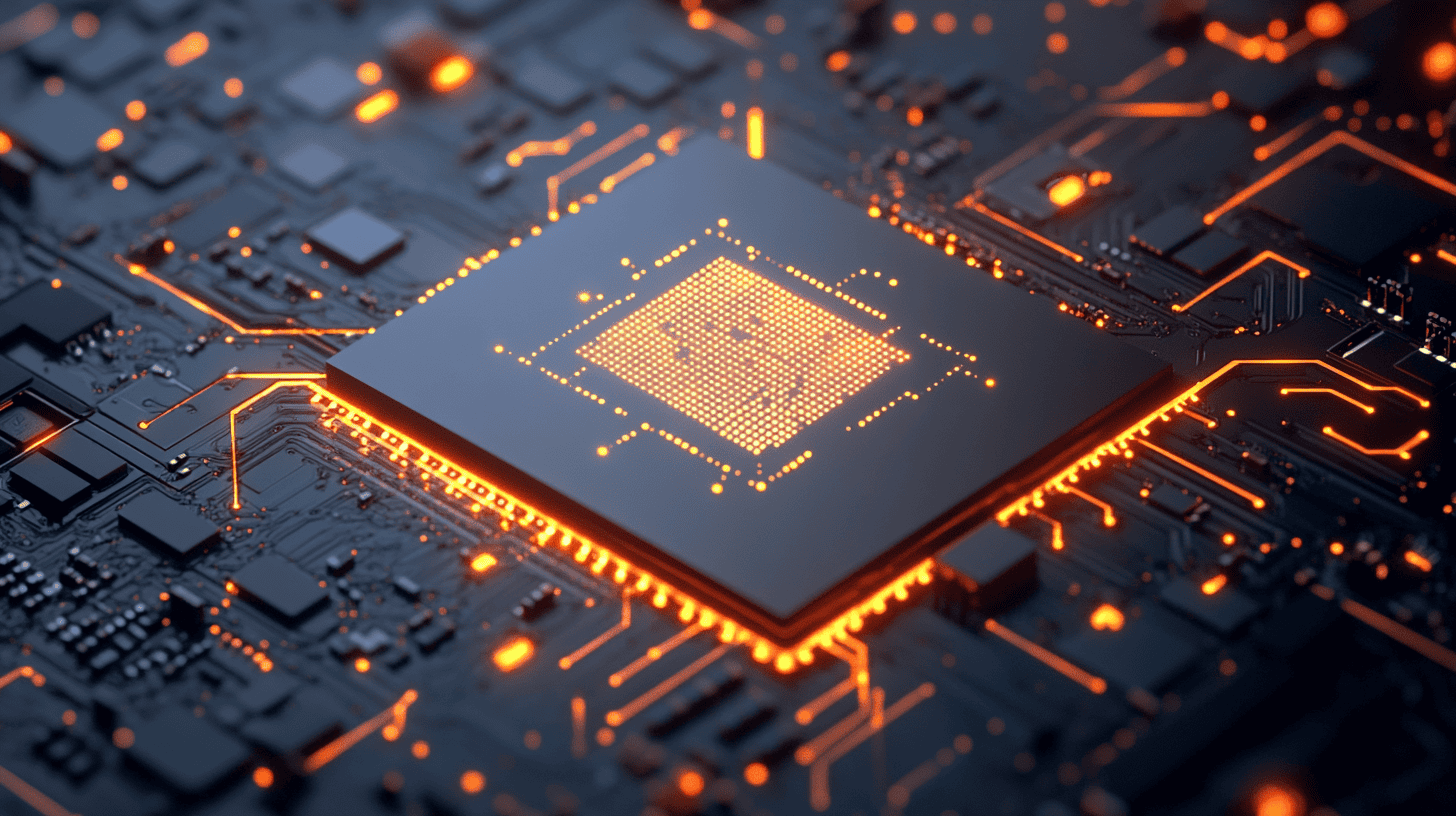
University of Minnesota engineers developed a hardware device that could reduce energy consumption for artificial intelligence applications by a factor of at least 1,000. The technology, called computational random-access memory (CRAM), processes data entirely within memory, eliminating energy-intensive data transfers between logic and memory modules.
Why this is important:
New AI applications are being introduced daily. Despite the many advantages AI brings in optimizing processes, it also comes with a major caveat: the high energy inputs needed to run the hardware. The University of Minnesota endeavor can pave the way toward a more environmentally-friendly AI.
The International Energy Agency (IEA) has forecasted a doubling global AI energy consumption from 460 terawatt-hours (TWh) in 2022 to 1,000 TWh in 2026. This surge underscores the urgency for more energy-efficient solutions. CRAM technology could potentially mitigate this looming energy crisis by offering energy savings up to 2,500 times compared to traditional methods.
The University of Minnesota’s breakthrough addresses a critical challenge in AI computing: the high energy cost associated with data transfers between logic and memory. Traditional computing architectures, like the von Neumann model, separate these units, leading to inefficiencies. CRAM integrates computation directly into memory, dramatically reducing energy consumption. The chip, based on magnetic tunnel junctions, offers flexible computation capabilities and has shown promising accuracy in experimental demonstrations.
Collaborative effort
This innovation is the result of over 20 years of research led by an interdisciplinary team at the University of Minnesota. The team includes experts from physics, materials science and engineering, computer science, and electrical engineering. Their collaborative efforts have culminated in the development of CRAM, a technology that builds on patented research into Magnetic Tunnel Junctions (MTJs).
MTJs, a type of spintronic device, leverage the spin of electrons to store data. This approach is more energy-efficient than traditional transistor-based chips, which rely on electrical charge. The CRAM architecture utilizes these MTJs to perform computation within memory cells, eliminating the need for energy-intensive data transfers.
From concept to reality
Jian-Ping Wang, a Distinguished McKnight Professor and one of the senior authors of the paper, recalls that the initial concept of using memory cells for computing was met with skepticism. ‘Twenty years ago, our idea was considered crazy,’ Wang said. However, the persistence of Wang and his team has paid off, demonstrating that CRAM technology is not only feasible but also ready for integration into existing systems.
The team’s research has been published in npj Unconventional Computing, with multiple patents protecting the underlying technology. Their experimental results show that CRAM-based machine learning inference accelerators can achieve energy improvements on the order of 1,000 times. In some cases, energy savings reached 2,500 and 1,700 times compared to traditional methods.
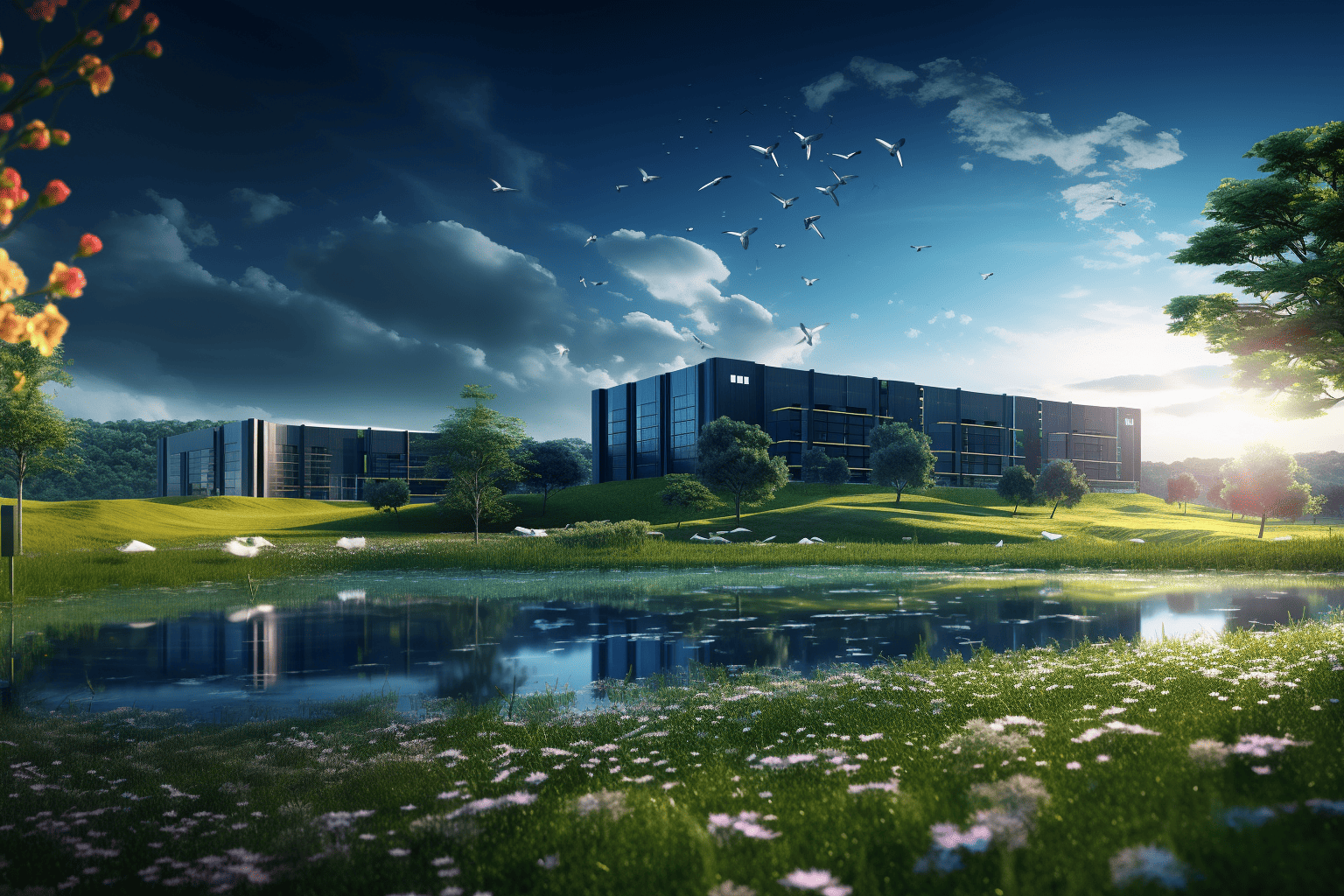
A versatile solution
CRAM’s flexibility is one of its key strengths. ‘Computation can be performed in any location in the memory array,’ said Ulya Karpuzcu, an Associate Professor and co-author of the research. This adaptability makes CRAM suitable for a diverse set of AI algorithms, enhancing its potential applications.
The technology’s versatility extends to its potential use in various AI-driven tasks, such as neural networks, image processing, and edge computing. By addressing the bottleneck of data transfer between logic and memory, CRAM could significantly boost the efficiency and performance of these applications.
Industry collaboration and future prospects
The University of Minnesota team is now collaborating with semiconductor industry leaders to scale up demonstrations and produce the necessary hardware. This partnership aims to bring CRAM technology to market, providing a viable solution for energy-efficient AI computing.
Support for this research came from several prestigious organizations, including the U.S. Defense Advanced Research Projects Agency (DARPA), the National Institute of Standards and Technology (NIST), the National Science Foundation (NSF), and Cisco Inc. These collaborations highlight the broad interest and potential impact of CRAM technology on future computing paradigms.
As AI continues to evolve and its applications expand, the demand for more efficient computing solutions becomes increasingly critical. The University of Minnesota’s development of CRAM represents a significant advancement towards meeting this demand. By drastically reducing energy consumption, CRAM not only addresses the immediate energy concerns but also paves the way for more sustainable and advanced AI technologies.