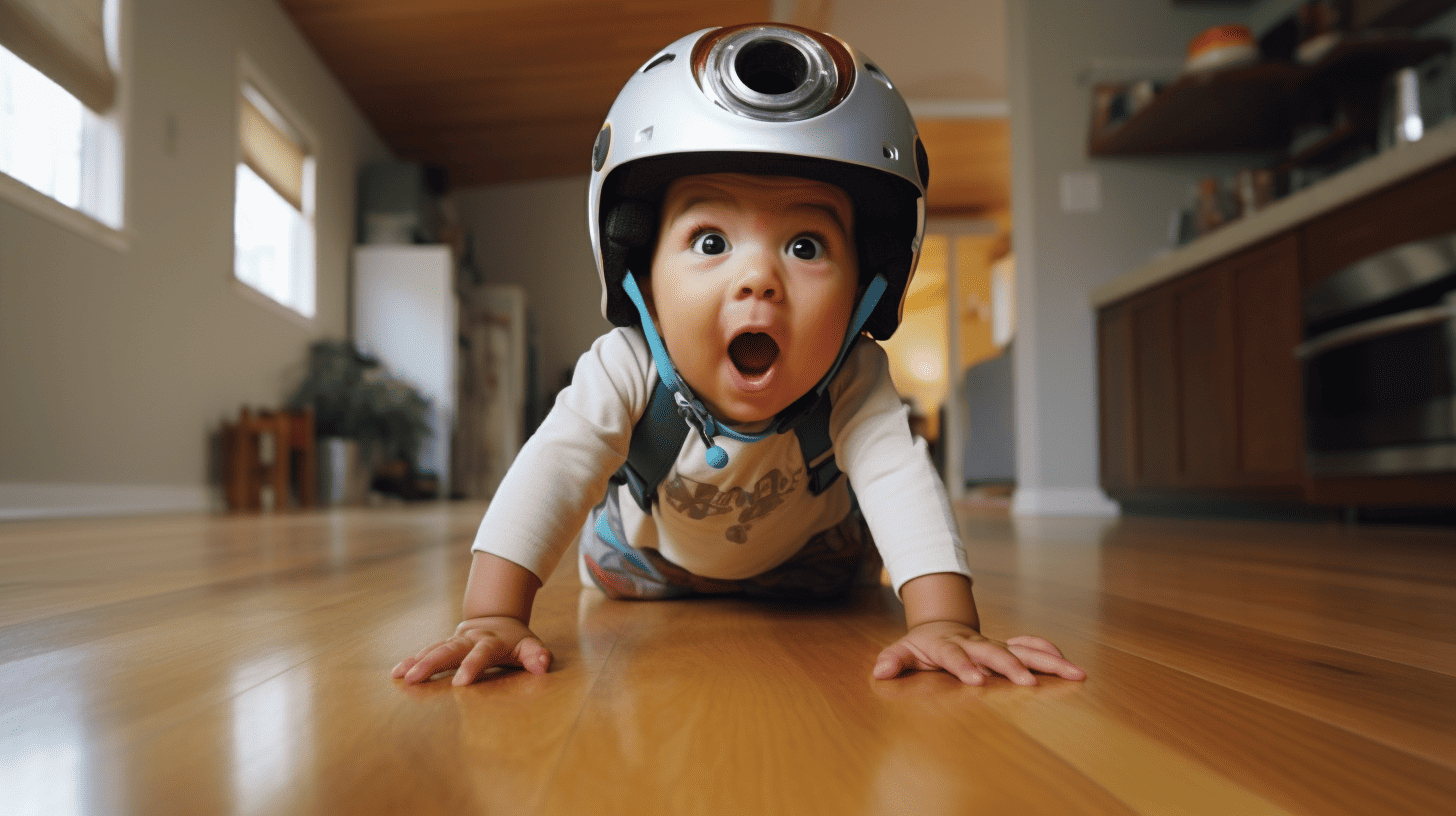
In a groundbreaking study published on Science Today, an AI model trained on a mere 61 hours of a child’s life has astonishingly grasped a fundamental language element: connecting words to their corresponding objects. This research, spearheaded by New York University, utilized footage from a head-mounted camera on a toddler to feed the AI 600,000 video frames alongside 37,500 spoken phrases. The child’s ability to learn language with limited data outshines current large language models, revealing a potential pathway to more efficient and human-like AI learning. The project aims to edge artificial intelligence closer to human cognition, addressing the current limitations of AI’s brittle nature and lack of common sense.
Why you should read this
While powerful, the current generation of AI systems often lacks the flexibility and common-sense reasoning that come naturally to humans. The support of a child’s fresh eyes can help improve AI’s capacities.
As the world grapples with the intricacies of artificial intelligence, a new frontier in machine learning is emerging from an unexpected source: a child’s perspective. The latest research from New York University delves into the essence of language learning by observing the world through the eyes of an infant. The study, which involved a neural network model, has provided compelling evidence that AI can acquire language in a manner akin to human infants. This discovery could revolutionize our understanding of both cognitive development and AI.
From infant observations to AI learning
The methodology behind this remarkable feat was both innovative and meticulous. Scientists equipped an Australian child, known simply as Sam, with a head-mounted camera, capturing his daily experiences from the age of six months to two years. This visual diary comprised 61 hours of footage, covering just 1% of Sam’s waking hours. Yet, this seemingly insubstantial glimpse into a child’s world allowed the AI to make significant strides in word recognition.
By pairing the video frames with the spoken phrases from Sam’s environment, researchers amassed a dataset of 600,000 frames and 37,500 instances of speech. This data became the training ground for the AI, which was not pre-programmed with any prior knowledge of language. The AI’s education was entirely reliant on the associative learning of words and objects as they co-occurred in Sam’s field of vision.
AI’s leap in learning
The AI’s learning process was not simply a matter of rote memorization. It employed a technique known as contrastive learning, which recognizes patterns based on the frequency and context in which words and objects appear together. This approach mimics the natural learning process of children, who often learn to speak and comprehend their native language without explicit instruction but rather through immersion and interaction within their environment.
Interestingly, the AI model demonstrated an ability to generalize its learned knowledge. When faced with a choice between multiple images, the AI could correctly identify the one that matched a target word. The model displayed a 62% success rate in object recognition, a figure significantly higher than the 25% chance level and comparable to more extensive AI models trained on vast datasets far beyond what Sam’s experience provided.
Challenging traditional theories
This study not only showcases the potential for AI to learn in more human-like ways but also challenges long-held views in cognitive science. The prevailing notion that language acquisition requires specialized mechanisms or innate knowledge is put to the test, as the AI’s performance suggests that exposure to naturalistic human environments could be sufficient for learning the core aspects of language.
Moreover, the research has broader implications for the field of AI. While powerful, the current generation of AI systems often lacks the flexibility and common-sense reasoning that come naturally to humans. AI could overcome these limitations by adopting learning strategies observed in children, leading to more robust and adaptable applications.
Fresh eyes
The significance of this research extends beyond the laboratories and into the real world, where the applications of such an AI learning model are vast. The possibilities are manifold, from enhancing educational technology to improving natural language processing systems. The study’s revelations on language acquisition also offer a beacon for future cognitive science research, promising deeper explorations into the marvels of human learning and cognition.
In essence, the seemingly simple act of capturing the world through a child’s eyes has initiated a profound leap forward in AI development. It reveals the transformative power of viewing the world with fresh eyes.