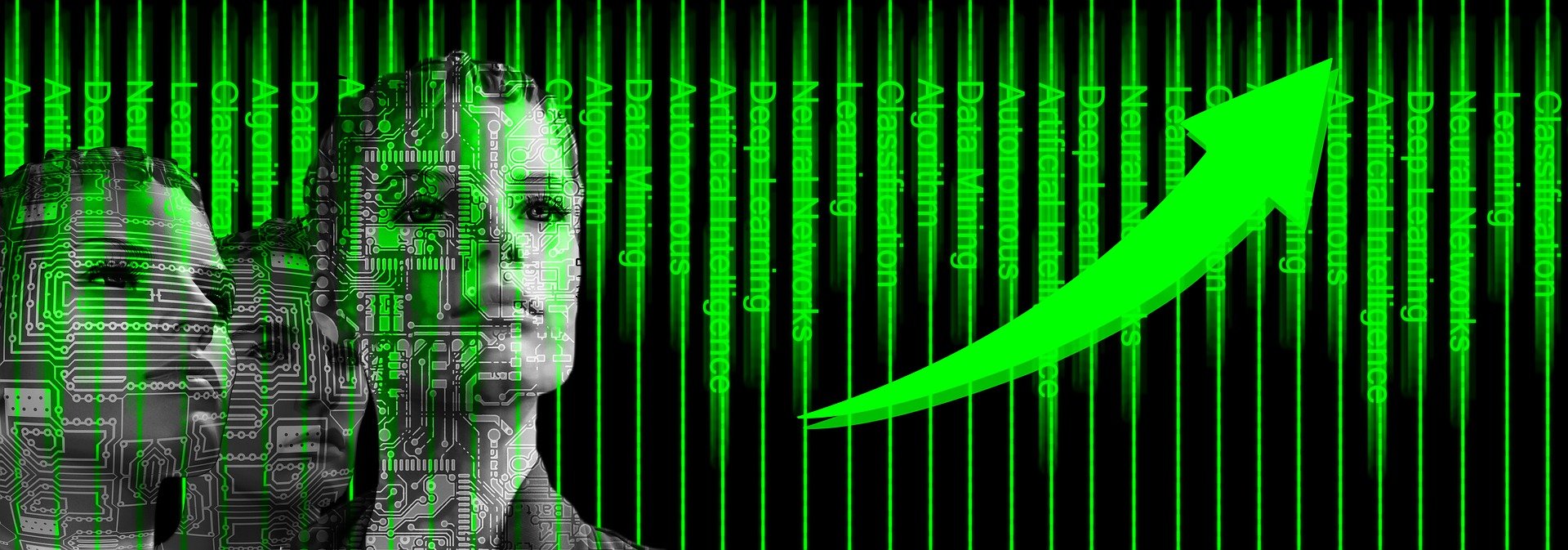
The professor and the politician sat at the table of a lunchroom in The Hague with cups of fresh mint tea. The politician had invited the professor to talk about the transparency of algorithms. He wanted to set strict rules for the use of algorithms by the government, with emphasis on the word “strict,” he added.
The politician said, “I want a watchdog who will check all the government algorithms,” words which he clearly found unsavory. The professor noticed that the politician had a preference for the words “rules” and “watchdog”, and for the expression “with an emphasis on…”.
The usefulness of a watchdog
By the time they had finished their first cup of tea, they had found that there are roughly two types of algorithms: simple and complex. Simple algorithms, they thought, translate rules into a kind of decision tree. On a napkin the politician drew blocks and lines to represent this and as an example she cited the application for a rent allowance. She noted that there are already thousands of simple algorithms in use by the government.
The professor suggested that such algorithms could be made transparent relatively easily, but that this transparency would actually bring you back to the regulations on which the algorithm is based. Sipping his tea, he added: “So you could rightfully ask what the use of an algorithm watchdog would be in this case.”
At this point, the conversation stopped for a moment, but then they decided they agreed on this after all.
“B-uu-uu-t,” said the politician, looking ominous again, “then there are the complex algorithms. Neural networks and all that.'”
The professor looked thoughtfully out the window since that seemed like the right thing to do, then replied that neural networks are as transparent as the human brain. If you could make neural networks transparent, you wouldn’t be able to derive anything from them.
The politician nodded slowly. She knew that, too.
Training the network
You can train such a network, you can test the outcome and you can also make it work better, but transparency, or the use of an algorithm watchdog, wouldn’t add any value here either, the professor concluded.
Once again, the conversation came to a standstill.
The politician had spoken and the professor couldn’t disagree with her. “That’s precisely why I want a ban on the use of far-reaching algorithms by the government,” added the politician, “emphasis on the word ban.”
“The effect would then be counterproductive,” the professor said, “by prohibiting the use of algorithms by the government, you create undesirable American conditions in which commercial parties develop ever-smarter algorithms, become more powerful as a result, and in which the democratically elected government becomes marginalized.
The professor felt that the last part of his sentence had turned out to be softer than he would have liked. He considered repeating it, but instead asked “Why do you always use the word ‘watchdog’?”
“Because a watchdog conveys decisiveness,” the politician replied. “We want to make the public feel safe with the government, and a watchdog is a good representation of that.”
Curious bees
The professor was starting to feel miserable. The government as a strict watchdog? The image reminded him of countries like China. Or America.
“I don’t like that metaphor,” he said, “it has such an indiscriminate character. It’s powerful, but also a bit stupid and simplistic.
“Then why don’t you come up with a better analogy!” the politician challenged him cheerfully.
The professor was reminded of an article he had recently read and replied: “I think the image of a bee population would fit better.” It was a somewhat frivolous answer, but in a bee colony, curious bees are sent out to look for opportunities that are of value to the entire colony.
The politician laughed a lame laugh.
“Nice image, professor, but an algorithm bee wouldn’t work in the political arena!”
The professor suspected that the politician had a good point there.
They had one final cup of tea together and then once again went their separate ways.
bout this column:
In a weekly column, written alternately by Bert Overlack, Mary Fiers, Peter de Kock, Eveline van Zeeland, Lucien Engelen, Tessie Hartjes, Jan Wouters, Katleen Gabriels and Auke Hoekstra, Innovation Origins tries to figure out what the future will look like. These columnists, occasionally joined by guest bloggers, are all working in their own way on solutions to the problems of our time. So that tomorrow is good. Here are all the previous articles.