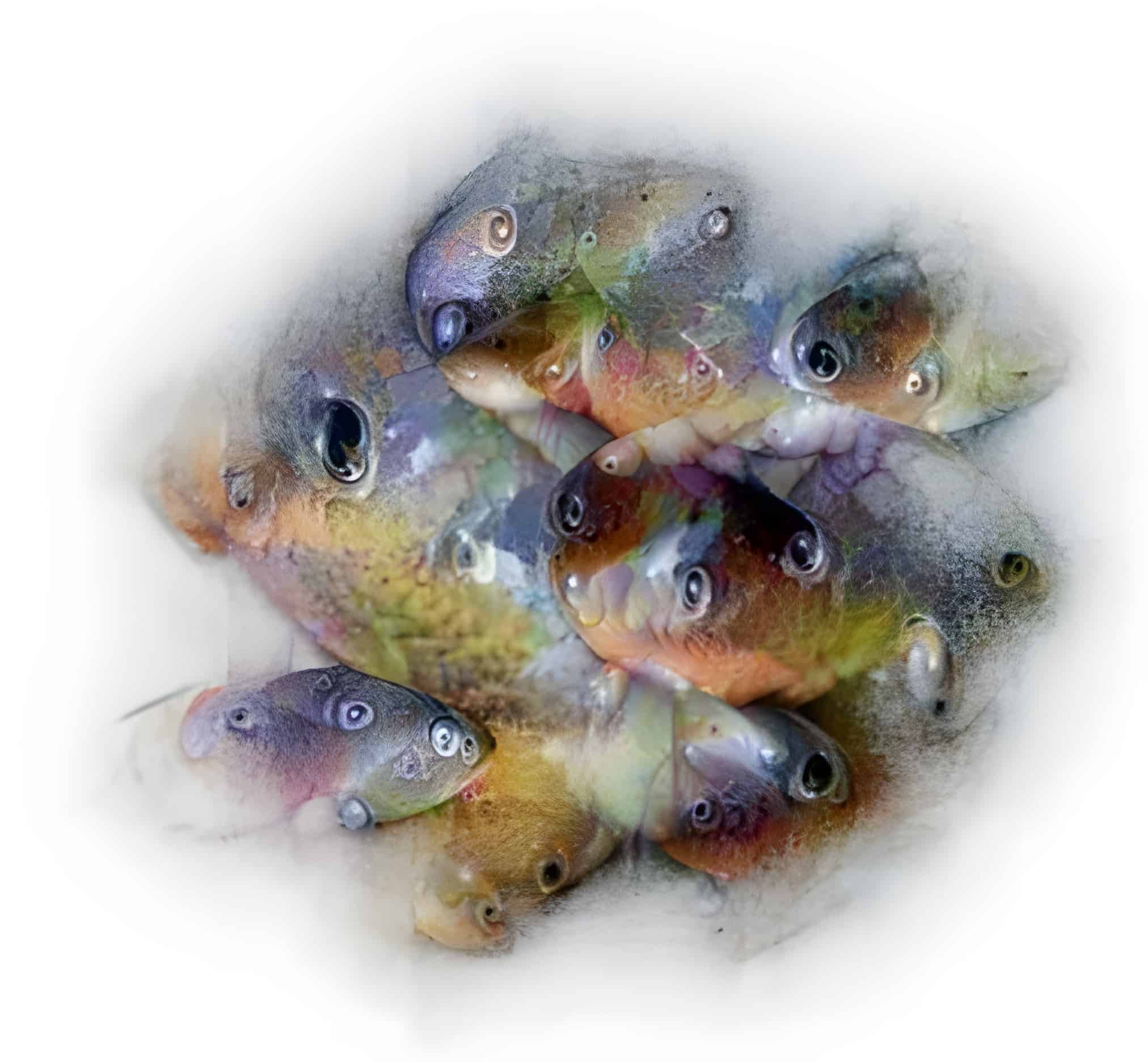
Scientists at Brown University’s Carney Institute for Brain Science have created CRAFT, a tool to elucidate how artificial intelligence (AI) systems perceive images, and why they occasionally fumble classifications. CRAFT deciphers visual representations learned by neural networks, offering insights into the concepts AI systems use for object recognition. The tool also exposes biases in data sets, which AI systems might inadvertently adopt. Unlike other methods, CRAFT identifies and ranks concepts based on importance, which aids in understanding the root cause of AI errors.
- CRAFT utilizes a recursive strategy, concept importance estimation using Sobol indices, and implicit differentiation to decode visual representations learned by neural networks.
- CRAFT’s effectiveness was evident in psychophysics experiments, where experts and non-experts found its generated sub-concepts to be coherent and meaningful.
- Future prospects include tackling scientific challenges in areas like cancer diagnostics, fossil recognition, and space exploration, leveraging the understanding gained from CRAFT.
Mechanics of the CRAFT method
The CRAFT (Concept Recursive Activation FacTorization for Explainability) tool incorporates three novel elements in its concept extraction process. It utilises a recursive strategy to detect and decompose concepts, a unique technique for concept importance estimation using Sobol indices, and the application of implicit differentiation to create concept attribution maps.
These innovative approaches enable CRAFT to provide a more accurate and comprehensive explanation of how deep learning models perceive images. The tool not only identifies ‘what’ the model sees in images, but also ‘where’ it sees these elements. The blend of these features makes CRAFT a potent instrument in the quest to understand AI’s visual processing mechanisms.
CRAFT outperforming other explanation methods
In human-centred utility benchmarks, CRAFT proved its efficacy by significantly improving results in two out of three test scenarios. It even surpassed other attribution methods and ACE, another concept-based explanation method. By providing better estimates of concept importance compared to TCAV scores, CRAFT allows for a more nuanced understanding of AI’s decision-making process.
The tool’s effectiveness was also evident in psychophysics experiments. Here, both experts and non-experts found the sub-concepts generated by CRAFT’s recursive strategy to be more coherent and meaningful. This feature makes CRAFT an invaluable resource in translating the complex language of AI into terms more easily understood by humans.
Implications and applications of CRAFT
Understanding the intricacies of AI’s visual strategies is essential for enhancing the performance and accuracy of vision-based tools like facial recognition. It also bolsters AI’s defences against adversarial attacks, in which tiny alterations to images can trick the system into making incorrect decisions. By illuminating the strategies employed by AI, CRAFT helps to make these systems more trustworthy and resilient.
Moreover, the insights gleaned from CRAFT can contribute to the development of more human-like and intelligent AI systems. For instance, OpenAI’s research on multimodal AI, particularly the CLIP model, has demonstrated the transformative potential of AI systems that can understand and generate content across multiple modalities. By integrating the key mechanisms of general intelligence and visual intelligence identified by CRAFT, AI systems can become more sophisticated and effective.
Future prospects of CRAFT
Looking ahead, the next stages of this research involve leveraging the understanding of AI and human vision to tackle significant scientific challenges. These include areas such as cancer diagnostics, fossil recognition, and space exploration. By illuminating the complex workings of AI, CRAFT is poised to make significant contributions in these fields and beyond.
Indeed, the journey to understand AI’s visual strategies is only just beginning. But with tools like CRAFT, the veil cloaking the inner mechanisms of artificial intelligence is gradually lifting, revealing a vista of untapped potential and promising opportunities.