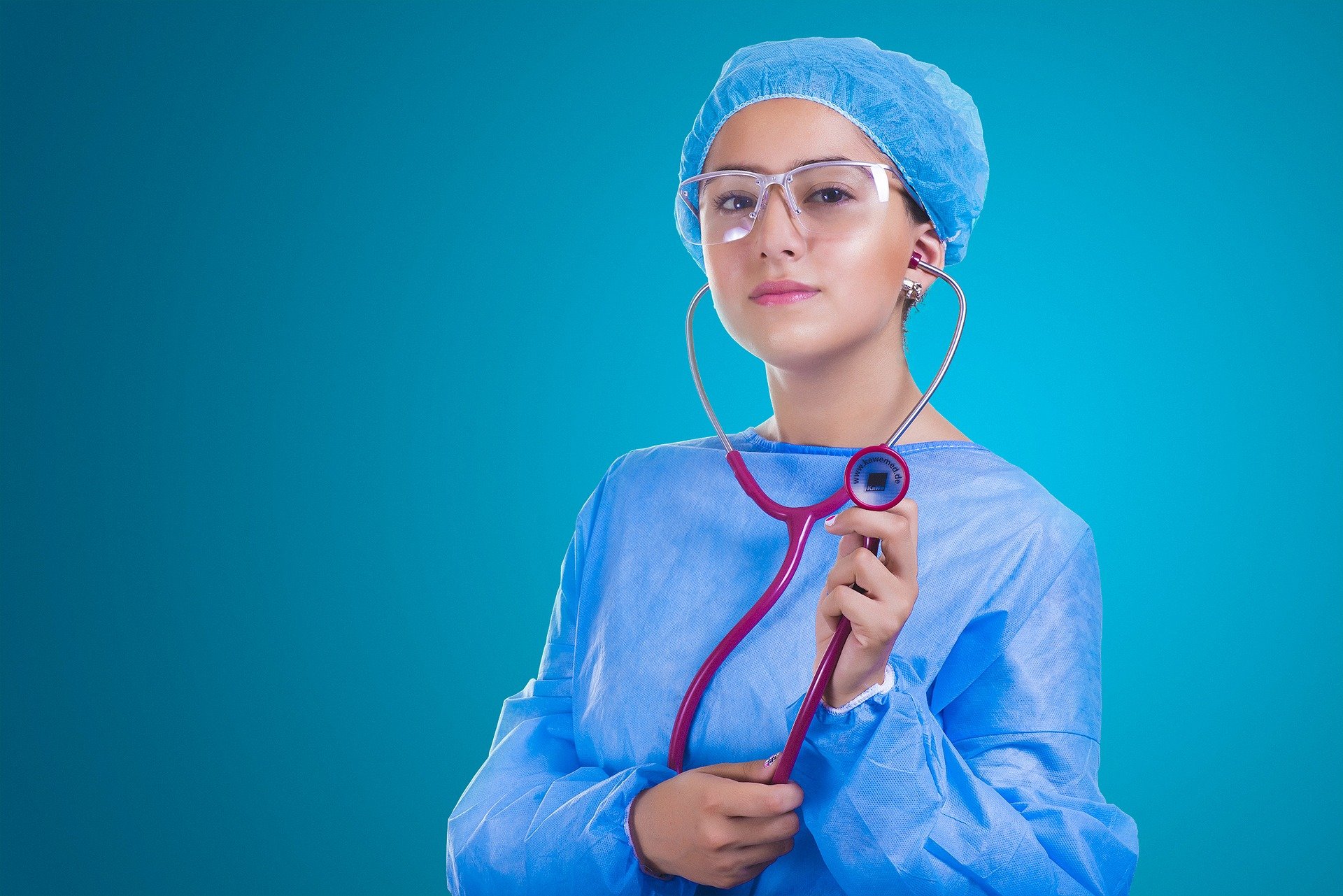
Artificial intelligence is already being used in medicine. If a doctor picks up his smartphone during an examination, he could use an app to seek support for a diagnosis in an imaging procedure. Other applications include automated appointment scheduling, telemedicine and videotelephony and apps that help patients identify their ailments and seek targeted medical help.
“What happens in the field of artificial intelligence in medicine today are pattern recognition techniques, algorithms that can recognize and classify patterns in very high-dimensional complex data. Here even predictive patterns are recognized. The current standard methods have been established for eight to nine years and are based on neural networks,” explains Dr. Horst Hahn, director of the Fraunhofer Institute for Digital Medicine MEVIS in Bremen, Germany, in an online discussion organized by the University of Vienna on April 15, 2021, as part of the Kaiserschild Lectures.
Complementarity of humans and machines
Artificial intelligence is fraught with several problems in the public debate. First, the very name artificial intelligence is very vague, suggesting the idea of an unpredictable power that could one day turn against us. In fact, however, it is mathematicians who develop the algorithms – and they usually know exactly what they are calculating, according to the consensus of the researchers in the discussion. Nor is it by any means the case that artificial intelligence should replace doctors. In research circles, the complementarity of man and machine is assumed. After all, there are already human-in-the-loop systems in which humans support machines in difficult or unknown situations.
The pitfalls of artificial intelligence systems in medicine
“The patterns or correlations found are only as valid as the underlying data, and even in medicine, data is not always collected without bias,” admits data mining expert Professor Claudia Plant of the Institute of Computer Science at the University of Vienna. For example, she says, it is well known that women, as well as certain ethnicities and genetic subgroups, are underrepresented in medical databases. “Even more problematic is that we don’t actually know what to look for,” Plant says. Research on this is still in its infancy, she adds.
Hahn points out that more generally, artificial intelligence could have undesirable effects on society. After all, he says, if machines can make predictions from comprehensive high-dimensional data faster than humans, that can easily lead to humans losing sight of details and handing over decisions to the machine. “Then you fall right into the trap of artificial intelligence systems, which is if, for example, data is not right and the subgroup is not trained well enough, then the answers are not right or have exactly the bias that Ms. Plant is addressing,” Hahn says.
“If machines can make predictions from comprehensive high-dimensional data faster than humans, that easily leads to humans losing sight of details and handing over decisions to the machine.”
Dr. Horst Hahn, Director Fraunhofer Institute for Digital Medicine MEVIS Bremen, Germany
The data protection problem
Artificial intelligence is based on very large amounts of data collected from open data platforms. Researchers are dependent on these sources. At the same time, the risk of patient identifiability is omnipresent. This is especially true when it comes to rare diseases when linking different data sources available online or even if a date is accidentally not deleted. Hahn is researching a project in which data stays where it’s generated and analyzed, and where the analyses are coordinated. It’s a form of federated learning, or multicenter machine learning approaches, that allows algorithms to be trained to keep data private.
He believes this approach is all the more necessary as increasingly complex questions are asked. Does this include the relationship of a particular genetic variant to patterns we see in imaging and the possible disease progression from that? At the same time, the questions are also becoming more sensitive, for example, regarding the likelihood of developing a disease, whereby multiple data sources are combined to find the answer.
Predictable artificial intelligence
Yet another aspect could lead to a knowledge gap in the longer term: Artificial intelligence can make very good decisions, but it cannot always explain itself. This means that causality and correlation cannot be observed separately, as required by the philosophy of science. According to Hahn, researchers around the world are investigating this and there are already various methods that merely need to be sufficiently implemented. For example, it is possible to query similar cases in the database to gain certainty. In addition, it would be possible to evaluate where a neural network is unsafe. “There’s a whole range of approaches there and I think that one day we’ll be talking to these systems in a sense, that we’ll be allowed to ask them, how do you know this, and that they’ll also learn to say, I don’t know,” Hahn says.
Excessive demand
Prof. Jens Meier, who holds the Chair of Anesthesiology and Intensive Care Medicine at the University of Linz, Austria, thinks the call for explainable artificial intelligence may be overblown. “We don’t know the mechanism of medicine in sufficient detail and only know how things work from observations. Causality in medicine is something we like to sell to the outside world, but if we’re honest, more often than not we only have observations.”
He received support from Dr. Barbara Prainsack of the Institute of Political Science at the University of Vienna. She says that experiential knowledge is not always explicitly articulated. But that doesn’t mean decisions are wrong or bad, she said. Says Prainsack, “So are we applying double standards here and saying the machine has to be able to explain everything and humans don’t?”
Shaping interaction
The social responsibility lies in designing the interaction between humans and machines correctly, concludes Prainsack. Machines don’t get tired and can process large amounts of data. But it’s not just a matter of conveying information. Contextual knowledge and communication cannot be expected from machines, she says.
“If the task requires people, then processes should not be automated, even if it might be technically possible.”
Dr. Barbara Prainsack, Department of Political Science, University of Vienna
Plant encourages intensifying artificial intelligence research in medicine with the stated goal of benefiting humans with it. Worldwide, most of the money for artificial intelligence research goes to the military sector, often in countries with questionable democratic systems. Plant comments: “I think we should have the courage to have artificial intelligence research that corresponds to European values not just in medicine, but also in other topics.”
Also of interest: Law in the making: European Commission wants a list of ultra-risky AI