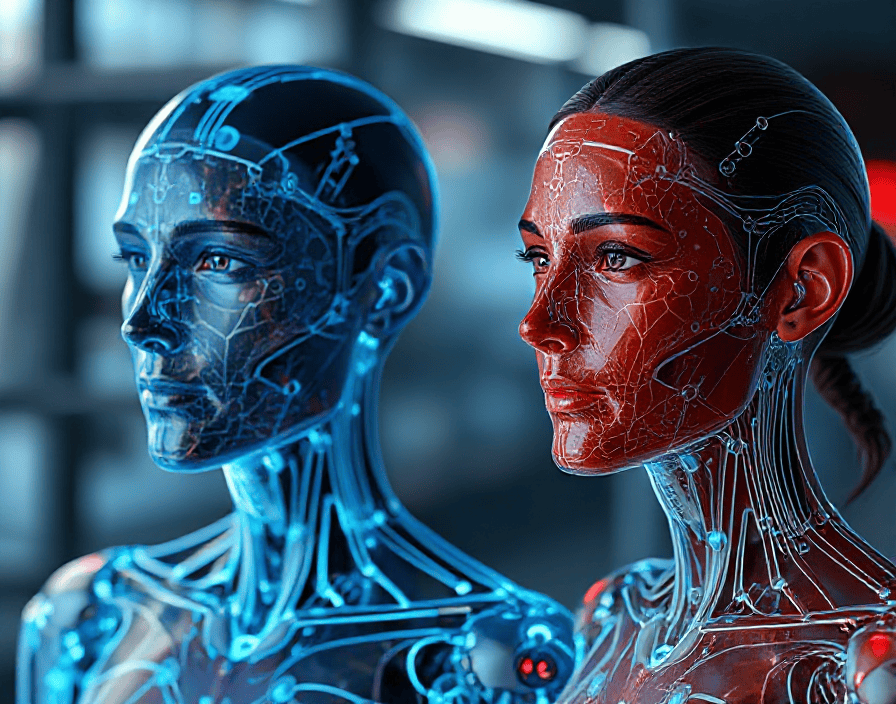
In the rapidly evolving field of marketing and communication, staying ahead means embracing technological innovations. The latest breakthrough, silicon sampling, leverages AI to revolutionize market research by creating synthetic personas that mimic human responses. This method, which utilizes large language models (LLMs) like GPT-4o, offers a cost-efficient and less time-consuming alternative to traditional market research. Roberta Vaznyte and Marieke van Vliet (Fontys University of Applied Science) have explored the promise and challenges of silicon sampling, highlighting key findings from recent experiments and the implications for the future of market research.
Read their full research paper here.
Why is this important?
As AI technology continues to improve, it is becoming more interesting for market researchers to use artificial intelligence to supplement data obtained from real people. It can both save costs and provide richer information. But Fontys researchers discovered limitations as well.
A new frontier
Marketing professionals have always relied on interviews and surveys to understand their target audience. However, finding willing participants and ensuring a representative sample can be challenging and resource-intensive. Enter Silicon Sampling, a novel approach where AI-generated personas simulate real human responses. Companies like OpinioAI and Synthetic Users are at the forefront of this innovation, using LLMs to generate hundreds of qualitative interviews in a fraction of the time it would take with real people.
Paradigm shift
Silicon sampling represents a paradigm shift in market research. Traditionally, personalized research involving in-depth interviews and surveys was essential for understanding customer opinions, beliefs, and behaviors. With silicon sampling, personalized research might become less critical, as synthetic personas can provide insights quickly and efficiently. For instance, OpenAIโs extensive library of GPT versions can be used to create diverse synthetic personas, further expanding the possibilities of this method.
Roberta Vaznyte and Marieke van Vliet designed a small-scale experiment to unravel the complexities of silicon sampling. Synthetic personas were created based on actual interviews with four people in the communication field, and results were analyzed using both qualitative analysis and a linguistic analysis of the interviews. The results shed light on the capabilities and limitations of synthetic personas. The linguistic analysis of the responses revealed significant differences, including the answersโ length, complexity, and authenticity.
Insights
The experimentโs findings highlighted the potential and the challenges of silicon sampling. Synthetic personas generally provided longer and more complex responses than real humans, but their answers often lacked the authenticity and nuance of human responses. For example, synthetic personas scored higher on analytical words but lower on authenticity, indicating a more robotic communication.
One striking difference was the approach to moral questions. When asked about the morality of gathering user data through synthetic personas, a real human respondent emphasized the value of real human insights despite the efficiency of AI. In contrast, the synthetic persona provided a detailed, structured response focusing on ethical principles like informed consent and transparency. This contrast underscores the potential for synthetic personas to offer comprehensive but sometimes overly generalized answers.
Limitations
While silicon sampling offers exciting possibilities, it also has limitations. The experiment revealed potential biases in AI-generated responses and a risk of misrepresenting underrepresented groups. For instance, synthetic personas might rely on data that does not accurately reflect the diversity of real human experiences, leading to skewed insights.
Responsible use of Silicon Sampling is crucial to addressing these challenges. Users must understand the limitations of AI-generated data and use it as a supplement rather than a replacement for traditional research methods. Ensuring diverse data sources and continuous development of LLMs is essential to avoid overrepresenting certain groups and ensure inclusivity.
No replacement
The researchers conclude that Silicon Sampling is not a replacement for traditional market research but a powerful tool that can complement existing methods. As AI technology evolves, further research is needed to explore the full potential of synthetic personas. This includes expanding studies to a broader range of professionals and exploring different AI platforms.