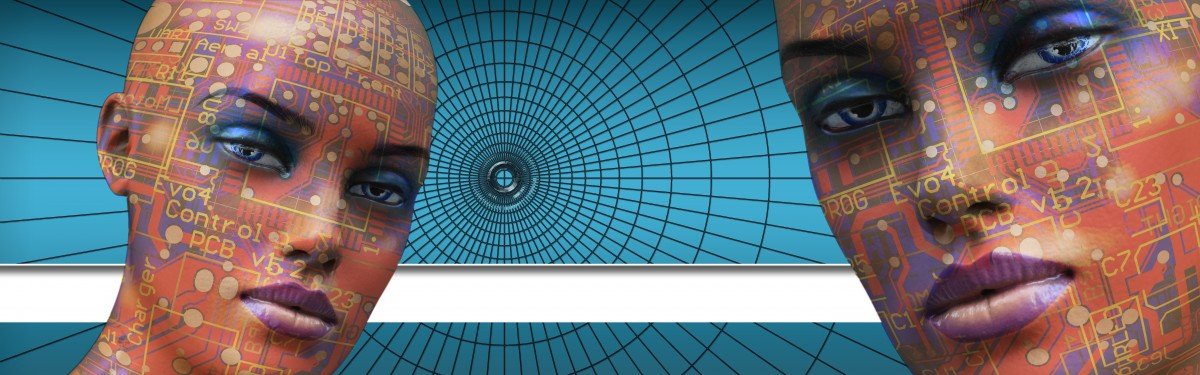
Actually, artificial intelligence’s (AI) purpose is to help people to cope with complex tasks. AI systems can quickly analyse and interpret the large amounts of data generated by modern big data and sensor technologies. They control vehicles or complex production processes.
When artificial intelligence fails

But artificial intelligence makes mistakes or lets itself be duped by childish tricks. In the USA, for example, misinterpretation of sensor data led to fatal accidents with autonomous cars. Chinese teenagers who want to deceive AI-supported surveillance systems in shopping malls or inner cities wear carnival masks. Then, the surveillance AI ignores them as a sensor error. Canadian researchers had AI identify objects in a living room. When they showed the image of an elephant, the AI ceased to function. It became blind to objects it had previously identified correctly. Scientists at the University of Frankfurt are now investigating how AI systems can be made more reliable and, above all, safer. The team around computer scientist Prof. Visvanathan Ramesh is particularly interested in the criteria according to which the reliability of AI systems can be assessed.
How artificial intelligence becomes more reliable
“Absolute security is impossible,” says Professor Ramesh. “In the past, the security of complex systems was proven by formal model-based design processes according to strict security standards in the development and by extensive system tests. That’s about to change. Data-driven machine learning techniques are widely used in AI development. The AIs based on them deliver unexpected and unpredictable results – or they fail when they see an elephant where none was before and which they cannot find in their database.
From Ramesh’s point of view, security comes from thorough and extensive simulation and modelling of application scenarios. The problem, Ramesh continues, is to anticipate as many changes as possible and translate them into simulations. For example, if you want to send an AI-controlled spacecraft to Mars, you have to anticipate as many scenarios as possible and compare them with real data. Simulations are then created from the tested scenarios. These are then used to teach the AI how to react to the respective situation.
Model for artificial intelligence: the human brain
Ramesh’s approach is to combine knowledge from computer science, mathematics and statistics with knowledge from fields dealing with the analysis of human abilities. These are neurosciences, psychology and cognitive sciences. The human brain serves as a model. With its learning architecture, it can handle a wide range of tasks in different situations and environments.
Ramesh has been working for 25 years on suitable methods for the development, formal design, analysis and evaluation of intelligent vision systems. These are optical sensors controlled by intelligent programs.
Design Principles for Reliable Artificial Intelligence
An AI system needs to accurately recognize its environment and also understand differences between different contexts – such as the difference between driving on an almost empty highway and driving in dense city traffic. How safe AI systems really are, depends on the fact that they can make plausible decisions for people and assess their reliability themselves. Above all, the systems must be able to explain their decisions at any time. It is important for developers to clearly distinguish between different areas: User requirements, modelling, implementation and validation. In the same way, the interfaces between them must be precisely defined.
The result is systems that actually identify a child with a dragon mask as such and cannot be disrupted by an elephant in the living room.
AEROBI: AI in practice
Ramesh and his team at the University of Frankfurt have refined and applied these principles over the past seven years. They have developed platforms for rapid prototyping, simulation and testing of vision systems. This included security systems, but also applications for the detection of brake lights in automobile traffic. Recently, as part of the EU project AEROBI, they developed a vision system for an autonomous drone. The drone is to inspect large bridge structures for damage. The Frankfurt scientists developed AI technologies with which the drone can navigate the airspace around the bridge and then go on to detect and classify fine cracks and other irregularities. AEROBI is coordinated by Airbus. The drone has so far been tested on two bridges.
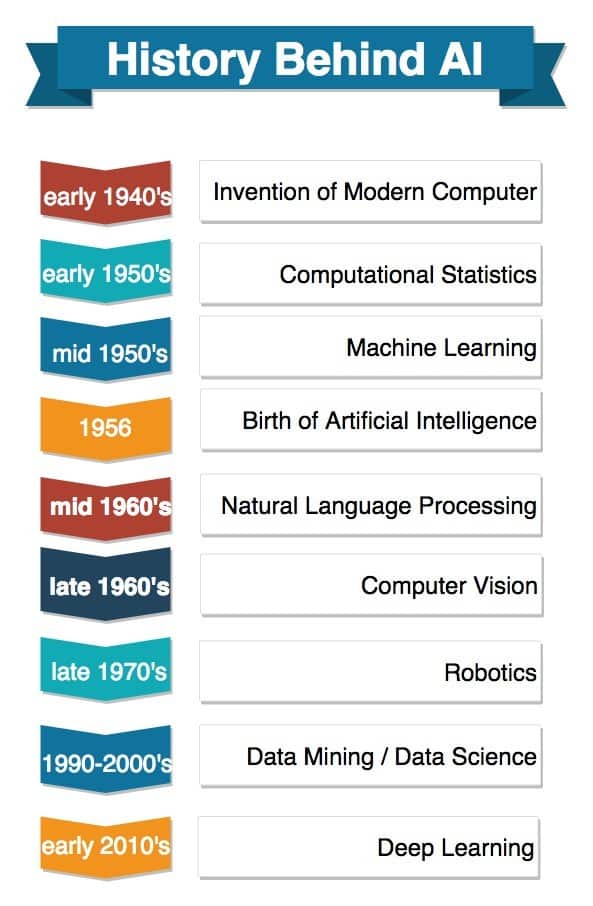