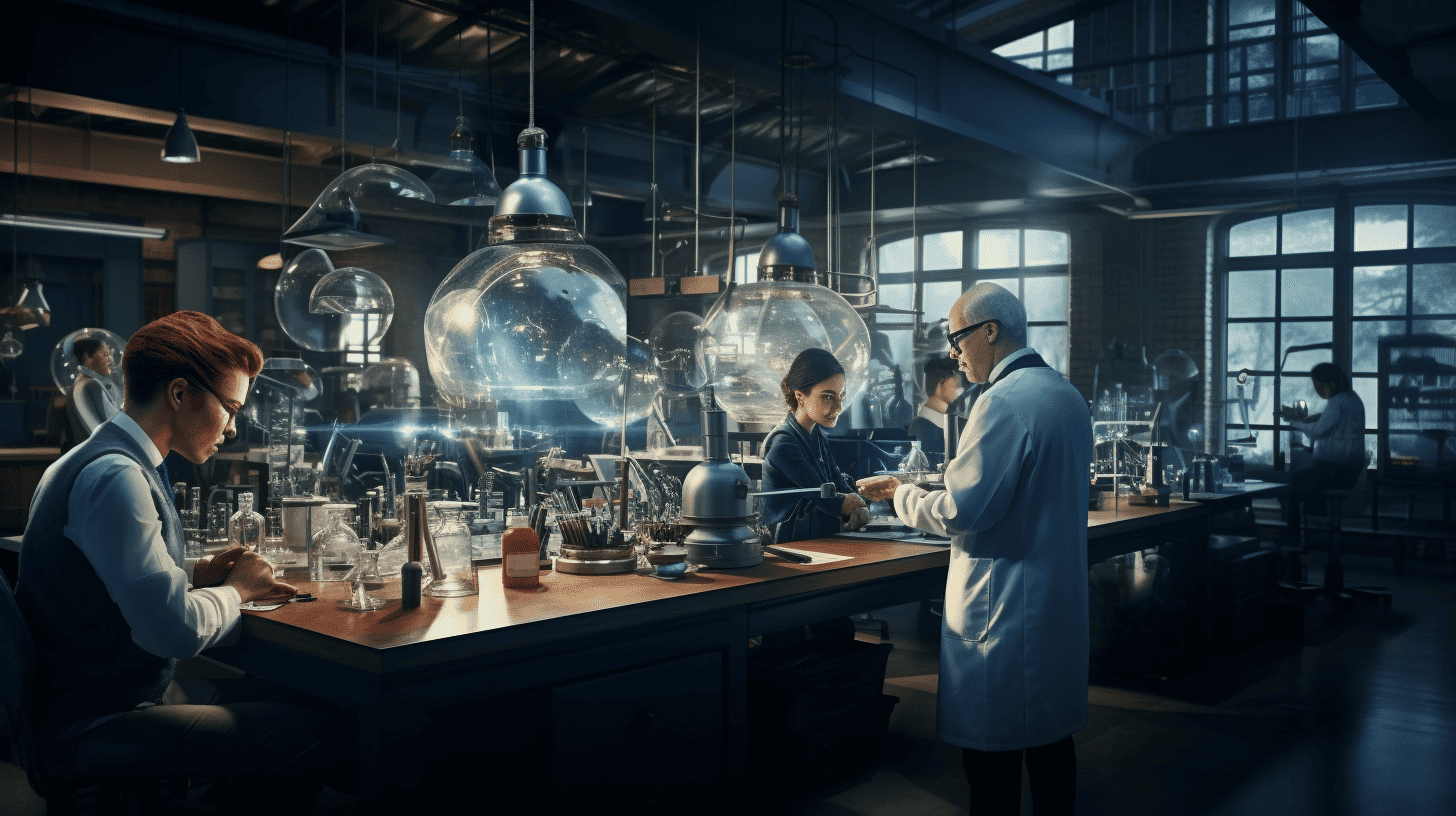
Discovering and developing new materials, a room temperature superconductor, the optimal battery design: artificial intelligence is poised to take us to the places that long seemed out of reach. And maybe more important than anything else: the time needed to get health discoveries from a research stage to clinical trials will drop from many years to just weeks. “We’re a couple of years away from having the first truly AI designed drugs for major diseases like cardiovascular or cancer.”
Why is this important?
AI’s increased usage is encountering mixed reactions, with public concern higher than excitement. In these circumstances it’s crucial to point out the potential, like Demis Hassabis does.
Demis Hassabis, the head of Google’s artificial intelligence division and co-founder of DeepMind, is expecting artificial intelligence to be the booster of big steps in many fields. On last week’s Hard Fork podcast, he elaborates on what AI according will be capable of in the next couple of years.
In the ever-evolving landscape of artificial intelligence, Google’s AI division, led by Demis Hassabis, stands at the forefront of pioneering the seamless integration of AI into practical applications. As DeepMind continues to push the boundaries of what AI can achieve, Hassabis sheds light on the transformative journey from specialized, handcrafted AI solutions to the utilization of general AI techniques for product development. This shift not only marks a significant convergence of research and product tracks within Google but also heralds a new era where AI’s capabilities are directly aligned with solving real-world challenges. “Today, the research tracks and the product tracks have converged. They’re one and the same now.”
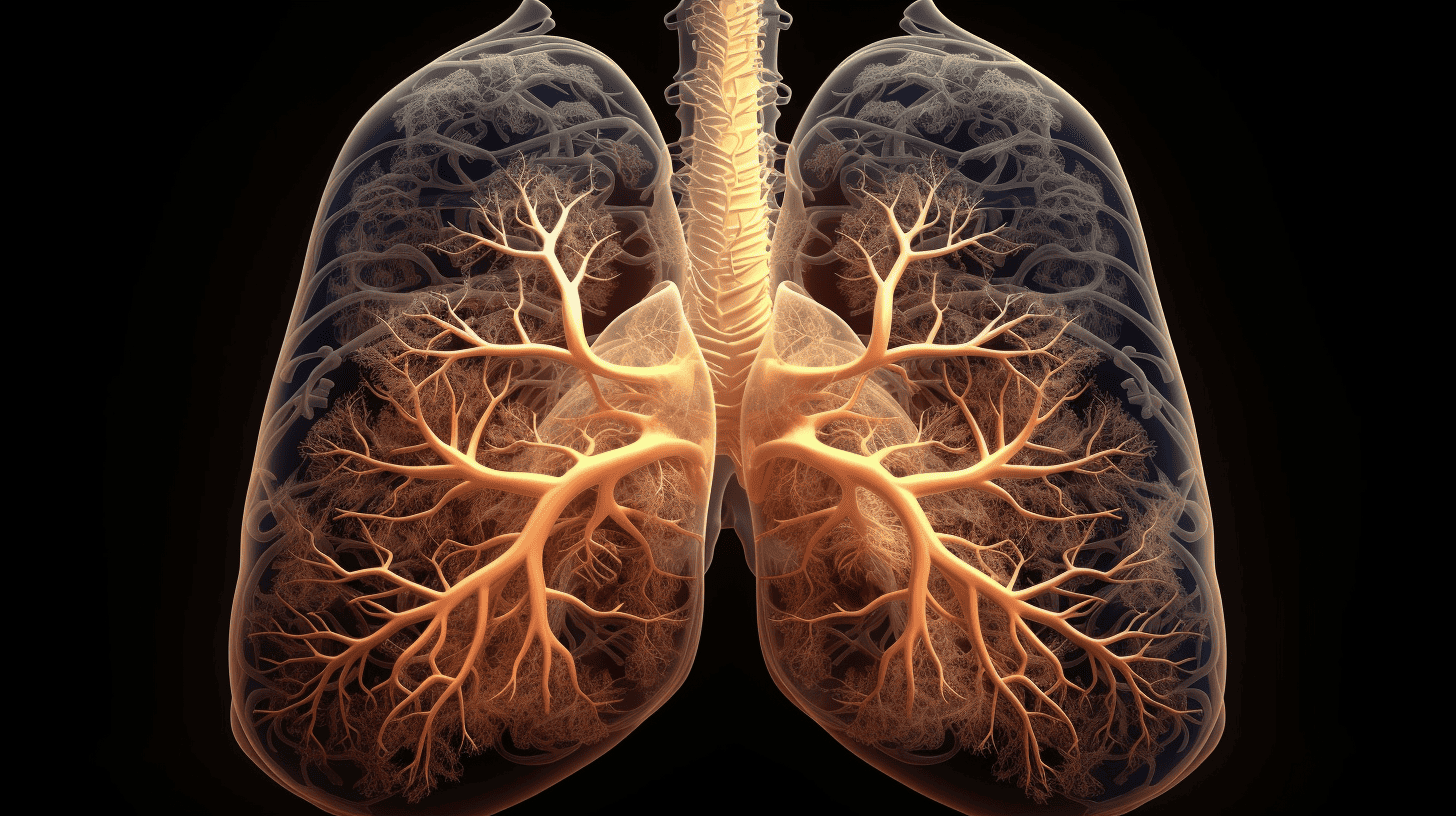
Feedback loops
Hassabis emphasizes the critical role of feedback loops between AI research and application, suggesting that real-world usage and user feedback are invaluable in refining AI models. “It’s actually really good for research to have tight feedback loops with grounded, well-designed applications, because that is the way you really understand how your models are doing. You can have academic metrics coming out of your ears, but the real test is when millions of users use your product. Do they find it useful? Is it beneficial to the world? And you get obviously a ton of feedback that way, and then that leads to very rapid improvements in the underlying models.”
You can have academic metrics coming out of your ears, but the real test is when millions of users use your product.
Demis Hassabis
This approach has accelerated the pace of innovation within Google and paved the way for breakthroughs such as AlphaFold. AlphaFold’s success in predicting protein structures has vast implications for drug discovery, showcasing AI’s potential to revolutionize the pharmaceutical industry. With projects like Isomorphic Labs, which aims to leverage AI in designing effective drugs with minimal side effects, Hassabis is optimistic about the near future, where AI-designed medicines for major diseases like cancer and cardiovascular illnesses will enter clinical trials.
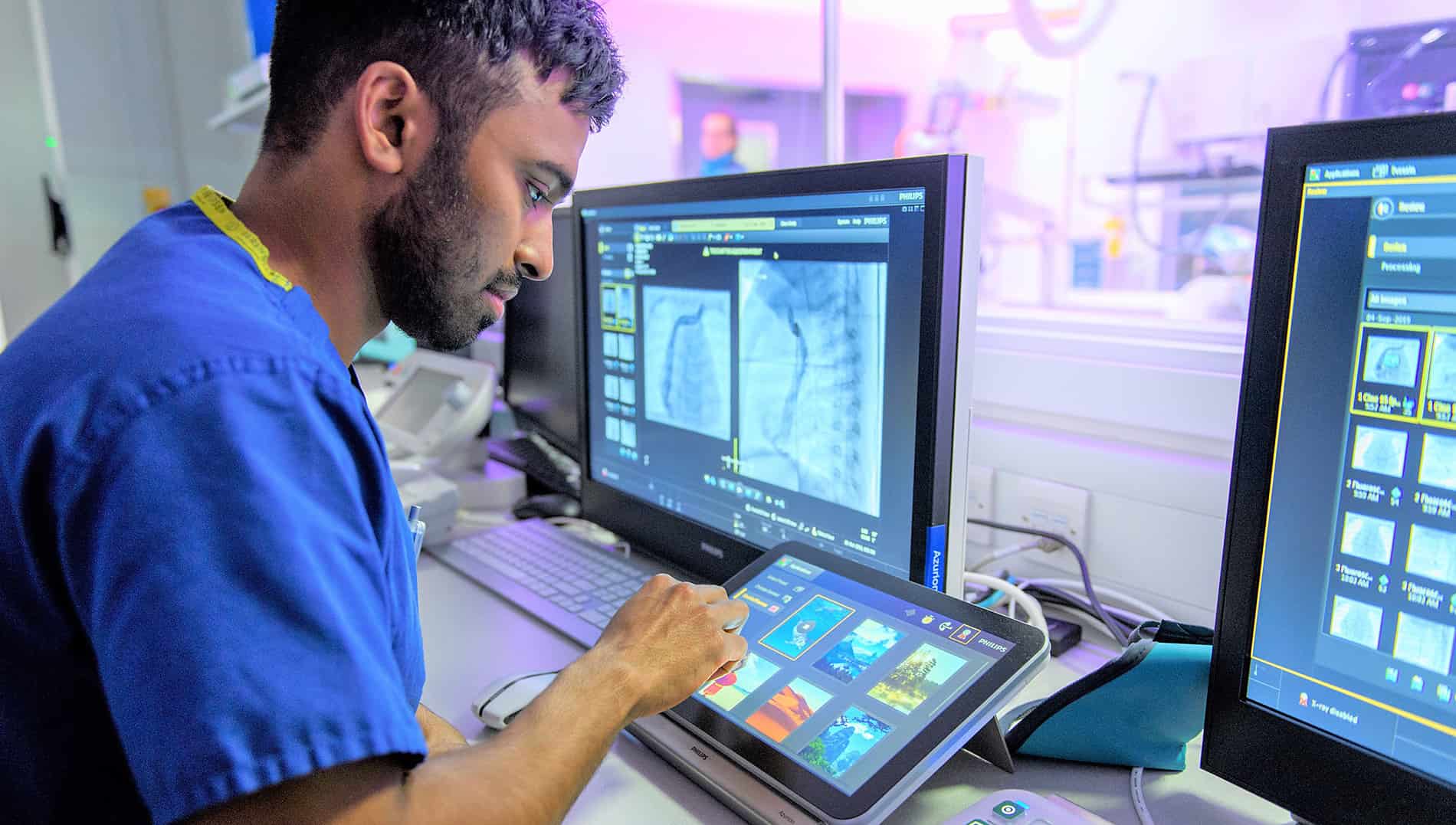
Mixed sentiments
Despite the optimism within the tech community, public sentiment towards AI remains mixed. A survey by the Pew Research Center revealed a predominant concern over excitement for AI’s increased usage. Hassabis believes that the key to shifting this perception lies in demonstrating the tangible benefits of AI through impactful applications like AlphaFold and its implications for medical advancements. “I think people are always worried about change, or disruption, and clearly, AI will bring enormous change. It’s dawning on people, but they haven’t interacted with it. What we need to do, as a field, is present concrete use cases that are clearly incredibly beneficial. The average person in the street probably doesn’t know about AlphaFold yet, what that impact will be, but they will do if that leads to AI designed drugs and cures for really terrible diseases. And I think we’re only just a few years away from that.”
With Isomorphic Labs, Hassabis wants to take the AlphaFold technologies into chemistry and biochemistry to actually design drugs to bind to the right parts of the protein structures that AlphaFold already predicted. “We’ve just signed big deals with with Big Pharma, on real drug programs. I expect in the next couple of years, we’ll have AI designed drugs in the clinic and clinical testing. That’s going to be an amazing time. That’s when people will start to really feel the benefits in their daily lives in really material and incredible ways.”
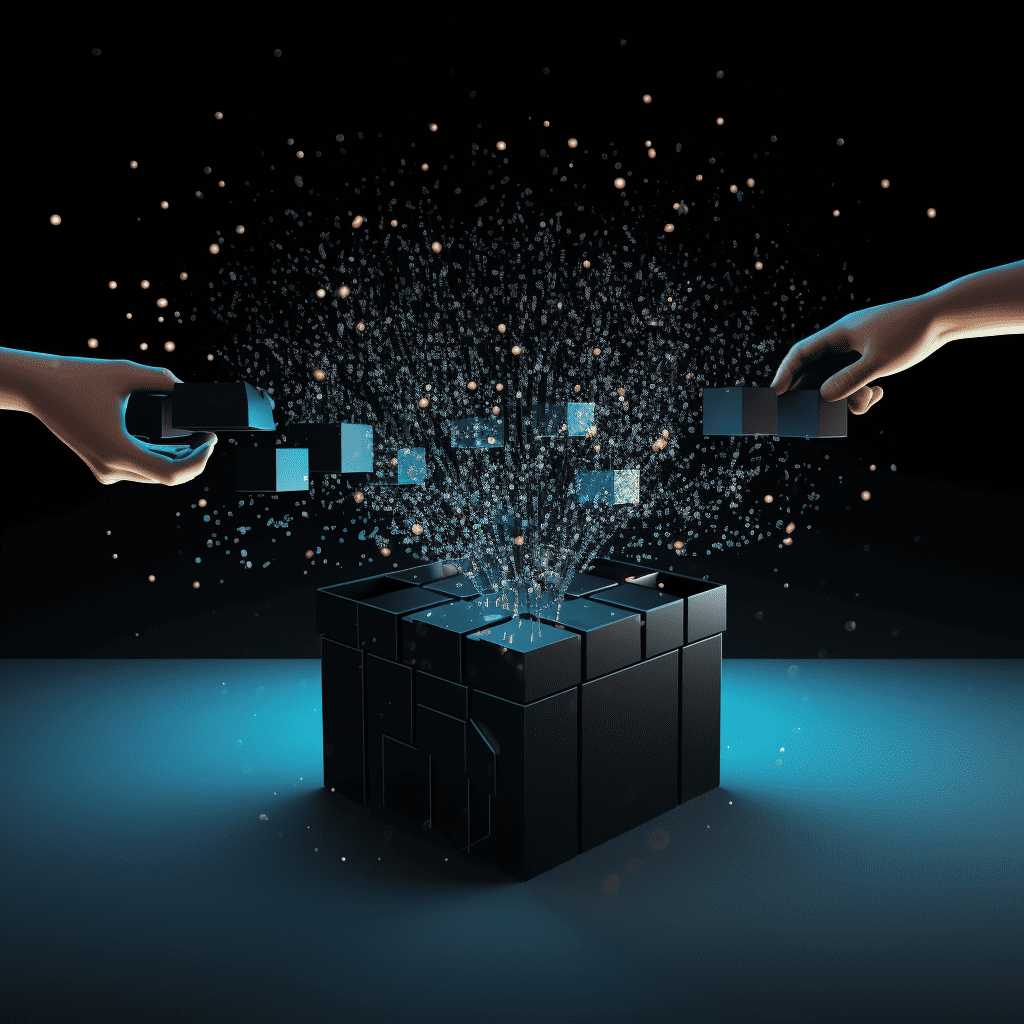
Benefit humanity
As AI advances, its integration into scientific research and everyday applications seems inevitable. Hassabis’s vision for AI is not just about technological progress but about harnessing this progress to solve some of the world’s most pressing challenges. The journey of AI from a specialized tool to a general solution applicable across various domains illustrates the tremendous potential of AI to benefit humanity.
Which goes much further than ‘just’ healthcare. “Imagine any problem in science with a huge combinatorial search base, huge numbers of possibilities, way more than you could search by brute force. Let’s take chemistry, the space of possible compounds. Some people estimate that’s 10 to the power 50 regarding the possible compounds one could create. Intractable to do that by hand. You can build a model of chemistry that understands what’s feasible in chemistry, you could use that to do a search, but you search just a tiny fraction of the possibilities that are of the highest potential value. There are a lot of things in science that fit that. Finding a drug compound that has no side effects, but binds exactly to the thing that you want in it, on your protein or the bacteria, that’s an example. Finding new materials, like a room temperature superconductor, or the ultimate battery design, those are the things I’d love to turn our systems to. All of those things can be re-imagined in a way where these types of tools and methods will be very productive.”