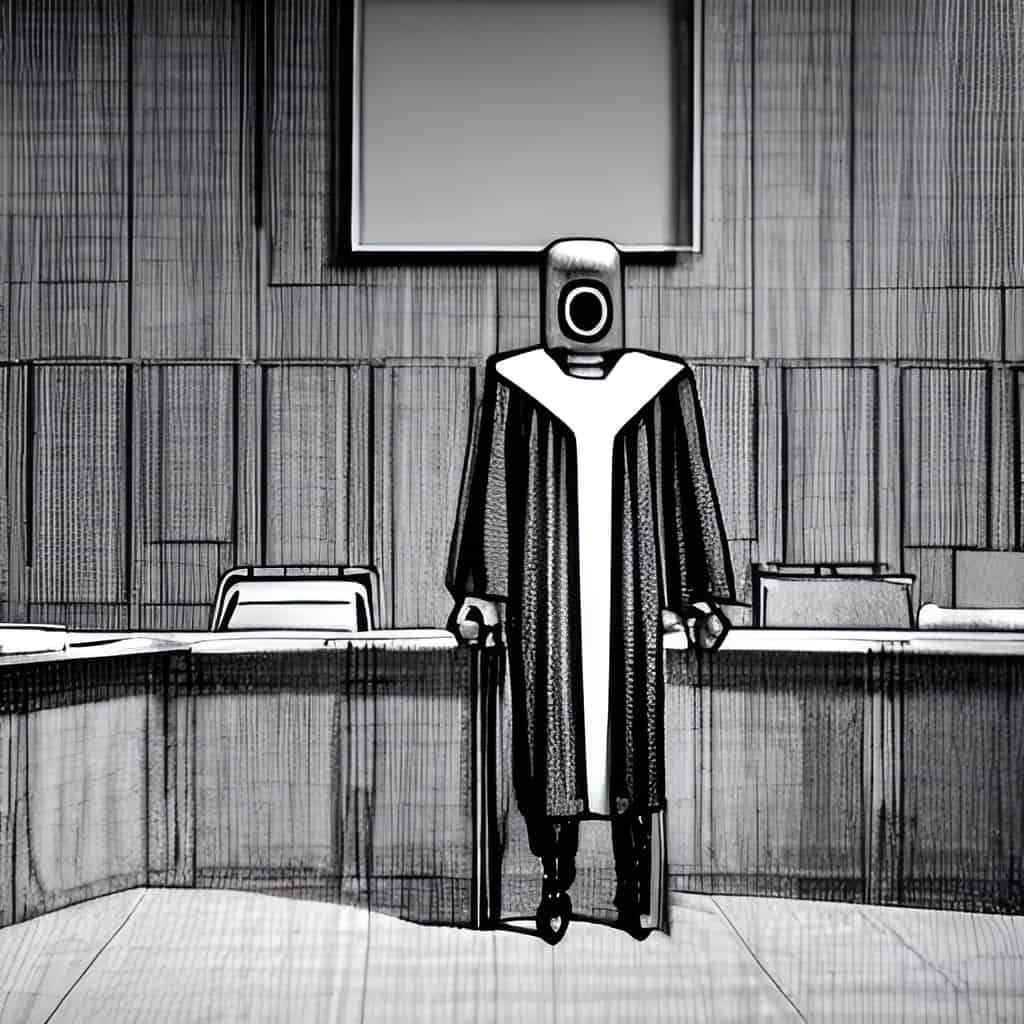
MIT researchers have discovered that machine-learning models mimicking human decision-making often make harsher judgements than humans, due to being trained on the wrong data. Models should be trained on “normative data” (labelled by humans for rule defiance), but are typically trained using “descriptive data” (factual features labelled by humans), leading to over-prediction of rule violations. This inaccuracy can have serious real-world consequences, such as stricter judgements in bail or sentencing decisions. The study highlights the importance of matching training context to deployment context for rule violation detection models and suggests that dataset transparency and transfer learning could help mitigate the problem.
The human-AI fairness gap
A separate study involving 6,000 US adults examined views on AI judges, revealing that while AI judges were perceived as less fair than human judges, the gap could be partially offset by increasing the AI judge’s interpretability and ability to provide a hearing. Human judges received an average procedural fairness score of 4.4 on a 7-point scale, while AI judges scored slightly below 4. However, when an AI-led proceeding offered a hearing and rendered interpretable decisions, it was seen as fair as a human-led proceeding without a hearing and uninterpretable decisions.
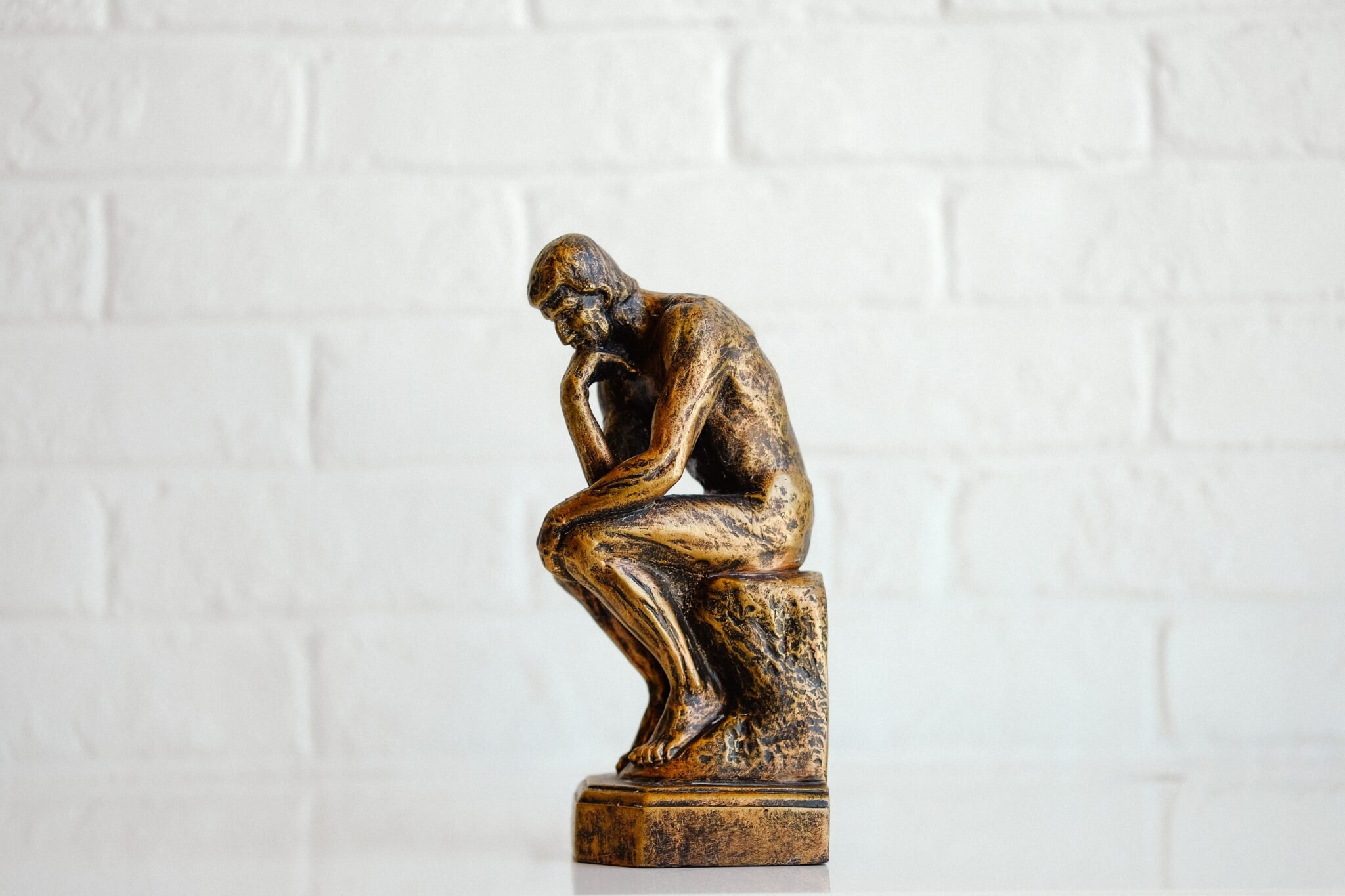
As AI tools like ChatGPT demonstrate higher accuracy in certain domains, such as tumor classification, and pass legal reasoner tests like Minnesota Law School exams, the human-AI fairness gap may continue to narrow. In some cases, advanced AI decisions are seen as fairer than human judicial decisions, suggesting that future AI judging developments might result in AI proceedings being generally perceived as fairer than human proceedings.
AI and blockchain in the courtroom
AI-driven legal services are gaining traction, with platforms like LegalZoom providing consumer-level automated legal services. AI has the potential to reduce human bias, emotion, and error in legal settings, addressing the “access-to-justice gap” experienced by low-income Americans. University of Toronto Professor Gillian K. Hadfield states that AI reduces cost and helps address the access to justice crisis. However, she also acknowledges that more work is needed before AI becomes common in courthouses due to the law’s intolerance for technical errors.
Blockchain technology is also making its way into legal services. Public blockchains offer transparency, trust, and tamper-free ledgers, with strengths like traceability and decentralization complementing AI to generate trust and provide valuable information about origin and history. Smart contracts are expected to play a role in the evolving legal system, with many commercial contracts likely to be written as smart contracts in the near future. 2Decentralized justice systems, such as Kleros, use blockchain-based arbitration solutions with smart contracts and crowdsourced jurors.
Addressing the issue: Dataset transparency and transfer learning
Improving dataset transparency is one way to address the problem of harsh AI judgements. If researchers know how data were gathered, they can ensure the data are used appropriately. Another possible strategy is transfer learning – fine-tuning a descriptively trained model on a small amount of normative data. This approach, as well as exploring real-world contexts like medical diagnosis, financial auditing, and legal judgments, could help researchers ensure that AI models accurately capture human decision-making and avoid negative consequences.
In conclusion, AI models making harsher judgements on rule violations due to descriptive training data instead of normative data can have real-world implications, such as stricter judicial sentences and potential negative impacts. Researchers suggest improving dataset transparency, matching training context to deployment context, and exploring real-world applications to ensure AI models accurately replicate human decision-making.