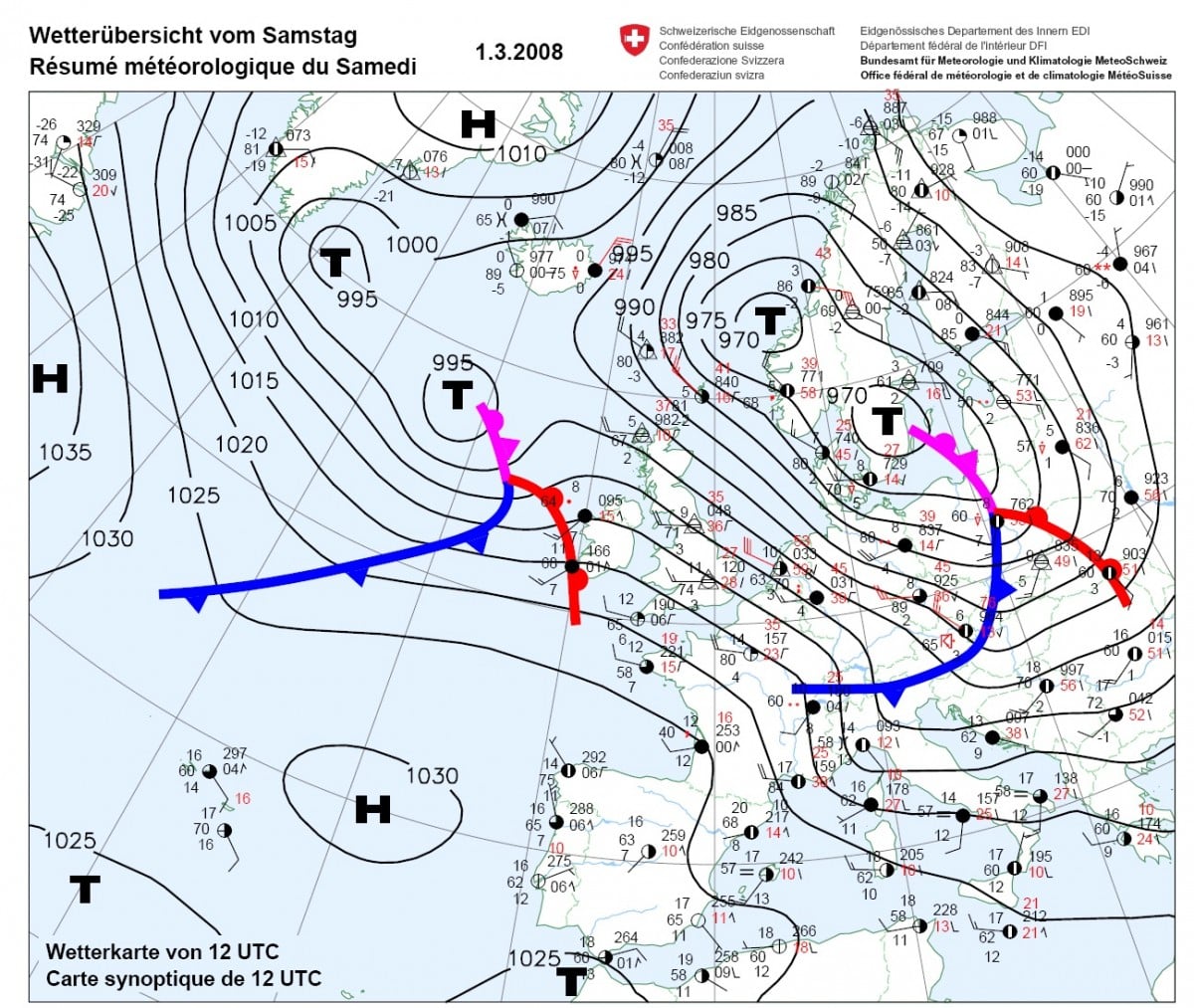
Weather forecasts often change daily and even hourly. And depending on which channel you watch on TV or which app you use on your mobile phone, there are immense differences for the same day. However, it is not the inability of the meteorologists but the “chaotic system” atmosphere that is to blame for the deviations or even false predictions. “Physical properties such as temperature, humidity or cloudiness change constantly,” explain scientists from the Karlsruhe Institute of Technology (KIT). It can happen that thunderstorms, sun, cold and heat follow each other on one day – like the weather this May.
Meteorologists are therefore faced with the challenge of predicting this chaos and making reliable statements. They assume current measurements in the atmosphere and simulate alternative scenarios. For example, they calculate how changes in temperature or humidity could affect the weather and compare up to 50 scenarios for each parameter. “If the results are similar, this indicates that a forecast with these values is relatively reliable and that the state of the atmosphere in this area is stable and predictable,” says Dr. Peter Knippertz of the Institute for Meteorology and Climate Research at KIT.
Nevertheless, this method does not guarantee truly reliable predictions. “The computer scenarios cannot map some physical relationships with the necessary depth of detail or spatial resolution,” explains Dr. Sebastian Lerch from the Institute of Stochastics at KIT, who works closely with Knippertz. For example, temperature predictions were always too mild at certain locations and too high at others, because “local conditions, some of which are time-variable, cannot be given to the models”. Therefore, it is necessary to post-process the results of the simulations with elaborate statistical procedures and expert knowledge in order to obtain better forecasts and probabilities of occurrence for weather events.

INFORMATION MODELLED ON THE HUMAN BRAIN
“Most weather forecasts today are based on physical models of the atmosphere calculated on supercomputers. In order to take into account uncertainties (e.g. in initial conditions or details of the physical models), ‘ensemble simulations’ are used, meaning several simulation runs, for example with varying initial conditions,” Lerch describes. “However, the predictions of these ensemble simulations usually show systematic errors that have to be corrected by statistical methods. Based on predictions and observations from the past, statistical models are used to correct the errors. In the past, only ensemble predictions of the target were used: So if you want to correct the temperature predictions, only temperature predictions were used as input.”
Lerch and his colleagues from meteorology and mathematics at KIT have now developed a new method based on artificial intelligence to avoid errors in weather forecasts. “We have developed an approach that provides better predictions than established standard methods,” said Lerch. This approach involves neural networks that process information according to the model of the brain. The computer programs are trained to process certain data optimally. Like the human brain, the neural networks collect “experience” during training and can thus continuously improve – but much faster than a human brain. “The most complex network-based models take about 25 minutes to train (on a single Nvidia Tesla K20 GPU),” emphasizes Lerch. Ideally, the networks can then precisely determine, for example, the probability of local weather events such as thunderstorms occurring.
Weather models would produce predictions for a variety of other variables that influence temperature, such as pressure, cloud cover, solar radiation, wind, etc., Lerch continues. “The concrete functional dependencies of the temperature prediction errors on these input variables is very complex and non-linear, and therefore cannot be described in a simple functional form even by meteorological experts. While standard methods require such a functional description of the dependencies for the formulation of the models, the neural network-based models proposed by us ‘learn’ these dependencies independently, without the specification of concrete functional correlations”.

WEATHER DATA FROM TEN YEARS FOR TRAINING
To train the network, the scientists used weather data from Germany, which 537 weather stations had recorded from 2007 to 2016. Input parameters for the neural network included cloud cover, soil moisture and temperature. The researchers then compared the forecasts made by the network with forecasts from established techniques. “Our approach has made much more accurate predictions for almost all weather stations and is much less mathematically complex,” summarizes Lerch. And can it be said how much more accurate the forecasts are than before? Yes, says the scientist.
The quality of the predictions would be measured using the continuous ranked probability score (CRPS). “This is a mathematically based evaluation rule for predictions in the form of probability distributions that takes into account the uncertainty quantified in the prediction. Averaged over all days and stations, a CRPS improvement of about 3% is achieved compared to the best standard procedures,” he explains. “The network-based models provide the best predictions at about 80% of the 537 observation stations. In paired comparisons, the observed CRPS differences between network-based models and the best standard procedures are statistically significant in between 30% and 67% of the observation stations.
Next to their learning ability and the fact that they can recognize non-linear relationships independently, neural networks have another advantage: they can process large amounts of data faster than previous methods and human experts. “The two previous standard methods, which can process the same number of predictors as the most complex network models, took 48 minutes or 430 minutes to train (i.e. about two or 17 times as long),” says Lerch. The direct comparison is difficult due to the different programming environments (Python for the network models, R for the standard procedures) and hardware environments (GPU for the network models, CPU for the standard procedures), but: “For the first time, we were able to show that neural networks are ideally suited for improving weather forecasts and obtaining information about meteorological processes.
You can find out more about Artificial Intelligence here.