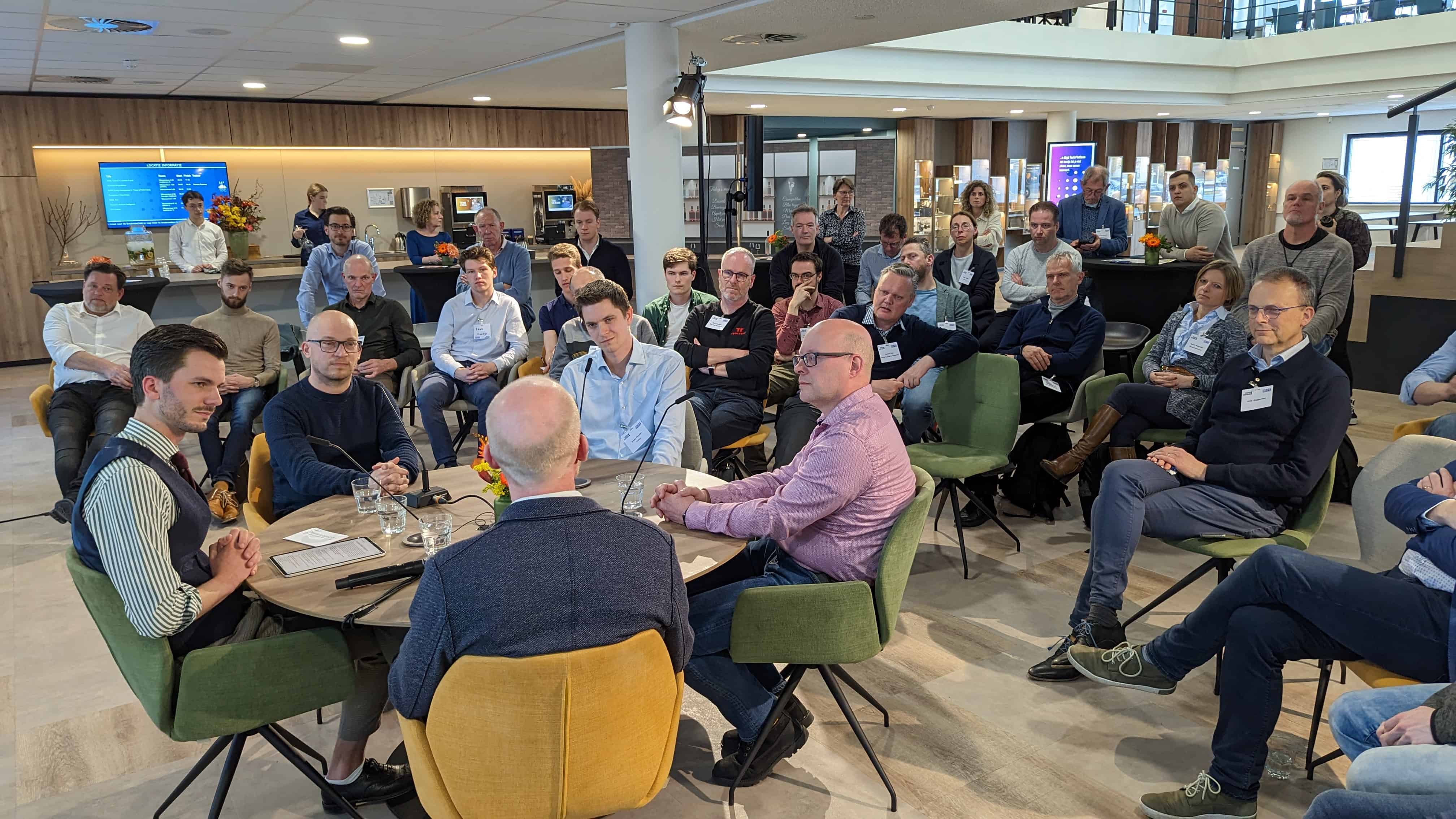
Artificial Intelligence’s role in the manufacturing industry is increasing. By collecting, analyzing, and applying data, companies can work more efficiently, save costs and deliver higher-quality products. Examples include predictive maintenance, quality control, supply-chain optimization, robotics applications, or smarter product design. By using these technologies, companies can improve their competitive position and better respond to changing market demands.
On April 20, the Mikrocentrum Techcafé took an audience from the high-tech manufacturing industry into the world of AI. What can we do with AI in the manufacturing company? Where should we start, or have we already started? Maarten Roos engaged in a conversation with 4 experts:
Albert van Breemen – CEO/CTO at VBTI
Hugo Ploegmakers – Director of Product at AM-Flow
Frans Fonville – Lecturer of the Master Digital Technology Engineering, Fontys University of Applied Sciences
Sjoerd van Agtmael – FMI ImProvia
It seems like everyone is working on artificial intelligence these days. Whereas it was first reserved for web-tech companies such as Google and Meta, this technology has now penetrated the manufacturing world as well. “Digital technology is often driven by big tech, after which we as a society respond to it,” says Frans Fonville. “We as a society look at how we can incorporate it and benefit from it. But now we are to the point where we start using that digital technology ourselves. That’s a different direction. No more technology push but the other way around; we will fill in and embed it ourselves. The starting point here is often a target group that you can help with digital technology.”
0.2 seconds
But how does that work for the manufacturing industry? At AM-Flow, a lot of experience has been built up with it. Hugo Ploegmakers: “For years, we have been looking for ways to control the quality of the many thousands of products made with 3D printing. For a very long time, this was done manually, but of course, that is a huge job. With AM-Flow, we managed to create a model that can check every object in 0.2 seconds based on vision and machine learning. A human would need roughly half a minute to do that so that business case is clear.” In doing so, AM-Flow uses its own data and the customer’s. “And if we don’t have enough data, we try to generate it, for example, by deploying additional cameras.”
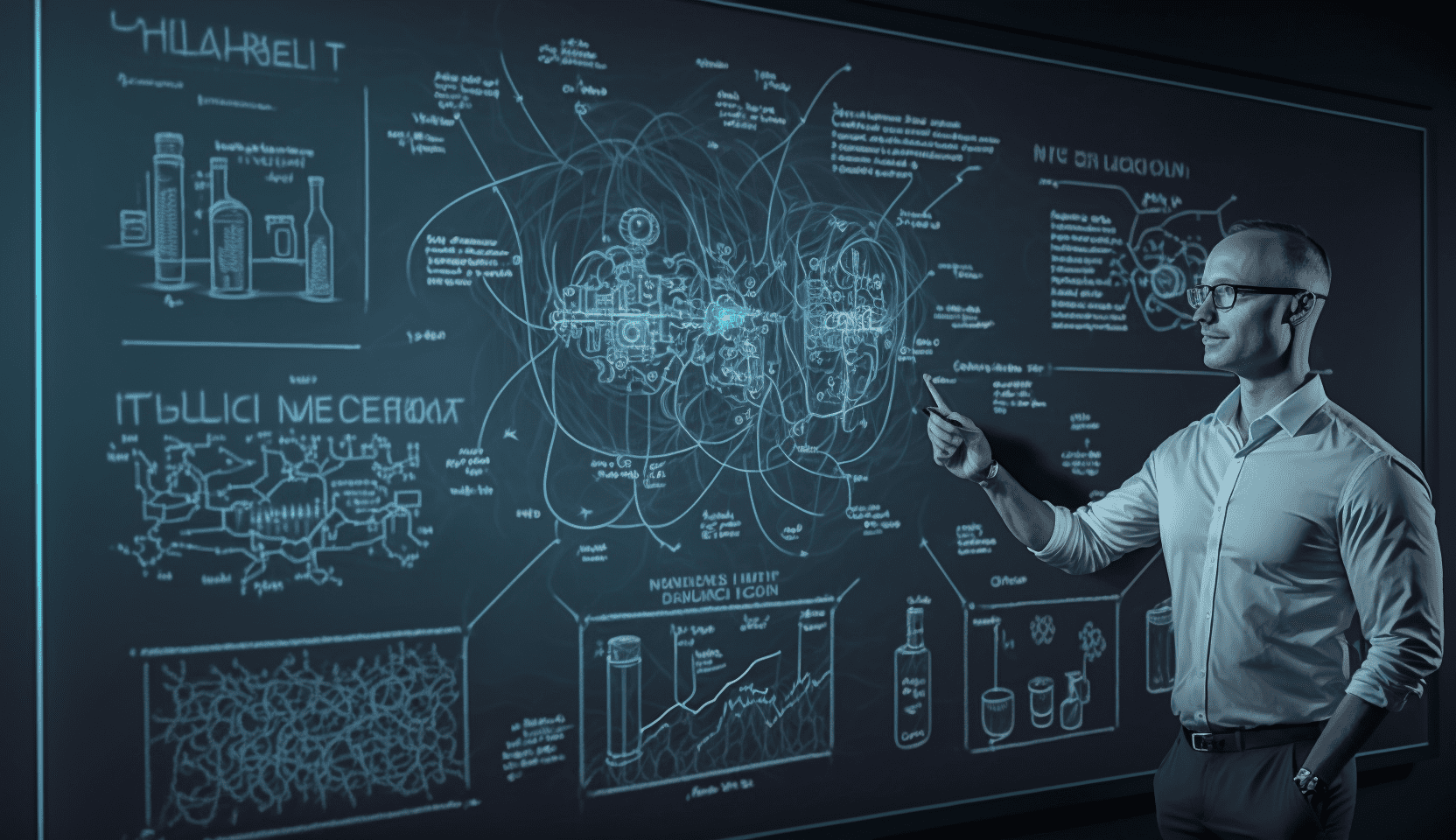
Yet the quality of the result certainly does not depend solely on the number of cameras or sensors, Fonville says. “We sometimes think that by first collecting data at random and then going at it like some kind of Sherlock Holmes, we automatically arrive at the best result, but that is not the case. Not only does such a method often cost a lot of time and money, but it is simply more effective to first look at which sensors are elementary to maintaining your system. You want to know which sensors add value and what the connection is between what you are interested in and what you are measuring. So don’t just start hanging everything full of sensors, but start small and think smart.” Sjoerd van Agtmaal couldn’t agree more. “Good old 6Sigma is not so bad at this point. You can always expand it later, but it’s precisely by starting small that you get the best results.”
Branching
Added to that is learning to understand where the strengths and weaknesses of AI lie and what that means for the deployment in your company, Albert van Breemen adds. “So define your goals. AI is nice, but only if you know how you want to deploy it. For example, if you’re using it to guide a certain vacancy process, make sure you don’t just do that with data from old selection processes. Because then the algorithm is going to work as it used to, while you’re looking for someone who fits the needs that are yet to come.” For a manufacturing company, this is sometimes difficult to understand, Van Breemen said. “AI is a field with all kinds of subsections. We now employ 20 people, all with their own specific expertise. It’s not like you can be an AI expert with some Python and Google experience. The performance requirements of the high-tech industry require more than a little basic knowledge. You can then get to 90% at most, while 99.99999% is needed.”
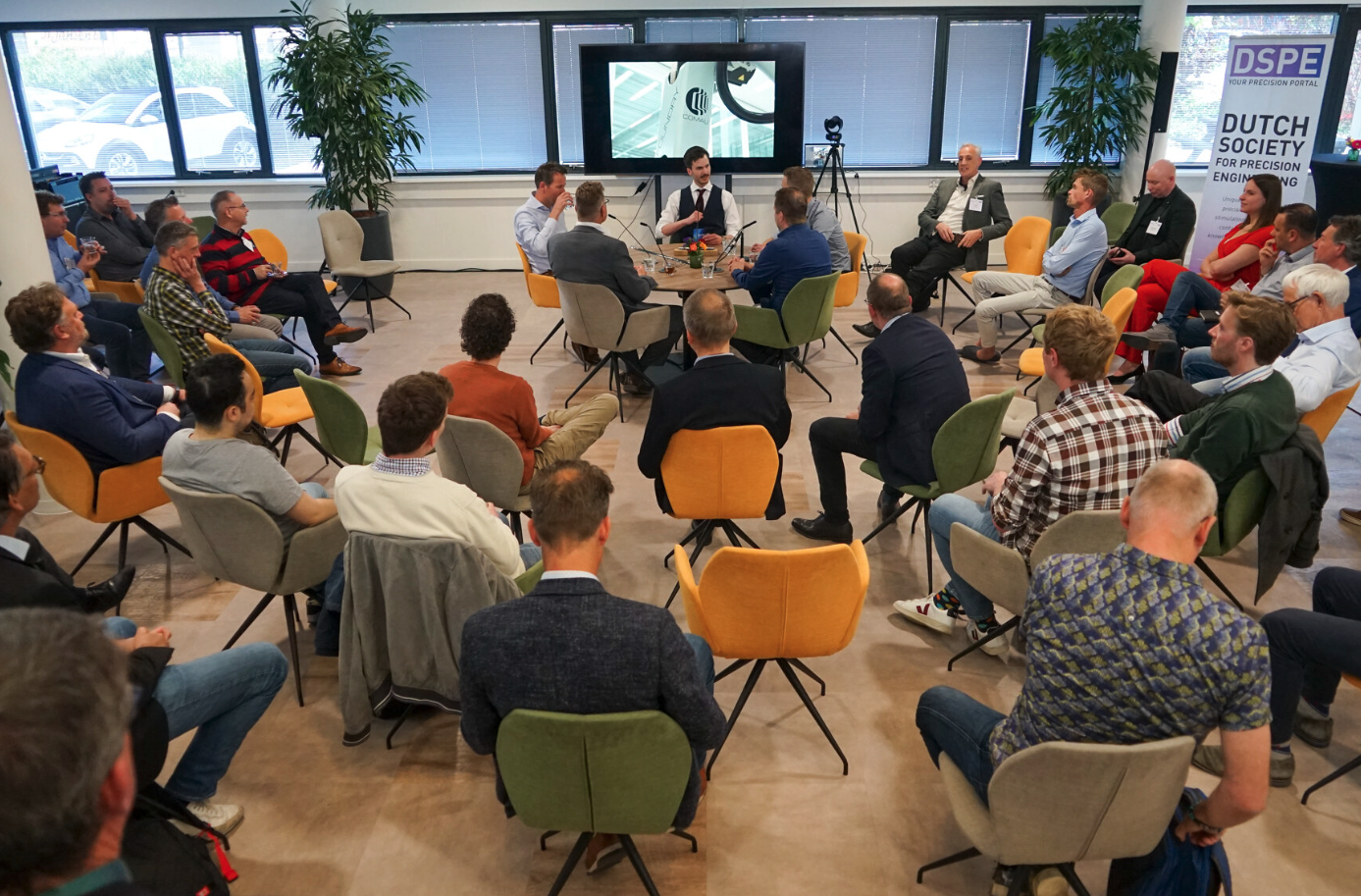
With VBTI, Van Breemen is now active in several industries. Horticulture is one of them: thanks to algorithms based on image recognition, robots can pick exactly the right fruit or cut the right leaves. Van Breemen: “In a greenhouse, every detail is different, the variation is extreme. A traditional algorithm cannot handle that, but AI can, thanks partly to its self-learning aspects. With this, we remove a bottleneck that had been there for years. And we can finally properly automate jobs for which there is little demand – such as that of leaf cutter.”
Growth market
Variation is thus an important condition for the useful deployment of AI in the manufacturing industry. In most parts of the manufacturing industry, such an approach is not yet an issue because of the uniformity and predictability of the process, says Van Breemen. “But if there is more variation there too, as with the tens of thousands of different products AM-Flow wants to monitor, then AI does come into the picture.” Van Agtmaal agrees: “At the industrial level, we must continue to ask ourselves whether the machine can become self-learning, whether an AI can control the machine. There still need to be guidelines and safeguards around quality and safety.”
Even though AI may be causing a transformation of many jobs and perhaps even making some redundant, that does not yet mean it poses a structural threat to employment, the panel notes. For that, the manufacturing industry is still too much a growth market, with more problems around finding sufficient talent than disappearing jobs. But that does not alter the fact that much can change. Ploegmakers: “We really do have to make sure that the people who are less digitally adept are not left standing aside.”
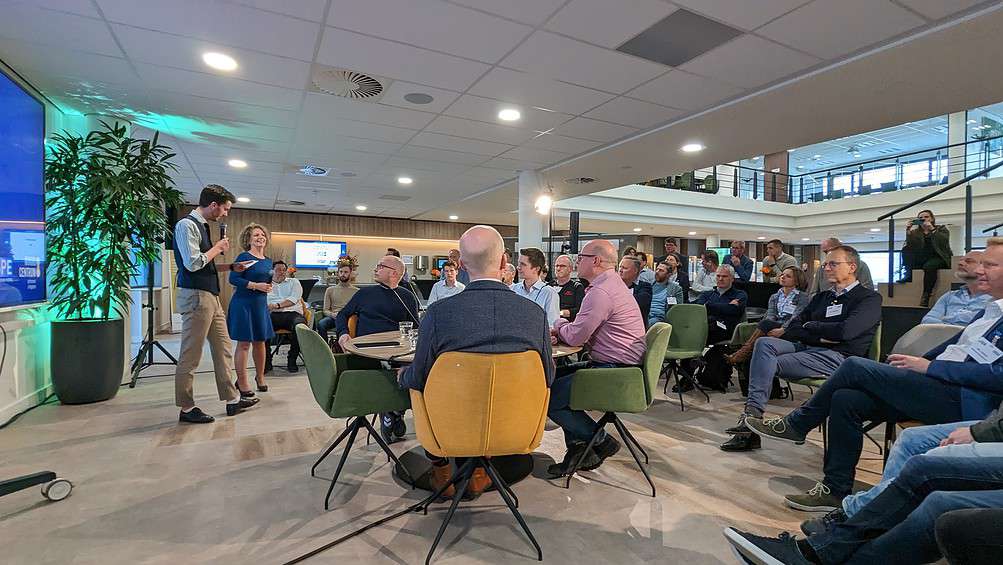