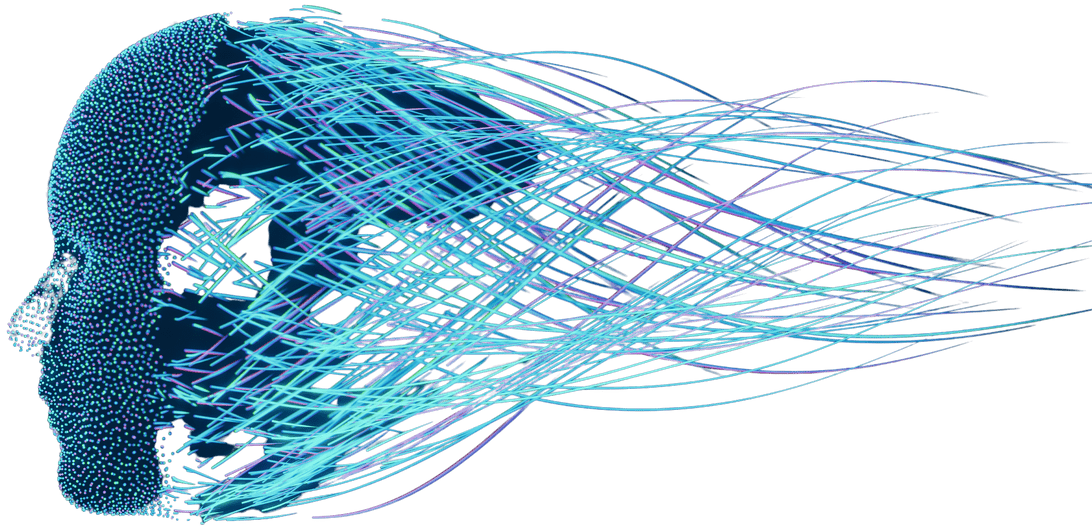
About Sapiema
- Founders: Alexandru-Mihai Glontaru
- Founded in: 2022
- Employees: 2
- Money raised: 75,000 Euros in CLA
- Ultimate goal: AI-solutions provider in Europe, specially in the Fintech sector
With recent advancements in the AI field, more and more companies are developing products based on this technology. But implementing this successfully is not child’s play, as it requires knowledge of data science and Machine Learning. There is a noticeable gap of experts in the industry, and thus companies have been struggling to utilize AI to its full potential.
In an attempt to help companies, especially SMEs, to utilize this space and build AI solutions effortlessly, Sapiema has built an online assistant named Adam. The assistant acts as a virtual expert providing specialized solutions for one’s Machine Learning need. In this installment of the Start-up of the day series, Alexandru-Mihai Glontaru tells us more about his solution-based assistant.
What is the problem that you are solving? How are you addressing the issue?
“There is a huge lack of data scientists and Machine Learning (ML) specialists in the market. Even if most people in the industry expect the problem to get gradually solved by training more people, in reality, it becomes worse. With people in academia moving towards the industry for higher pay, there is a subsequent gap in the teaching ecosystem as well.
So we are automating the development of AI-based projects virtually by transforming anyone into a machine learning specialist, no matter what their technical skills are. We transform them into data scientists by giving them the proper tools, namely an assistant who does it for them.”
How does this virtual assistant function?
“It is a virtual machine learning engineer, as we call it, which has a conversational interface and offers a similar experience as the user would have with a remote engineer sitting somewhere in the world. They open the chat and have a conversation with this assistant, a virtual engineer. They describe the problem at hand from the business requirements perspective, and then the virtual engineer named Adam starts guiding the user through an entire process of collecting relevant information. The conversations are designed in such a way that they are accessible to anyone, no matter what their level of knowledge about technical things. Usually, what we expect from our users is to have knowledge of what problem the users want Adam to address. And during this conversation, Adam just tries to fill in a configuration file based on which it then generates the entire solution, trains it automatically, deploys it on the customer’s infrastructure, and just outputs back web API that the customer can integrate with their product.”
With so much data transmission, what are the possibilities of data privacy issues?
“The entire training, data transformation, and data cleaning part, as well as the deployment of the models, happen on the customers’ infrastructure. So we never see the data. There is no data sharing involved. Even if Adam knows where the data source is, it never accesses the actual data.”
How is innovation different from those already existing?
“We are in a space that has a lot of visibility, and we have very big companies as competitors. What we do differently, first of all, is a change of perspective. If you look at the current solutions on the market, almost all of them are focused on making data scientists more productive. In reality, for a lot of companies, especially SMEs, it’s almost impossible to get their hands on one data scientist. What we are doing is to give to empower non-technical people to be able to manage such projects by themselves. There are data engineers and back-end engineers who would like to work on machine learning projects, but they don’t have the time or budget to learn about machine learning. So with such a tool, they can start working immediately without a steep learning curve.
There are ML cloud providers who are focused on developing large models that are good at a broader scale. For example, if you look at Google Cloud they have the text model, the image model, and the video model, but the reality shows that these kinds of models cannot perform effectively because they are too general. So our approach is to train smaller models on specific problems, on specific data sets, such that we afford to become a little bit less general but still be right most of the time in our predictions or classifications.
And most importantly, we are the only solutions provider on the market who offers a fully guided experience. None of the other providers have such a fully assisted experience, which makes it very easy to adopt our solution because there is no learning period. You can just chat as you do with your colleague, and it will fulfill the work.”
What is the biggest obstacle you have faced till now?
“Building trust with our customers has been our biggest issue because what we are offering sounds a little bit Sci-Fi. And as we are still developing the first version of the product, even if we show them a demo that can already train a model and deploy it, the customers feel the end goal is technically unachievable. You need to have a lot of context to understand it. For people, it’s difficult to understand how we get from point A to point B. So their immediate reaction is to say okay, maybe you don’t ever get to point B if you cannot make us understand. However, we know it’s possible.
We are already very close to launching the first version of the product. We solved the technical issues that arose. At this point, we need to build a better communication strategy to make our potential customers believe in the functioning of our product.”
What does the future look like for Sapiema?
“First of all, we need to close the pre-seed round, which is going very slowly because of global economic turbulence. We need to employ more specialists in different areas, which makes a successful pre-seed round all the more important. As our main goal for the next year, we want to become the AI solutions provider in the fintech market in Europe. Though we also want to work on health-tech and legal tech, fintech is a good use case for our technology. The big financial institutions have teams of data scientists who innovate at a fast pace, so the smaller companies need to keep up with the innovation pace, and this is where we step in.”
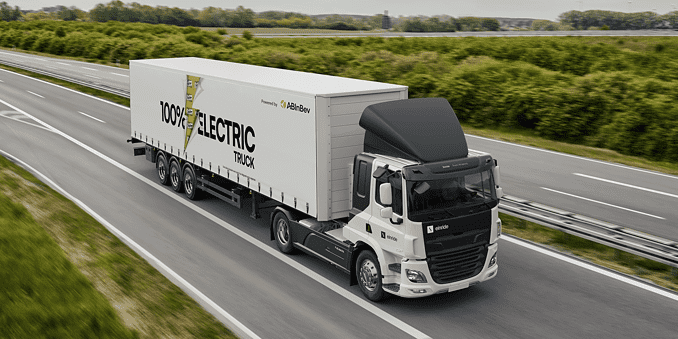