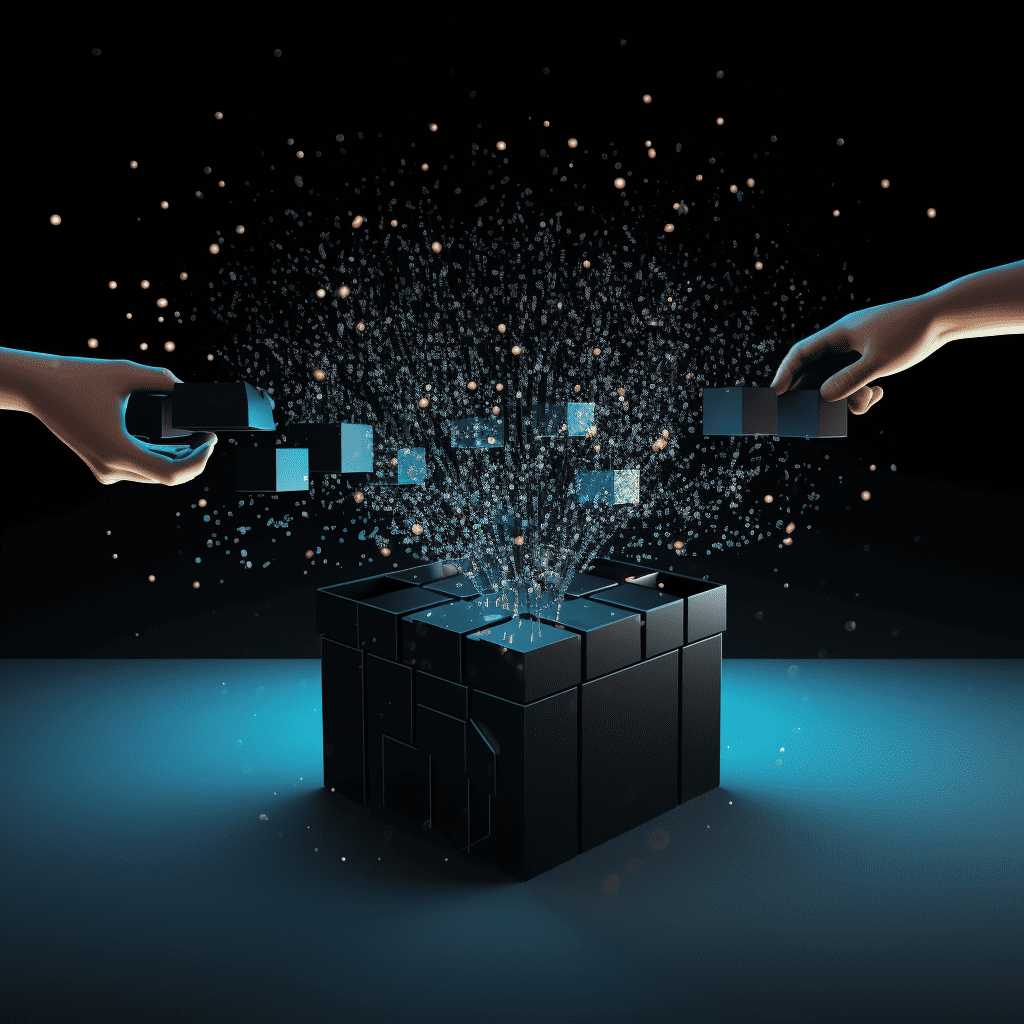
I have scrolled through many LinkedIn posts, read articles and attended a couple of panel discussions about the need of explainability and transparency when working with AI models to ensure their safety and trustworthiness. The general idea and overall accepted view is that we do need to be able to explain the AI outputs and trace the model inner workings somehow. Depending on which stakeholder the level of explainability can vary, but there should be a sort of baseline.
For this reason, I was very intrigued when I stumbled upon a podcast episode of Ethical Machines by Reid Blackman titled “In Defense of Black Box AI”. Black box AI, as the name suggests, refers to AI systems that generate outputs or predictions without disclosing the underlying mechanisms behind the outcomes. The guest of this episode was Kristof Horompoly, Head of Responsible AI at JPMorgan, one of the largest banks in the world. The perspective discussed was opposite to what I had seen until then: What if we cared more about maximising AI performance without compromising on complexity for the sake of explainability?
Explainability VS performance
From a technical perspective explainability can come with a trade-off with performance, for certain AI-models. Beyond performance, explainability is time-consuming, increases time to market, can be expensive, and has an environmental impact. Therefore, optimising for performance might outweigh the need for explainability in certain contexts. Instead, we could accept the technology by mapping its input and output spaces, thus checking for accuracy and fairness without understanding the model’s inner workings. The episode analogy describes how we all drive a car without necessarily all being able to know the mechanics of it. I highly recommend checking out the entire episode, as it also dives into simply explained technicalities.
Black box AI for medical use
I was surprised by these learnings, which got me thinking about black box AI for medical use. Both speakers acknowledged that explainability is imperative in regulated sectors such as healthcare or finance to spot biases and mistakes and rectify them. For instance, if an AI-driven conclusion on diagnostic imaging disproportionately impacts a specific ethnicity, explainability becomes pivotal in identifying and addressing such biases. Nonetheless, introducing explainability in the AI workflow does pose an additional step, raising concerns about the practicality and efficiency of AI integration. If we scrutinise AI results for biases, it may seem counterintuitive to leverage AI in the first place.
So, playing the devil’s advocate, what would make it possible to eliminate the need for explainability and focus entirely on the performance of AI in healthcare? How could we make sure that the input and output mapping is not introducing some unfairness? Thinking of the example of imaging diagnostics, AI has shown promise because of its pattern recognition abilities, spotting details, and subtle differences that might be challenging for humans to notice. But are we sure the models are being trained on data relevant to the population we are using the device for? Ethicists can and should work in the data collection and processing phase, advocating for ethical data practices and addressing issues related to dataset bias. However, for now, it is up to a manufacturer to make these ethical considerations and implement them as there are currently no regulations on AI.
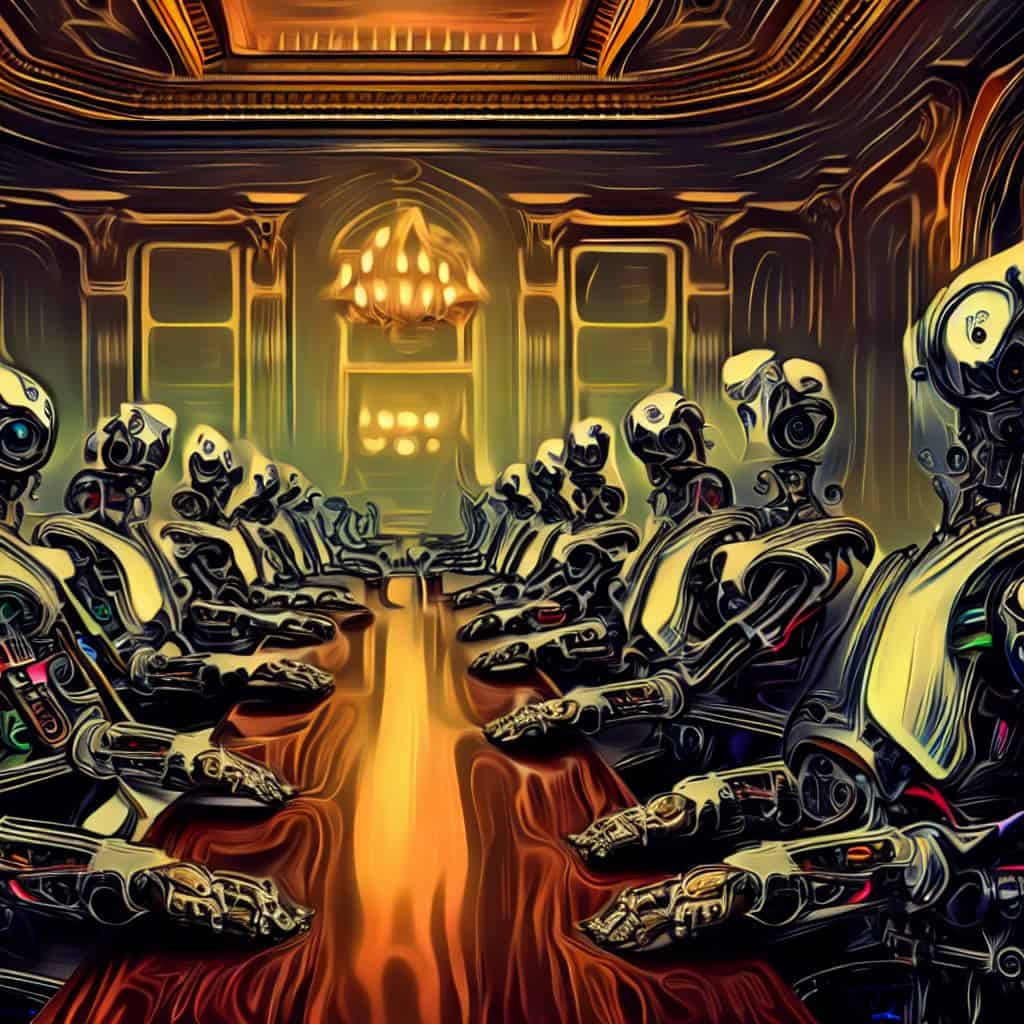
From overarching guidelines and principles to concrete rules
Looking at the medical device industry specifically, the EU Medical Device Regulation (EU MDR) represents the current regulatory framework, which has significantly transformed the European market since its introduction in 2017. This regulation has prompted all companies to prioritise compliance swiftly. It mandates enhanced monitoring and continuous data collection to demonstrate the safety and efficacy of devices. However, it does not prescribe specific details on what data to collect or how to collect it. Instead, it establishes overarching guidelines and principles that manufacturers must follow in gathering and handling medical device-related data.
What if subject matter experts could come up with thresholds and standards regarding amounts of data, which data to collect and how to label it to introduce into the regulation for medical devices? Could the introduction of a black box AI model in healthcare be possible if we had more control over avoiding unfair and biased outputs? The regulation may have to slowly branch to specific applications, for example the previously mentioned imaging diagnostics.
A group of experts could iterate and develop a concrete set of requirements for the data collection phase that would allow for the development of a safer model from an ethical perspective from the get-go. In this way, we would not have to compromise on explainability methods and less performant algorithms. The effort of continuous monitoring of the outputs would then follow, but it is less costly, faster and already done in compliance with the EU MDR.
With this opinion piece, I want to challenge the perspective of concentrating most efforts for ethical AI applications at the implementation phase, in favour of taking time to build safe AI models from the data collection and handling phase.
This could mean waiting some years to roll out a black box AI system after regulating the data collection with concrete rules from ethical and technical perspectives and collecting the data accordingly. However, a demonstrated close collaboration with ethical teams in AI development could enhance acceptance of AI within the healthcare sector.