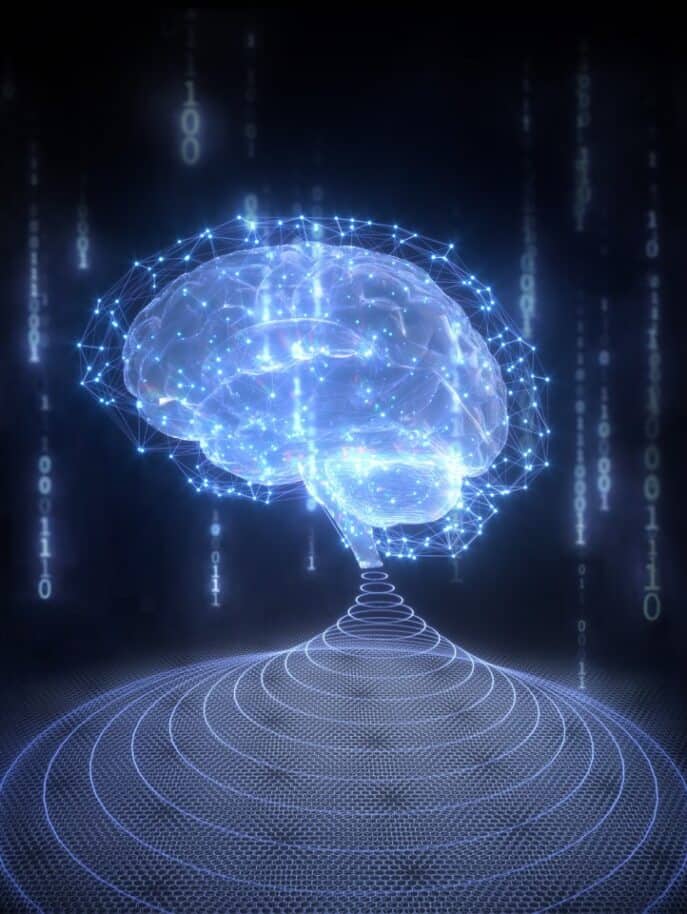
Researchers from Northwestern University, Boston College, and MIT have unveiled a revolutionary synaptic transistor, marking a significant leap in artificial intelligence. The device emulates human brain function, performing associative learning and recognizing patterns under imprecise conditions, all at room temperature. This innovation promises energy-efficient, fast processing with memory retention even without power, addressing the high energy demands of current digital systems. Published in Nature, the study introduces a technology that could transform AI by facilitating higher-level thinking and drastically reducing power consumption.
Why you need to know this
As AI applications surge, disruptive technologies are needed to foster these developments. Therefore, paradigm-shifting technologies such as the Northwestern University one are worth keeping an eye on.
Reshaping the future of artificial intelligence
In the realm of artificial intelligence, a new era beckons with the advent of a synaptic transistor that mirrors the human brain’s operational efficiency. The transistor not only handles simple machine-learning tasks but is also adept at associative learning, distinguishing it from its predecessors.
Conventional computing systems, with their distinct processing and storage units, encounter a bottleneck known as the von Neumann bottleneck. This separation leads to excessive energy consumption during data-intensive operations. The newly developed synaptic transistor overcomes these limitations by integrating memory and processing capabilities. This integration is critical for facilitating the complex tasks associated with artificial intelligence and machine learning.
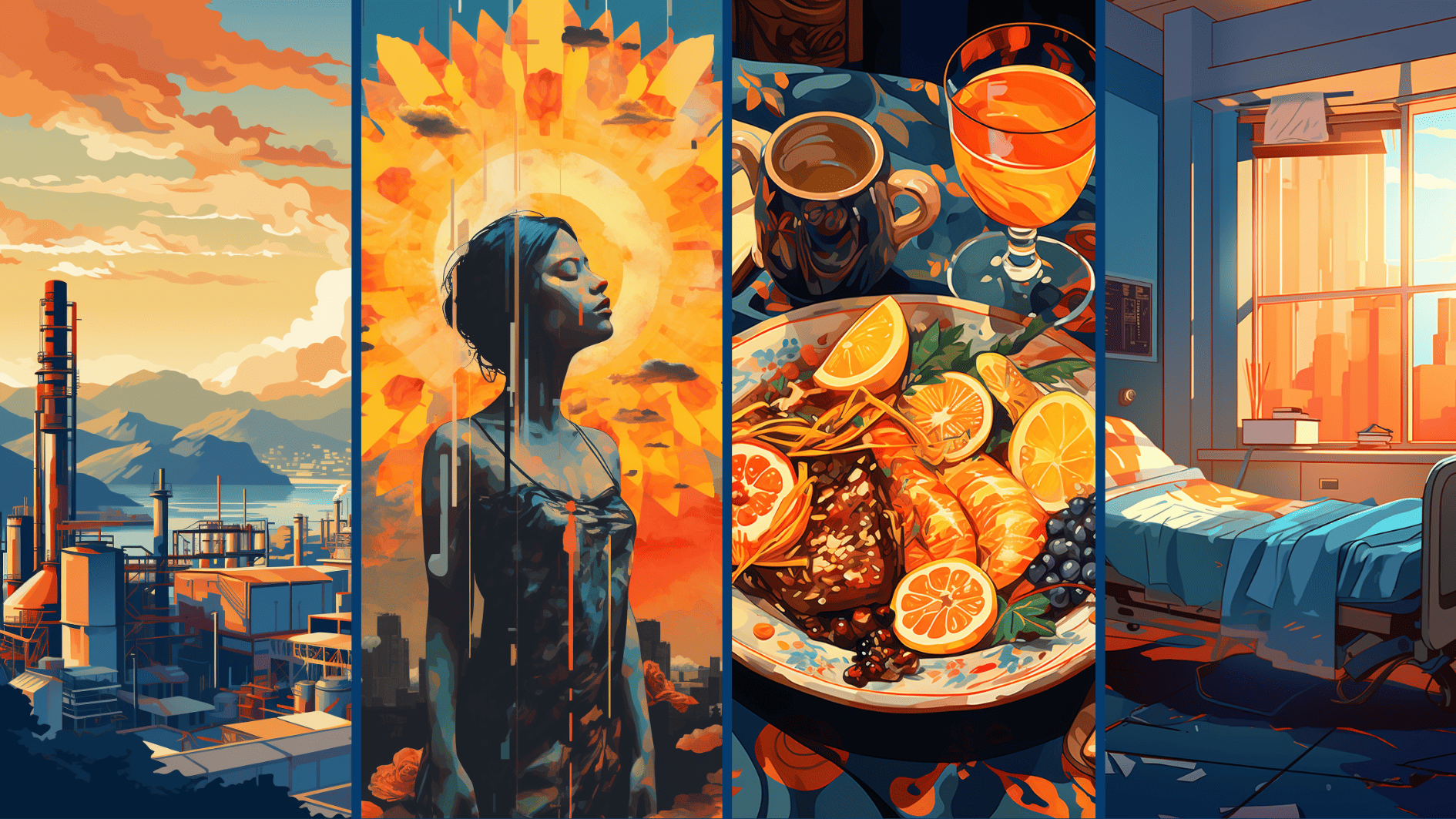
A leap in technology and efficiency
At the core of this breakthrough is the unique use of bilayer graphene and hexagonal boron nitride. These materials form a moiré pattern, which imparts unprecedented tunability to the electronic properties of the device. This tunability is a significant departure from traditional methods, which rely on scaling down the size of transistors to achieve better performance.
Mark C. Hersam, who co-led the research, emphasizes that the quest for greater efficiency in computing hardware is becoming increasingly urgent. The traditional approach of packing more transistors into integrated circuits has led to notable successes. However, with the era of big data upon us, the energy demands of digital computing are soaring, potentially overwhelming the power grid. Hersam’s work with the synaptic transistor aims to address this concern by rethinking the way we approach AI and machine-learning tasks.
Advancing towards higher-level cognitive functions
Artificial intelligence strives to replicate human thought processes. One of the fundamental aspects of such cognition is the ability to classify data. Hersam’s team has propelled AI technology towards this objective by introducing a device that analyzes and categorizes data in a way that mimics higher-level thinking.
The transistor’s ability to perform associative memory tasks is remarkable. It successfully recognized similar patterns even when the input was imperfect, showcasing an attribute akin to the human brain’s tolerance for ambiguity and error. This capability could pave the way for AI that can deal with the complexities and nuances of real-world data, a significant step towards AI that can truly think like a human.
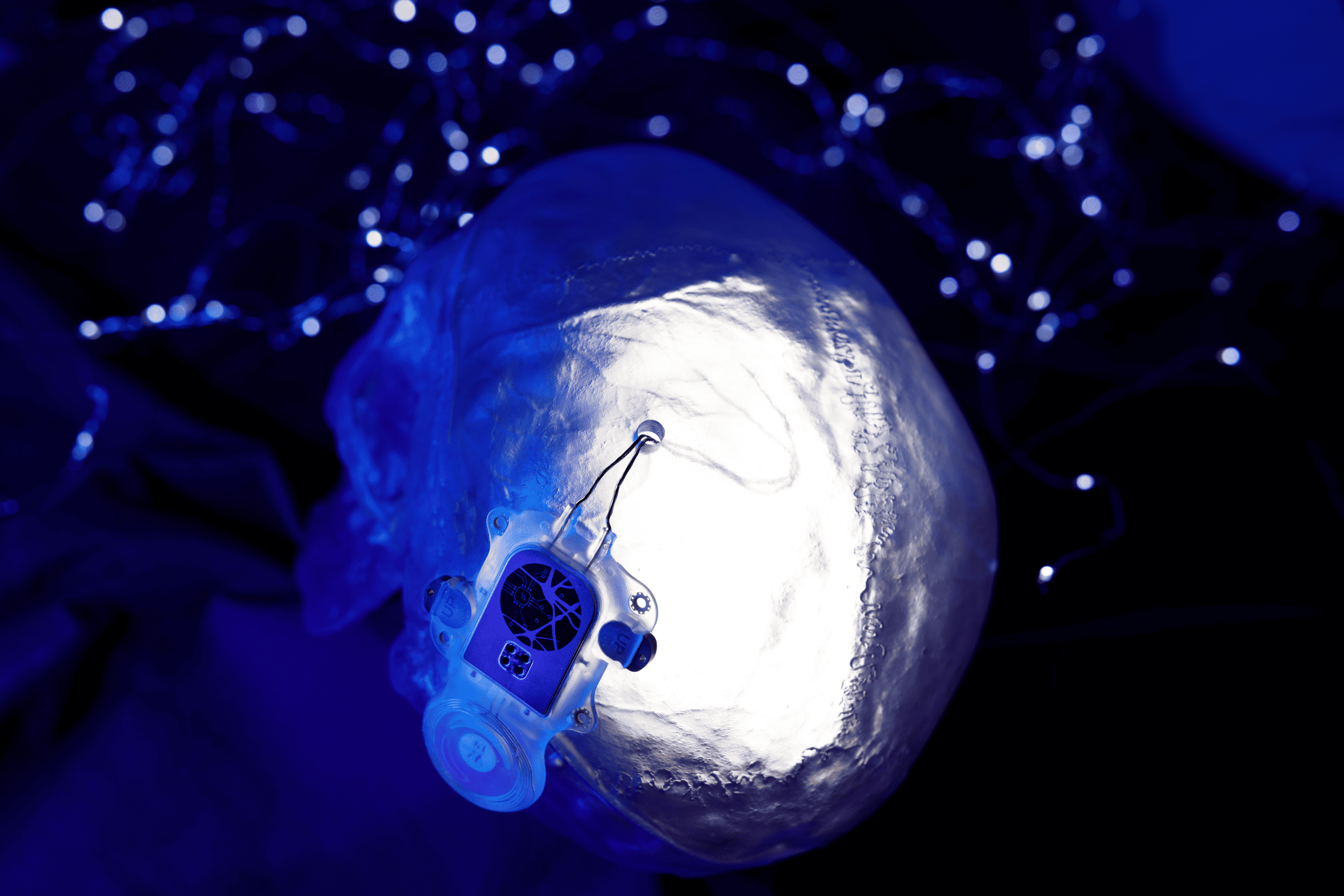
Implications for the future of computing
The implications of this research are vast. With a device that operates at room temperature, consumes less energy, and retains information without power, the potential applications are wide-ranging. This technology could lead to more efficient personal computing devices, advanced neural networks, and even new forms of data storage and retrieval.
Moreover, the synaptic transistor could be instrumental in addressing some of the most pressing challenges in computing today. The energy savings alone would have a substantial impact on the environment, reducing the carbon footprint of data centers and other infrastructure that support the digital economy. With AI and machine learning set to play increasingly prominent roles in various sectors, the need for more energy-efficient computing hardware cannot be overstated.