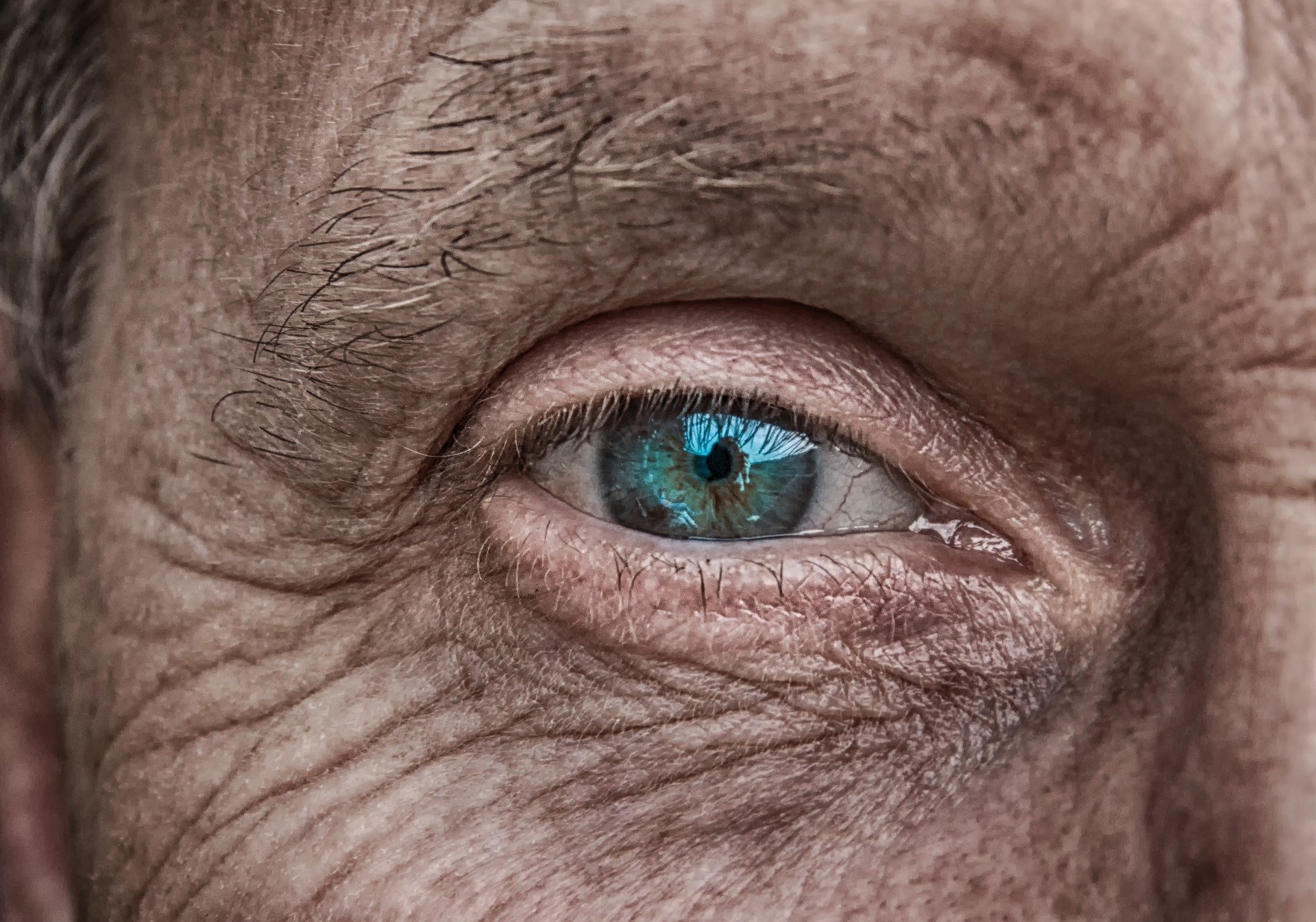
A group of scientists developed an AI model capable of predicting skin cancer risk based on a face photograph. The Erasmus Medical Center (EMC) in Rotterdam researchers’ model outperforms current assessment methods.
The AI algorithm’s power lies in spotting risk factors invisible to the human eye. “As a dermatologist, I pay attention to things like pigment spots, wrinkles, or knowing someone has lived in a sunny country. The algorithm picks up more features than just those classic risk factors for skin cancer,” explains dermatologist Dr. Marlies Wakkee, head of the research.
The research team trained the model with over 2.800 photos. The doctors knew who had developed skin cancer. The algorithm automatically extracts 200 features from the photos, based on which it predicts the risk of skin cancer, either on the face or elsewhere on the body. The AI prediction proved to be more accurate than skin examination, questionnaires, and genetic research. The researchers published their findings in the scientific journal The Lancet’s eClinicalMedicine.
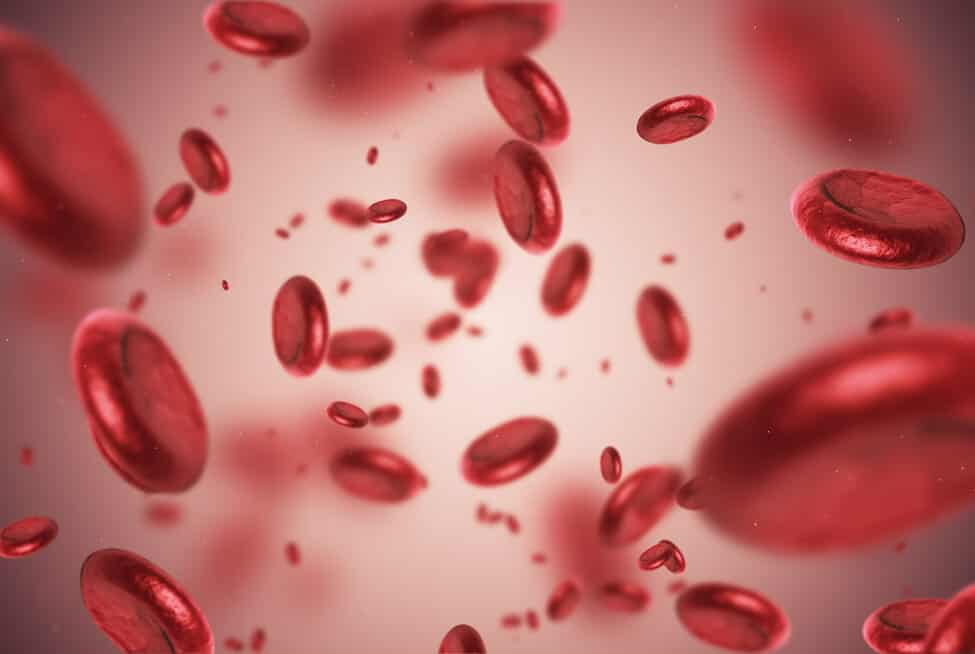
Explainable AI
The researchers used so-called explainable AI methods. This means it’s possible to understand what the algorithm bases its predictions on. This isn’t always the case with AI. Often, it’s a kind of black box where it’s unclear how the model arrives at a particular outcome. “We visualize exactly what the 200 features are that the model bases the prediction on. When we translate them back into what is known about the risk of skin cancer, we see that the algorithm picks up features from the faces we already know are associated with skin cancer. Think of wrinkles, for example,” Wakkee explains. In addition, the model picks up less clear features to humans. “Explainable isn’t the same as interpretable. We can explain what the algorithm does, but what that means in a human way isn’t so obvious,” says AI expert Dr. Gennady Roshchupkin.
Wakkee and Roshchupkin see the AI risk model as improving the prevention and awareness of diseases. The model isn’t available for use in the clinic yet as the focus is on further research. The team plans to test the algorithm on larger datasets with people of different ages and ethnic backgrounds.