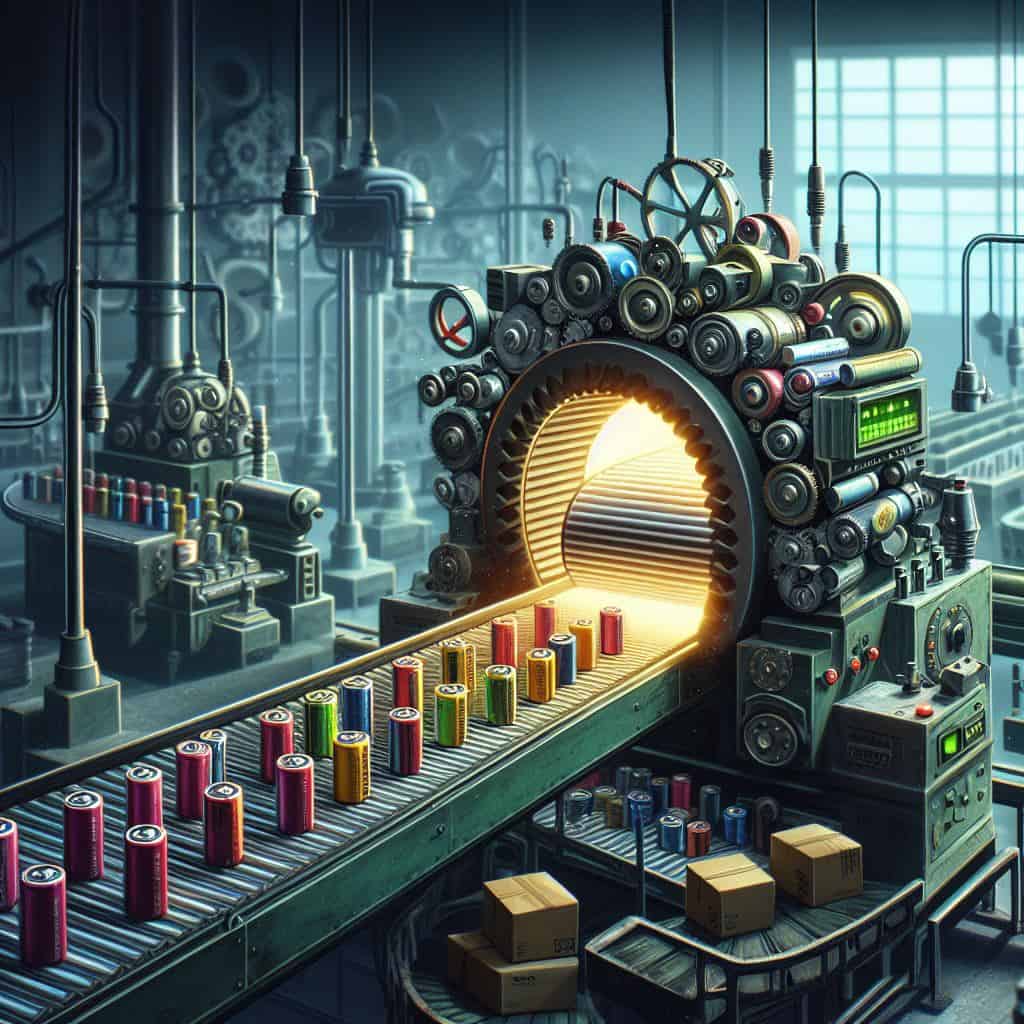
The urgent need for sustainable battery recycling is now addressed by a machine learning system that efficiently sorts retired batteries. With the increased use of batteries, the issue of proper recycling becomes critical. Batteries contain hazardous chemicals that cannot be disposed of in landfills and they include valuable resources. The proposed system significantly boosts sorting accuracy, reaching up to ninty-nine percent precision. This innovative approach could lead to a more profitable and environmentally friendly direct recycling process.
- The Tsinghua-Berkeley Shenzhen Instituteโs breakthrough in battery recycling utilizes federated machine learning, achieving 99% sorting accuracy.
- Direct recycling of lithium-ion batteries proves economically and environmentally superior, preserving resources and privacy with innovative sorting techniques.
- The proposed system not only ensures profitability but also transforms the battery recycling industry towards automation, collaboration, and privacy preservation.
As the world tilts towards cleaner energy and electrically powered devices, the production of lithium-ion batteries (LIBs) is skyrocketing. The global production scale of LIBs is projected to surpass 1.3 terawatt-hours by 2030. However, with the average lifespan of these batteries spanning only 5 to 8 years, there is a looming environmental challenge: the disposal and recycling of retired batteries. This situation not only poses a threat to environmental sustainability but also risks wasting valuable resources such as lithium and cobalt โ materials already in high demand and short supply.
Direct recycling: a sustainable solution
In a breakthrough study published in Nature Communications, the Tsinghua-Berkeley Shenzhen Institute has advanced direct recycling of lithium-ion batteries. This method rejuvenates spent batteries while maintaining their original structure. It eclipses traditional recycling methods โ pyrometallurgy and hydrometallurgy โ in both environmental and economic efficiency, reducing energy use and emissions.
The studyโs key focus is on overcoming the critical challenge of accurately sorting various spent batteries for large-scale recycling success. It sets out a clear strategy for effectively segregating mixed battery materials, a significant step towards sustainable battery reuse.
The role of machine learning
Machine learning has emerged as a promising tool to tackle such complex issues within the battery field. The study utilises federated machine learning โ a method that allows for collaborative and privacy-preserving data analysis โ to sort retired batteries with remarkable accuracy. This system doesnโt require data exchange between contributors, thereby preserving data privacy while leveraging the collaborative effort.
The study introduces a novel technique called Wasserstein distance voting (WDV), which has proven superior to traditional methods like majority voting (MV). The WDV achieves a sorting accuracy of ninety-nine percent. This high sorting accuracy is crucial for the profitability of direct recycling since it ensures that different types of batteries can be sorted accurately and efficiently.
Economic implications of accurate sorting
One of the unique advantages of the proposed system is its ability to sort retired batteries efficiently by using existing data collaboratively and privately. This feature is particularly critical given the sensitive nature of industrial data and the competitive landscape of the battery recycling industry. The federated machine learning framework, especially with the WDV strategy, enables this profitable and privacy-preserving recycling to become a reality.
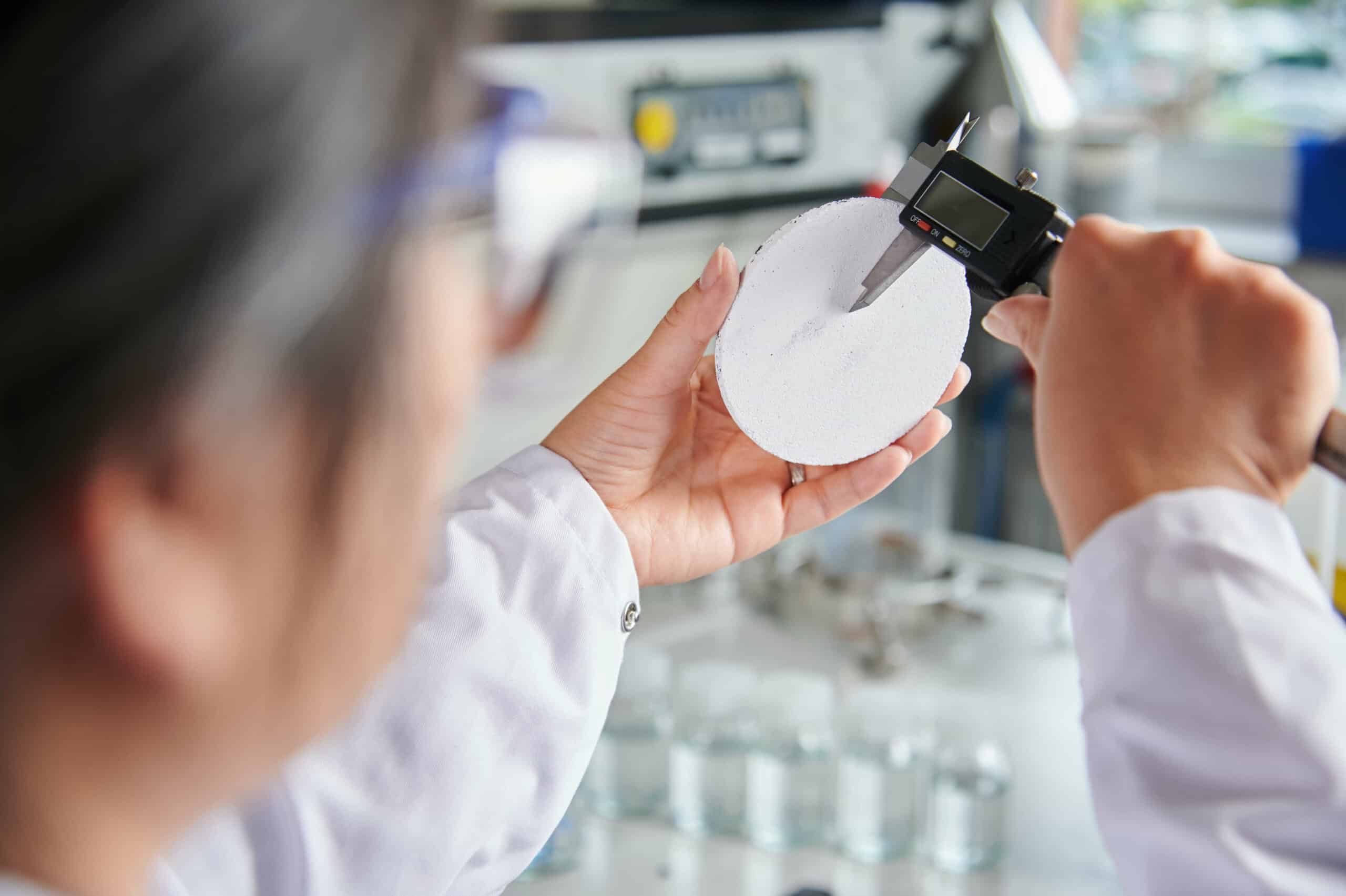
Next-generation battery recycling will have to manage a variety of battery types and conditions. Direct recycling will need to sort batteries not only by material type but also by their state of health (SOH), which directly determines the amount of reagents required in the recycling process. The federated machine learning framework, with its capability to handle heterogeneous data and preserve privacy, stands poised to tackle these challenges effectively.
Environmental and operational benefits
The implications of this research extend beyond the economic benefits. The high sorting accuracy of WDV ensures that batteries can be sorted completely, allowing them to be directly used to assemble new batteries. This process minimises environmental impact, simplifies operations, and enhances privacy and data sharing capabilities. With such a system in place, the battery recycling industry could undergo a transformative shift from the current labour-intensive methods to an automated, collaborative, and privacy-preserving model.