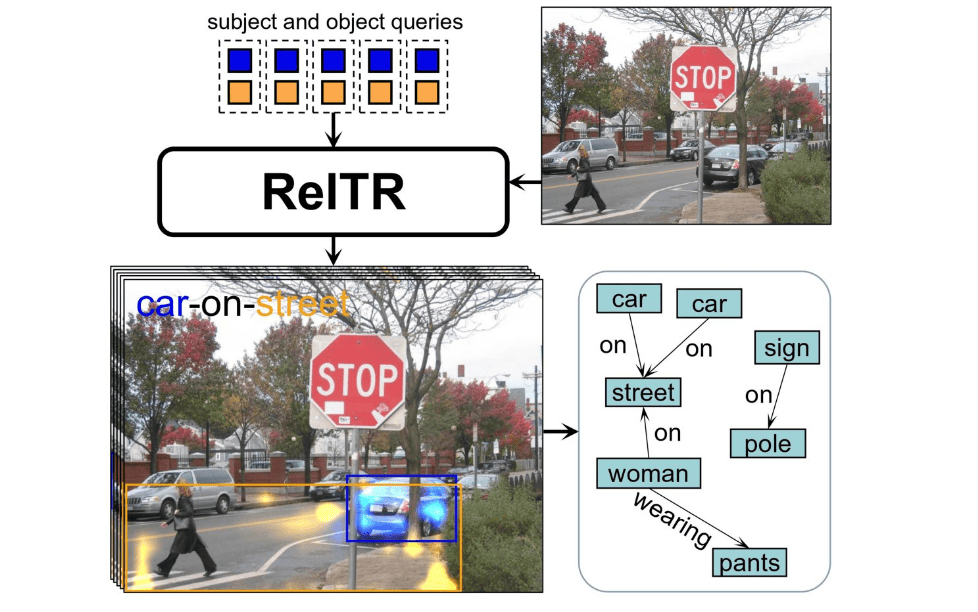
University of Twente researcher Michael Ying Yang has developed a groundbreaking method for generating realistic and coherent images from textual prompts using AI. The new approach, called ReITR, focuses on creating scene graphs, which are crucial for enhancing AI’s understanding of visual relationships between objects, the university writes in a press release.
This innovative one-stage method predicts subjects, objects, and their relationships simultaneously, significantly reducing processing time compared to traditional two-stage approaches. The research, published in the scientific journal IEEE T-PAMI, promises to improve image generation, as well as the perception of autonomous vehicles and robots.
- ReITR (Relation Transformer for Scene Graph Generation) generates realistic and coherent images from textual prompts using AI.
- ReITR focuses on creating scene graphs, which enhance AI’s understanding of visual relationships between objects and significantly reduces processing time compared to traditional two-stage approaches.
A Leap Forward in Scene Understanding
Artificial intelligence has made significant strides in generating images from textual prompts, but most generative AI models excel at creating images of single objects rather than complete scenes. Humans, on the other hand, are adept at defining relationships between objects, such as understanding that a chair is on the floor or a dog is walking on the street. To bridge this gap, Yang, an assistant professor at the Scene Understanding Group of the Faculty of Geo-Information Science and Earth Observation (ITC), developed a novel method called ReITR (Relation Transformer for Scene Graph Generation).
This new approach constructs scene graphs from images, serving as blueprints for generating realistic and coherent images. By improving AI’s ability to detect and understand visual relationships between objects, ReITR not only enhances image generation but also aids the perception of autonomous vehicles and robots, which require accurate scene understanding to navigate and interact with their surroundings.
One-Stage Method for Faster Processing
Traditional methods for graphing a semantic understanding of an image use a two-stage approach, which is slow and inefficient. The first stage maps all objects in a scene, while the second stage employs a specific neural network to go through all possible connections and label them with the correct relationship. However, the number of connections this method has to process increases exponentially with the number of objects, making it time-consuming.
ReITR, on the other hand, takes just a single step to achieve the same goal. It automatically predicts subjects, objects, and their relationships at the same time, drastically reducing processing time. ReITR’s model examines the visual features of objects in a scene and focuses on the most relevant details for determining their relationships. It highlights essential areas where objects interact or relate to each other, using these techniques and relatively little training data to identify the most crucial relationships between different objects. The model then generates a description of how the objects are connected.
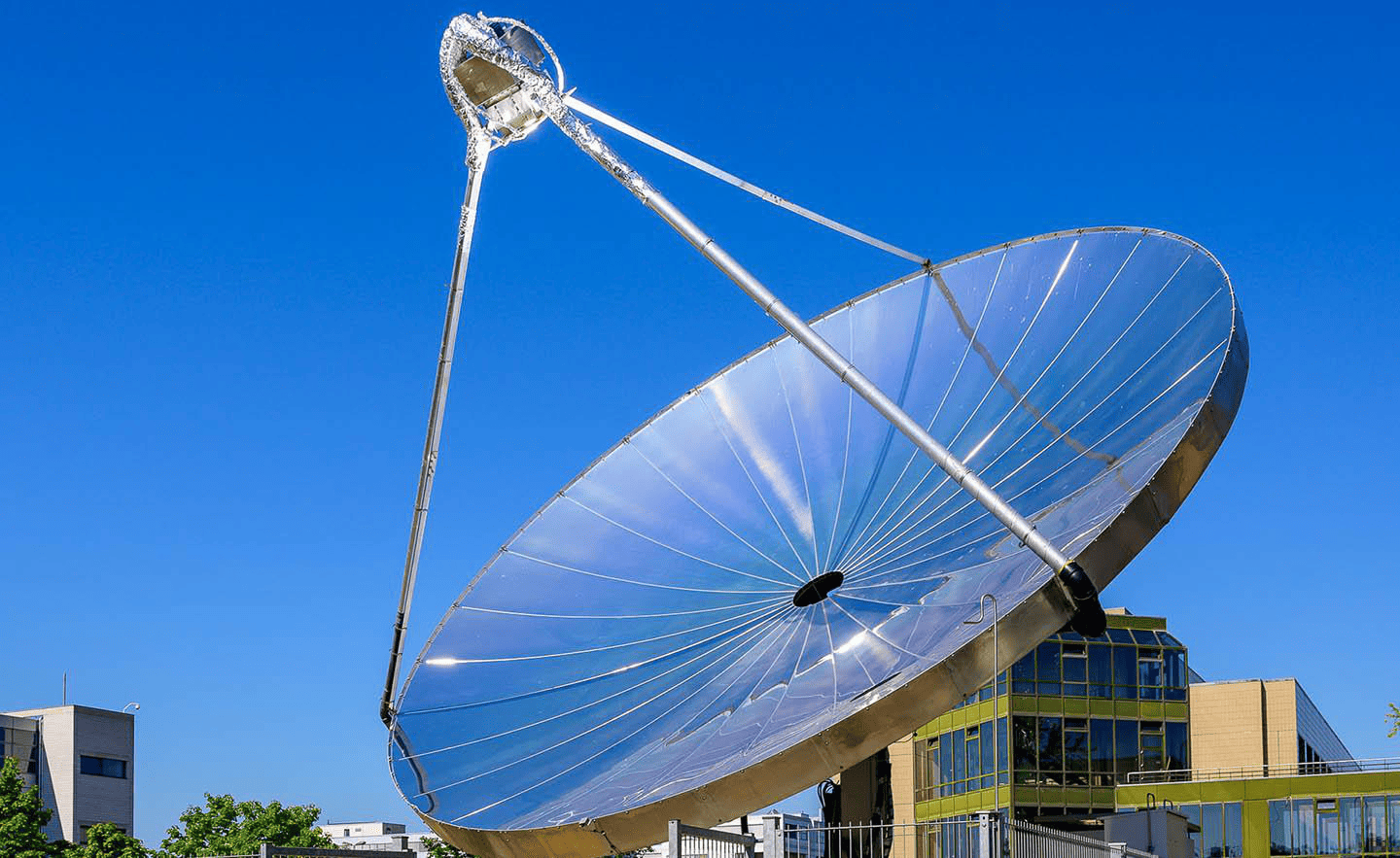
Practical Applications and Future Developments
ReITR’s advancements in scene understanding have the potential to impact various fields and applications. For instance, the breakthrough could benefit autonomous vehicles and robots, which rely on accurate scene understanding and object recognition to navigate and make decisions. Moreover, ReITR’s ability to generate realistic and coherent images from textual prompts could find applications in computer graphics, virtual and augmented reality, and even video game design.
While ReITR represents a significant step forward, it is part of a broader landscape of research into AI’s ability to process 3D data and understand scenes. For example, Google Research’s TensorFlow 3D library is designed to bring 3D deep learning capabilities into TensorFlow, enabling scene understanding technology for machine learning systems like autonomous cars and robots. As research continues to advance in this field, we can expect further innovations and applications that harness AI’s potential for image generation and scene understanding.