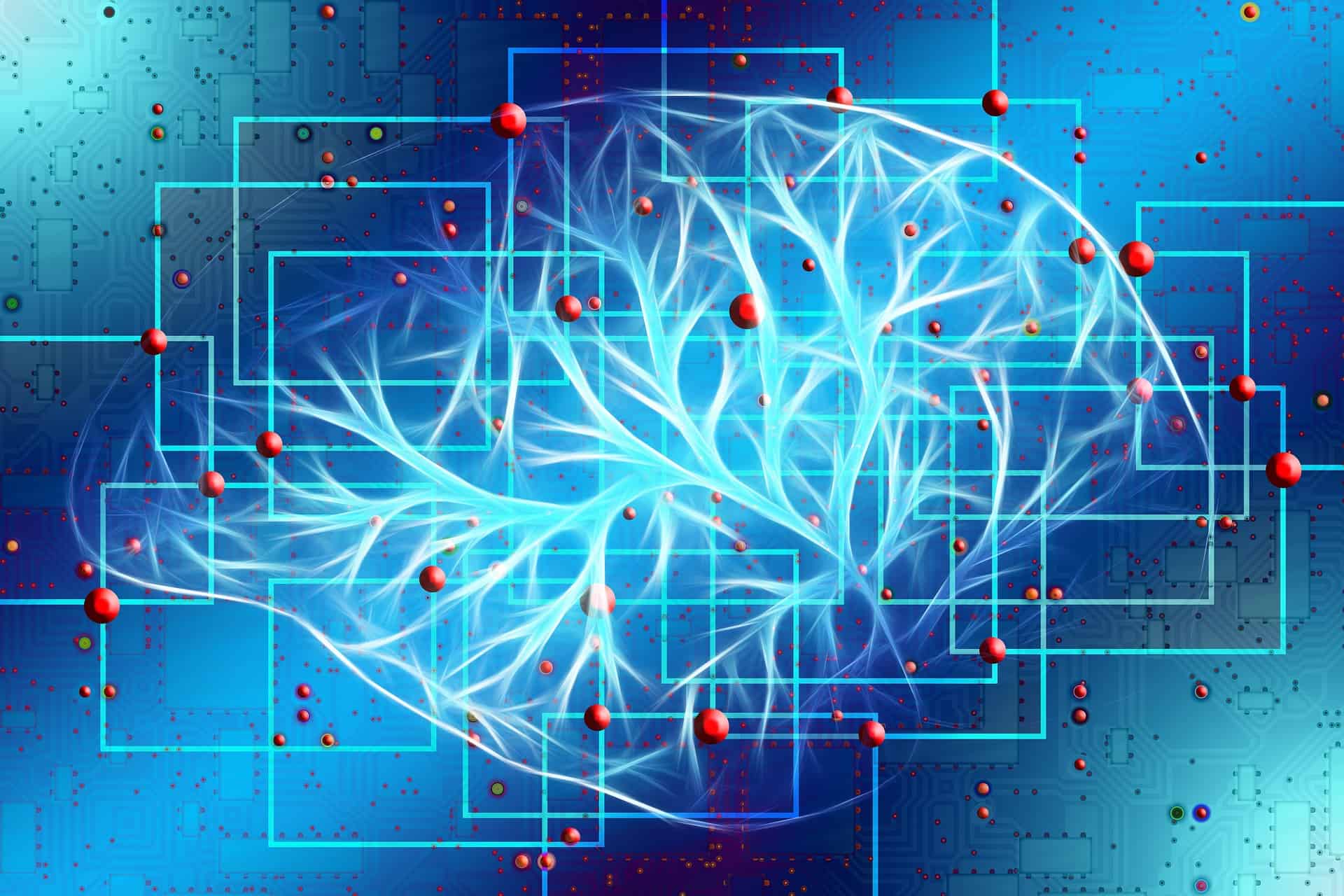
AI reasoning has a ‘black box’ nature. But researchers from the University of Geneva (UNIGE), the Geneva University Hospitals (HUG), and the National University of Singapore (NUS) have developed two new evaluation methods to help understand how an artificial intelligence (AI) makes decisions. The aim is to identify relevant portions of data and evaluate their relative importance with regards to the final prediction. This, in order to increase transparency and trustworthiness when used in medical applications or financial markets, says the UNIGE in a press release.
The progress of AI and deep learning in particular – which consists of training a machine using very large amounts of data with the aim of interpreting it and learning useful patterns – opens the pathway to increasingly accurate tools for diagnosis and prediction. However, the “black box” nature of AI technology raises important questions over trustworthiness.
‘‘The way these algorithms work is opaque, to say the least,’’ says Professor Christian Lovis, Director of the Department of Radiology and Medical Informatics at the UNIGE Faculty of Medicine and Head of the Division of Medical Information Science at the HUG, who co-directed this work. ‘‘Of course, the stakes, particularly financial, are extremely high. But how can we trust a machine without understanding the basis of its reasoning? These questions are essential, especially in sectors such as medicine, where AI-powered decisions can influence the health and even the lives of people; and finance, where they can lead to enormous loss of capital.”
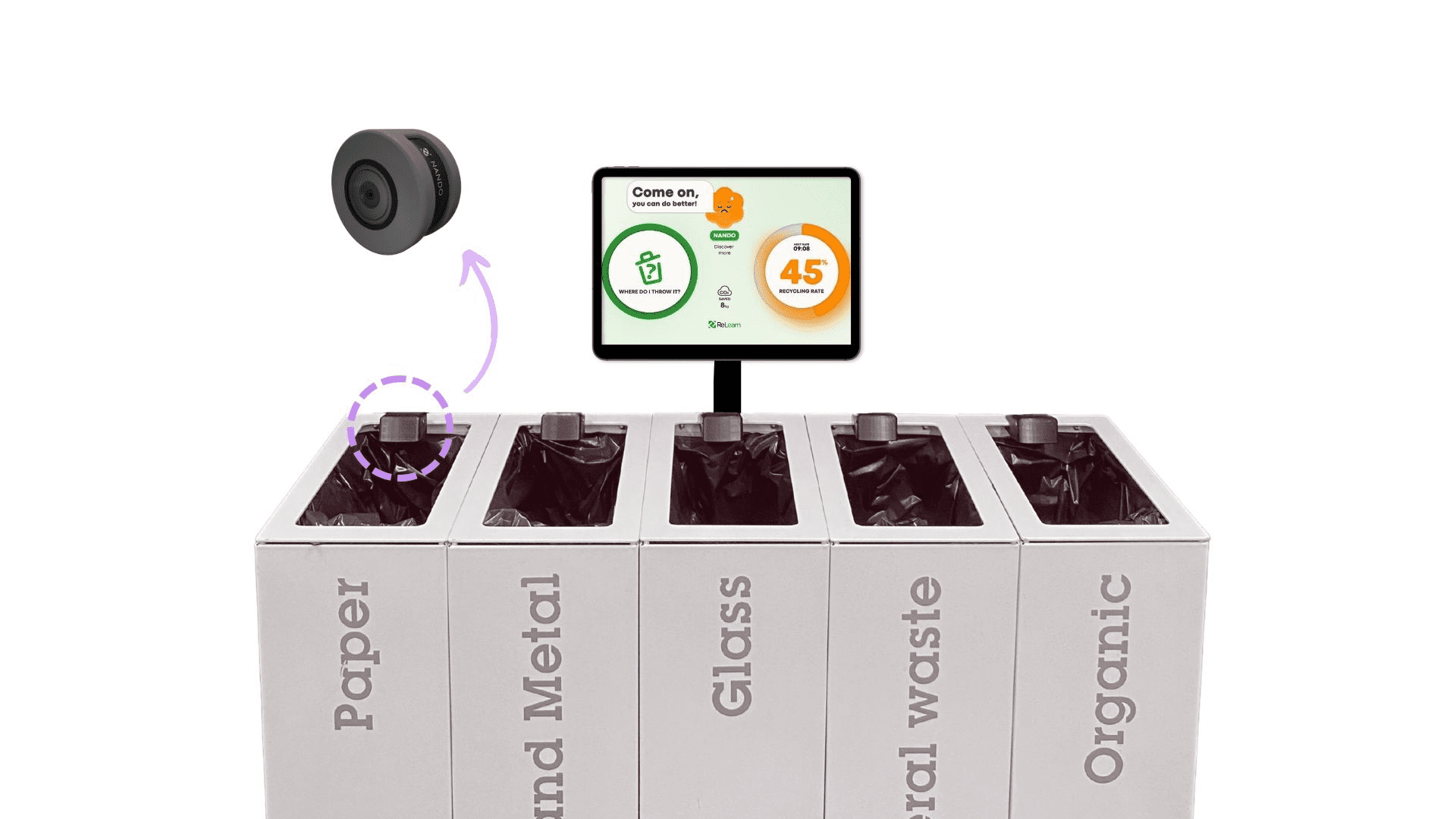
The Evaluation Method
Discriminating data is critical in developing interpretable AI technologies. For example, when an AI analyses images, it focuses on a few characteristic attributes. Doctoral student in Prof Lovis’ laboratory and first author of the study Hugues Turbé explains: “AI can differentiate between an image of a dog and an image of a cat. The same principle applies to analysing time sequences: the machine needs to be able to select elements – peaks that are more pronounced than others – to base its reasoning on. With ECG signals, it means reconciling signals from the different electrodes to evaluate possible dissonances that would be a sign of a particular cardiac condition.”
To evaluate interpretability methods, researchers developed two new evaluation methods: identification of relevant portions (IRP) and evaluation of their relative importance (ERI). The IRP method assesses how well an interpretability method identifies relevant portions of data – such as peaks in ECG signals – that are important for making predictions. The ERI method then evaluates whether these portions are accurately assessed in terms of their relative importance for the final prediction.
For example, when an AI predicts whether someone has a cardiac condition or not based on ECG signals, it is essential that it takes into account all relevant information – for example all peaks or valleys – and evaluates them accurately.
Relevance beyond healthcare
The new evaluation methods have been applied to various types of time series data such as ECG signals and financial markets. The team also tested their approach on AI models trained on images. They showed that this method can be used to evaluate interpretability methods for all types of data.
The research carries particular relevance in the context of the forthcoming European Union Artificial Intelligence Act which aims to regulate the development and use of AI within the EU. The findings have recently been published in the journal Nature Machine Intelligence.