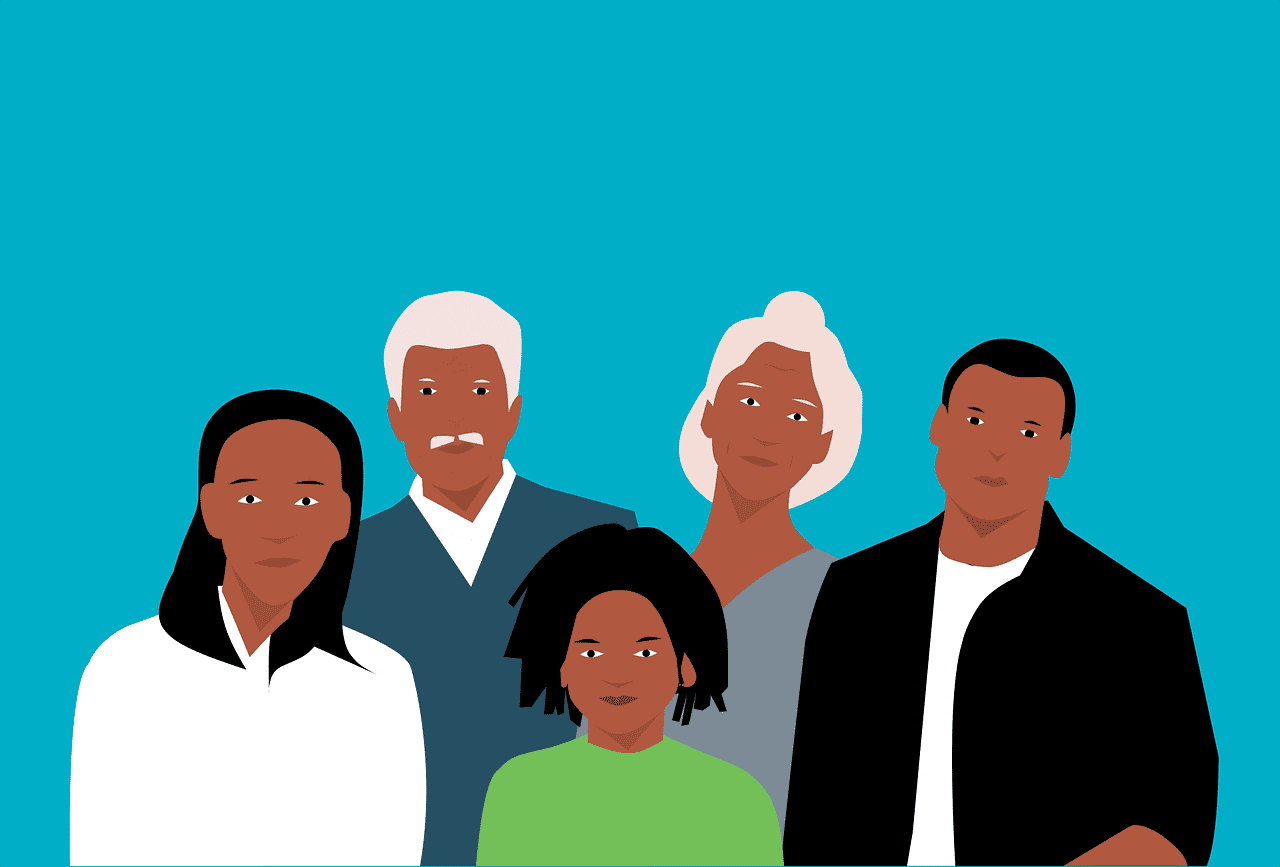
I am talking about “ageism,” which refers to biases, stereotypes, negative attitudes, and discrimination toward older people based on chronological age. I want to specifically dive deeper into “digital ageism” which concerns age-related biases in new technology.
Reading a journal article on health inequity introduced by AI and how the EU framework on medical devices can mitigate biases, inspired me to write about this topic. While I highly recommend reading the paper to get a good overview of the problems, the regulatory framework and some conclusions, I briefly summarise the ‘digital ageism’ introduced by medical AI and offer my reflections.
Technical AI ageism vs. contextual AI ageism
Having heard and read a lot about bias with AI, my mind goes first to data. Using datasets that no longer represent reality (historical bias), that do not represent certain population groups appropriately (representation bias), or, more simply, when data is inaccurate (measurement bias) can introduce a bias.
All three of these can occur for medical AI against older patients, for example, by using outdated data from old surveys when the internet was not as largely adopted or by training models with data from predominantly younger populations. At the modelling stage, there is algorithmic bias, when a model uses a biased variable to make a certain prediction (from the paper, e.g. using chronological age as a proxy for the willingness to undergo breast cancer surgery). Aggregation bias happens when one model is used for groups with different conditions, thus ignoring how diseases might show differently in older adults. Evaluation bias occurs when testing data does not match the population the model is meant for, such as using data from early-stage Alzheimer’s patients to test a device for late-stage patients.
What I found very interesting was learning about contextual bias, a bias introduced by the context in which medical AI is used. For example, if patients are required to use apps or enter health data online, older patients are at a disadvantage. Prejudices and stereotypes are also part of this category. For instance, a doctor could avoid recommending a technological solution to an older patient on the assumption that they are not tech-savvy.
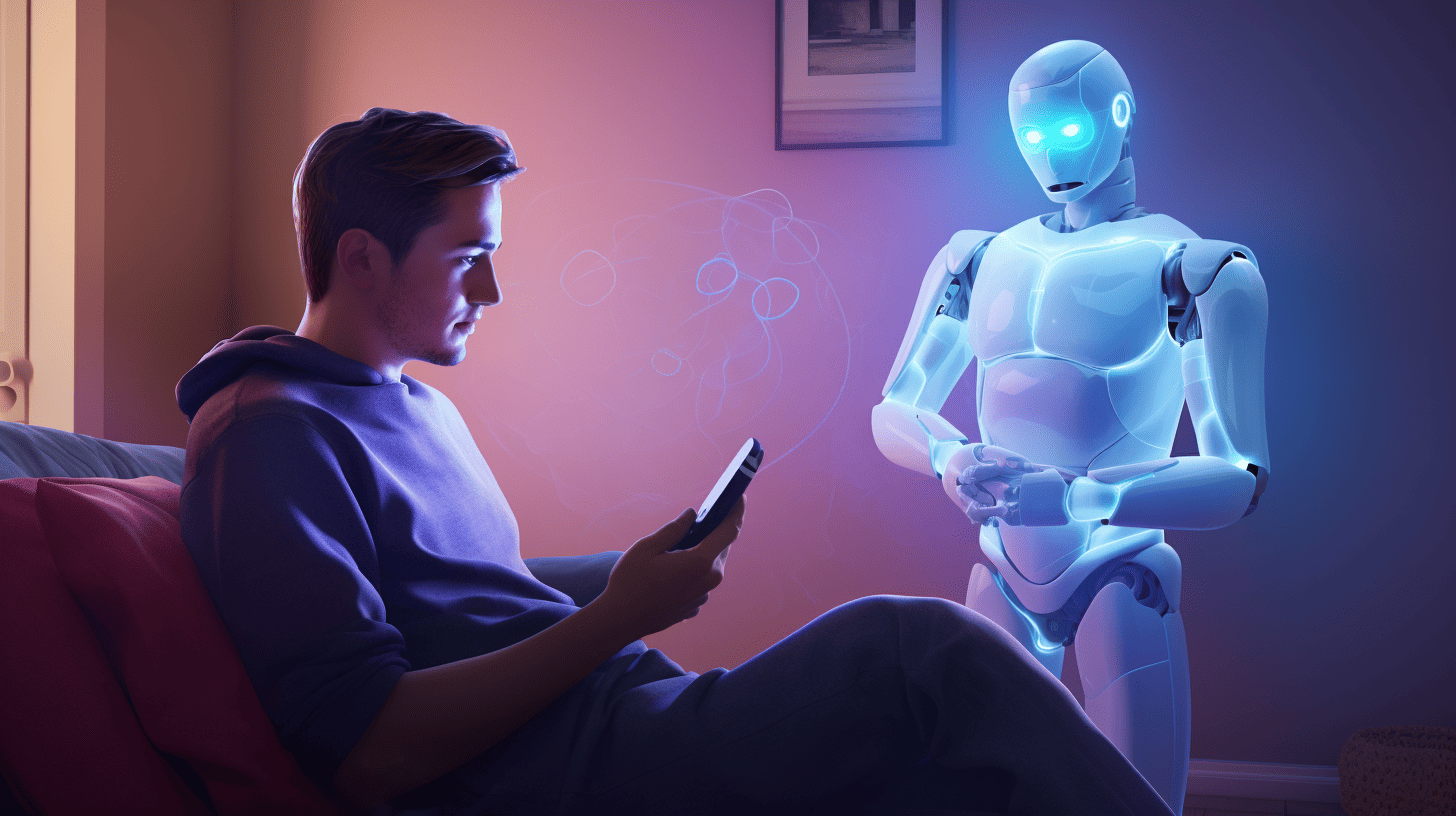
‘Help with Digital’
Before reading the paper, I had thought about the issue when spending time at the public library in Amsterdam. Did you know that among other services, they offer a “Hulp bij Digitaal” (Help with Digital)? It is a resource where individuals can seek assistance with things like DigiD and online shopping or practice using a computer independently with staff support if needed. While the service is not explicitly targeted to older individuals (which I believe is justified, considering digital literacy levels vary for many reasons), I immediately recognized its importance for inclusivity, especially for the ageing population. Initiatives such as this one help build the context to adopt these solutions for essential tasks, such as tax payments.
Having lived in the Netherlands for several years, I’ve noticed a great disparity in digitalization for government, postal, and healthcare services in Italy compared to here. While progress is being made, more than merely introducing technology is needed; creating an environment where people feel comfortable and confident using it is crucial. This highlights the importance of design in implementation, a factor often overlooked.
The role of design
In this context, design carries significant weight and responsibility. Technological advancements in this field are so rapid that there is a need for focused expertise to make these assessments. Taking the time to carefully consider the context and technical aspects before releasing a product can greatly enhance its adoption and value.
When considering design, particularly regarding the contextual AI matter, there are many factors that have an influence and questions that can be asked, but to start, I wonder how older people are defined. Of course, there are official guidelines that refer to certain age brackets, but I wonder whether they are fair in design contexts, considering they are closely linked to being tech-savvy, for example. And tech-savviness varies widely over time and location since the population is becoming more digital, but this progress differs greatly from one country to another and depends on many other factors.
Looking ahead, when only digital natives remain, contextual ageism may look completely different. This shift offers some hope, suggesting it might not represent as significant a problem. However, this doesn’t mean similar issues may not arise with new technologies, nor does it justify overlooking the current concerns. Even with generations of digital natives, universal access to technology and digital literacy remains a challenge.
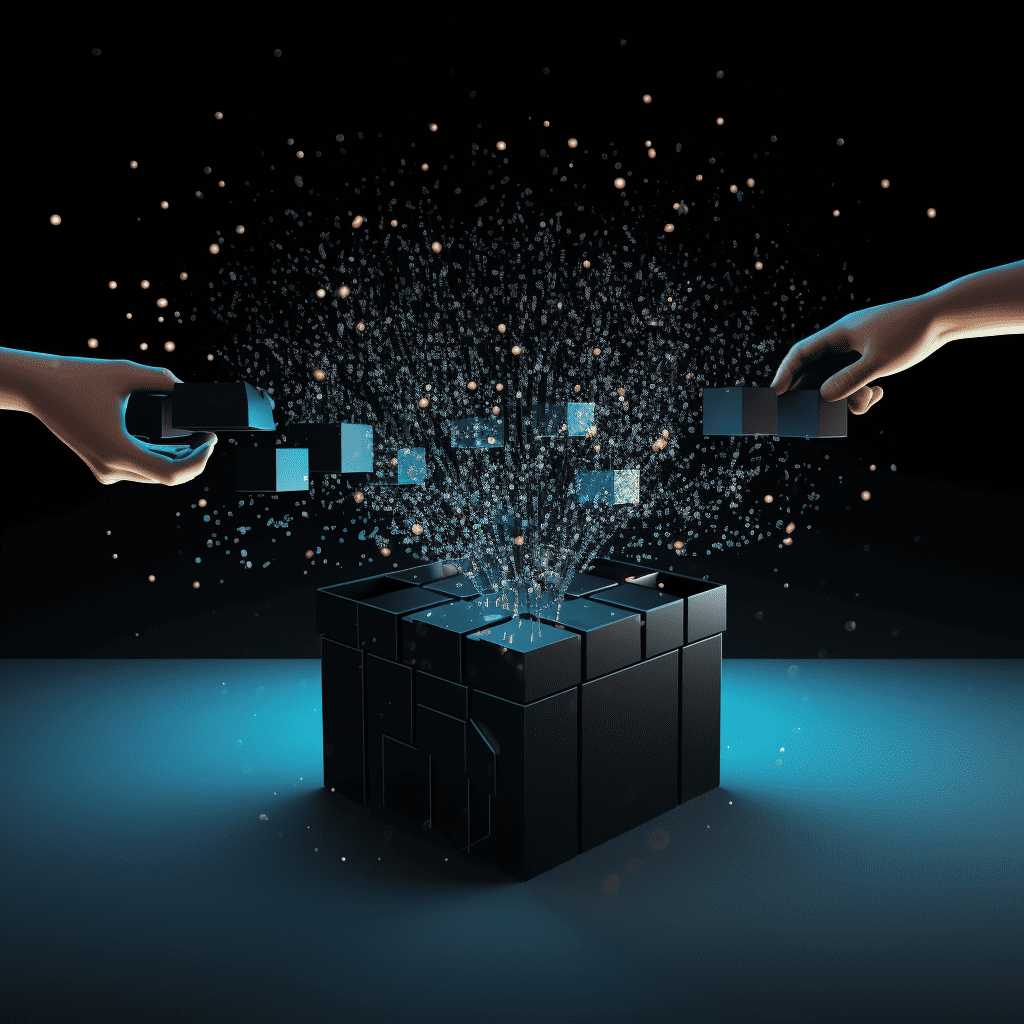