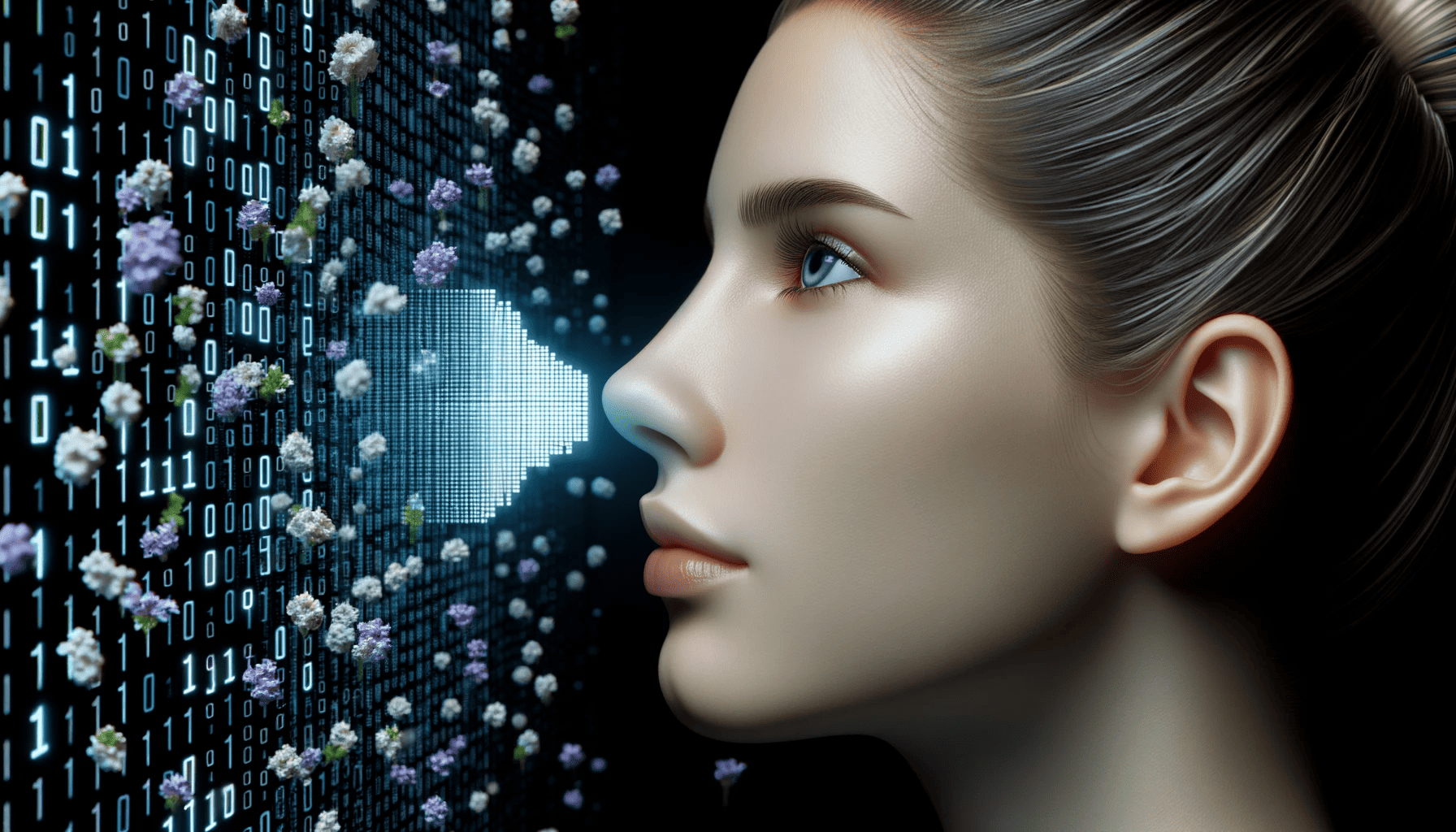
AI has finally beaten human smell. Applying machine learning to quantify, digitize, and engineer scent is now possible. Osmo AI has developed the Principal Odor Map (POM) as the olfactory version of RGB for colors. The map can predict scents based on molecular structures, surpassing human accuracy in 53% of tests. A recent paper published in Science proves the map’s usefulness by predicting the scent of molecules that had never before been smelled. With this tool, half a million potential scent molecules can be mapped.
With the study, Osmo AI, with experts from the University of Reading, Monell Chemical Senses Center, and Arizona State University, has made a significant leap in olfaction technology. Their revolutionary graph neural network-based model was validated on 400 structurally diverse compounds and further demonstrated its broad applicability by assessing the olfactory properties of half a million potential scent molecules. The development of this digitized scent perception technology is a key advancement in understanding the complex world of olfaction, with potential implications for human health, product safety, disease detection, and waste prevention. However, challenges remain, including predicting the intensity of smells, blending molecules, and integrating chemical sensor measurements.
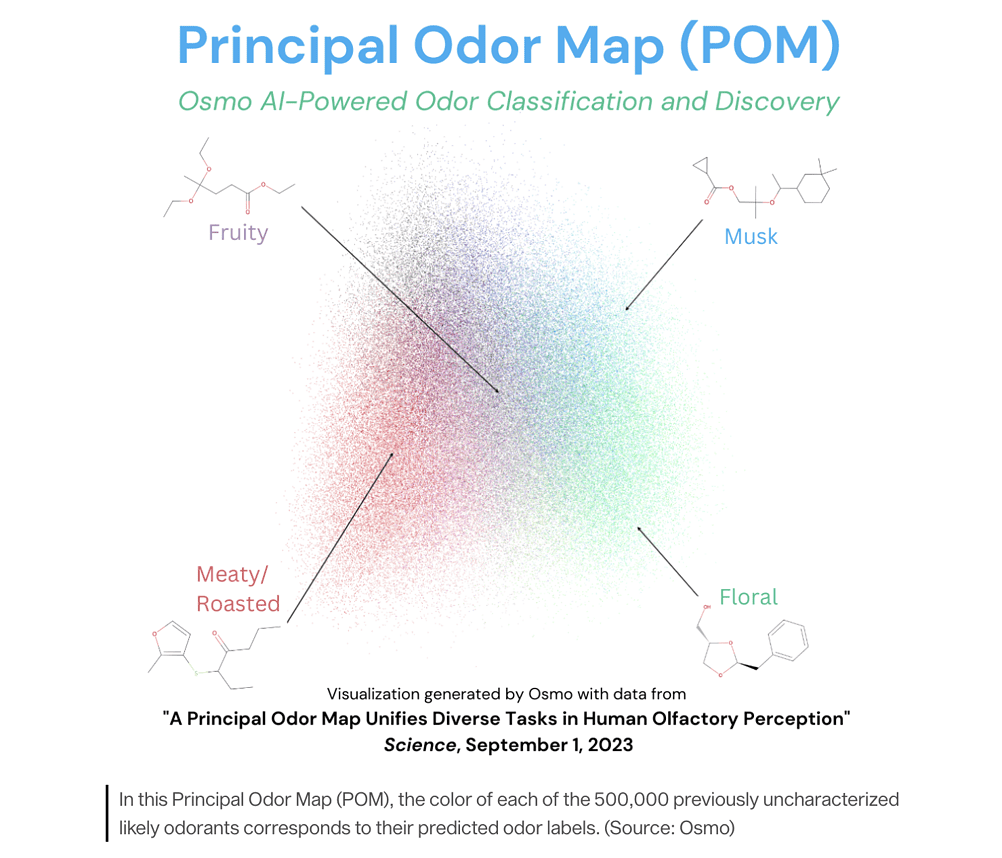
The technology behind Osmo AI’s model
Osmo AI has developed a unique method to digitize a sense of smell, which involves three key steps: reading, mapping, and writing. The first step, reading, is the conversion of atoms into digital bits. This resembles how a camera captures light, or a microphone records sound. The second step involves mapping the sensory world by understanding and organizing these digital bits. This is akin to using RGB for color or frequency for sound. The final step, writing, involves turning these digital bits back into atoms that can be perceived, similar to how a printer or speaker works.
In the case of smells, Osmo AI turns odor molecules into digital signals and then organizes these signals according to how they are perceived as scents. The digital representations are then converted back into real scents. The digitization of scent begins with creating a map of odors and converting scents from the map into fragrance ingredients. The company is working towards the end-to-end reproduction of a captured scent, an ‘Osmograph’.
Practical applications
The practical applications of Osmo AI’s technology extend beyond the fragrance industry. The company’s ultimate goal is to improve the health and well-being of human life through smell. This is achieved by digitizing the sense of smell, our oldest and most profound sense. Osmo AI envisions that the digitization of smell can activate treasured memories and evoke profound emotions, much like a photo or a song.
The technology could also be used in detecting diseases or preventing food waste. By digitizing the sense of smell, which is often the first to detect anomalies, early detection of diseases could be possible. The technology could also provide an effective way of identifying food that is no longer safe to consume, thereby preventing food waste.
“Our model performs over 3x better than the standard scent ingredient discovery process used by major fragrance houses”, said Osmo’s CEO Alex Wiltschko. “And it is fully automated. This discovery is the foundation of everything that Osmo will do next. Moving forward, we’ll be using this technology to discover the next generation of safe but powerful fragrance ingredients both in our laboratories and in partnership with leading industry players.”
Challenges and limitations
Despite the potential benefits, Osmo AI’s technology also faces several challenges. These include predicting the intensity of smells, blending molecules, and integrating chemical sensor measurements. The digitization of smell is a complex task that requires a sophisticated understanding of the molecular structure of scents and how individuals perceive them. Dealing with the subjectivity of smell perception among individuals is a significant challenge. Similarly, the model must also handle complex scents composed of a mixture of different odor molecules.
Furthermore, the model’s prediction capabilities could be affected by factors such as the age or health of the individual whose sense of smell is being replicated. As the sense of smell can change over time or due to health conditions, the model’s accuracy in replicating these changes is critical to its effectiveness.