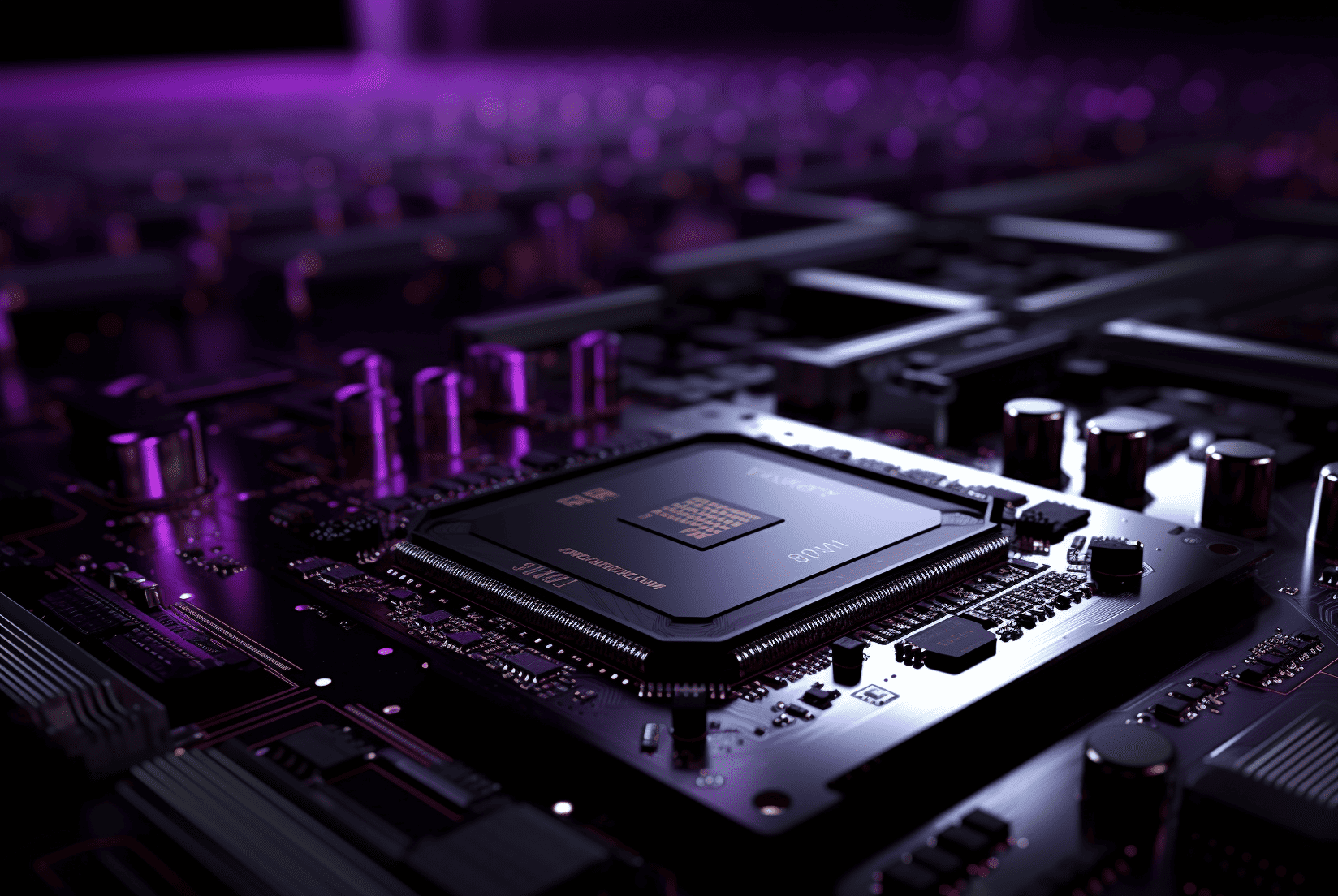
AI is a rapidly evolving field that requires high-performance and specialized hardware to run its complex and data-intensive algorithms. AI chips are dedicated devices that can handle these tasks faster and more efficiently than traditional processors. They enable parallel computing, neural network architectures, and optimized memory structures that boost the performance of AI applications. Many tech giants are investing heavily in developing and deploying their own AI chips, either for their own use or for the market. NVIDIA and AMD, the leading GPU makers, are tailoring their products for AI applications. Google and Amazon have their own custom chips in their data centres, powering their AI services. Apple has integrated AI capabilities into its own processors, enhancing its devices. And OpenAI, the current market leader, is exploring the possibility of creating its own hardware. The AI chip market is projected to grow exponentially, reaching $227 billion by 2032, and these companies are competing fiercely to dominate this emerging field.
- Demand for AI capabilities surging, prompting tech giants to develop specialized AI chips optimized for machine learning.
- Intensifying race to meet demand and reduce costs will shape future of fast-growing AI chip market, forecast to reach $227B by 2032
- OpenAI reportedly exploring possibility of developing its own AI chips, joining other tech giants in chip market.
The technical distinction between AI chips and traditional CPUs
The need for specific hardware to run AI applications arises from the unique requirements of these tasks. A traditional Central Processing Unit (CPU) is designed for a wide range of tasks and executes instructions sequentially. However, AI workloads, such as training complex models or processing large amounts of data, require parallel processing capabilities that can handle many tasks simultaneously. AI-optimised chips, such as Graphics Processing Units (GPUs), Tensor Processing Units (TPUs), and other application-specific integrated circuits (ASICs), offer this capability. They are characterised by features such as more cores, more threads, more vector units, more tensor units, more memory bandwidth, more memory capacity, more memory hierarchy, and more specialised instructions. These features allow AI-optimised chips to perform complex and repetitive operations on data faster and more efficiently than CPUs.
The battle for AI hardware dominance
With the global AI chip market expected to grow from $17 billion in 2022 to $227 billion by 2032, the competition among tech giants to dominate this field is heating up. NVIDIA, the current market leader, has a stronghold on the GPU market within the data centre space with a market share of over 95%. Its powerful GPUs and strategic partnerships with Amazon Web Services (AWS) and Azure have helped it maintain its dominance. AMD, however, is challenging NVIDIA’s supremacy with its new AI accelerator chips, the Instinct MI300A and PyTorch partnership. AMD’s HIP, a CUDA conversion tool, and its upcoming processors pose a significant threat to NVIDIA’s market position.
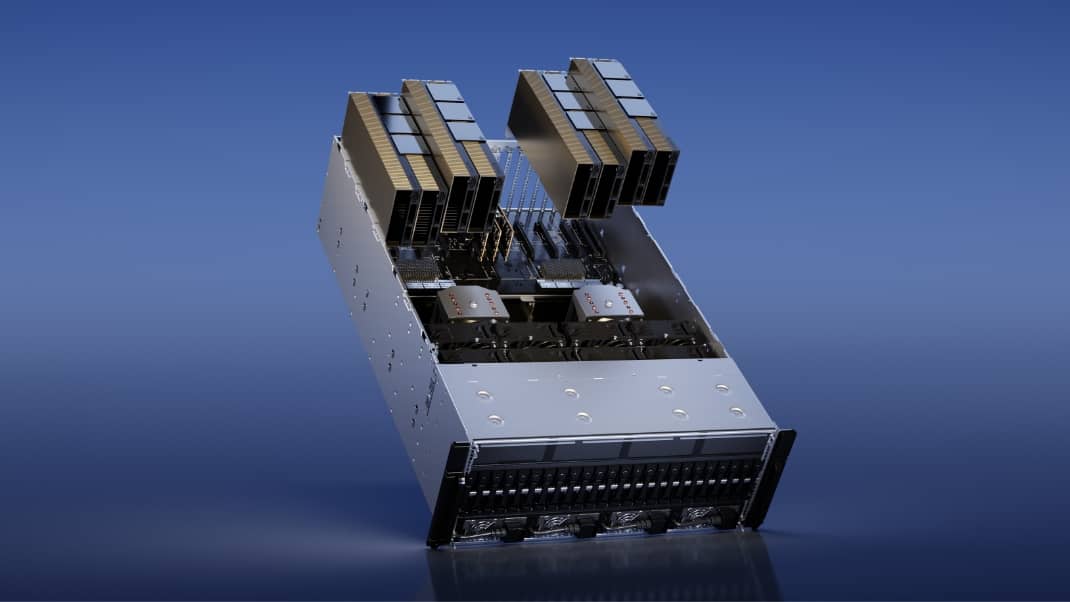
Google and Amazon’s in-house AI chips
Google and Amazon, while not selling chips, have developed their own AI chips for in-house use. Google has developed an AI model that can design complex chips in hours, a task that takes months for human engineers. The AI chip, called TPU (Tensor Processing Unit), is designed for machine learning tasks and can handle trillions of operations per second while consuming low power. Up until now these chips where only used in Google data centers. However, recently Google has introduced its third-generation AI chip, the Tensor G3, in the latest Pixel 8 and Pixel 8 Pro phones.
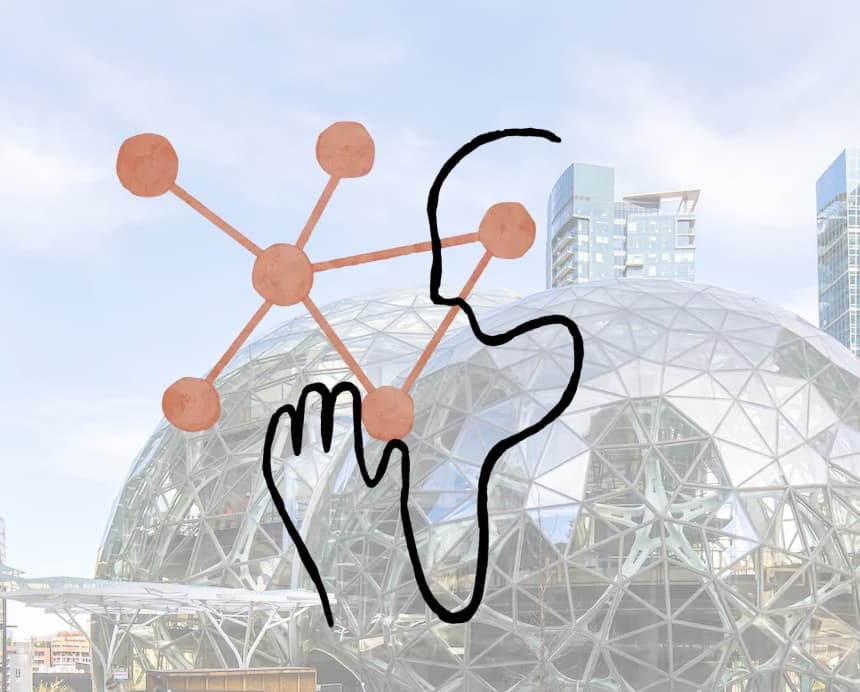
Amazon Web Services (AWS) has announced the general availability of its custom AI accelerator, Trainium. Designed for training large machine-learning models, Trainium offers up to 50% cost savings compared to comparable Amazon EC2 instances. The Trainium accelerators are optimised for training natural language processing, computer vision, and recommender models used in various applications. Amazon and AI research firm Anthropic have formed a $4 billion partnership to advance generative AI with AWS infrastructure and custom chips.
Microsoft’s strategic alliance with AMD
Microsoft has reportedly collaborated with AMD to support the chipmaker’s expansion into AI processors. The partnership aims to challenge NVIDIA’s dominance, which currently holds an estimated 80% market share in the AI processor market. AMD is assisting Microsoft in developing its own AI chips, codenamed Athena, with hundreds of employees working on the project and a reported investment of $2 billion.
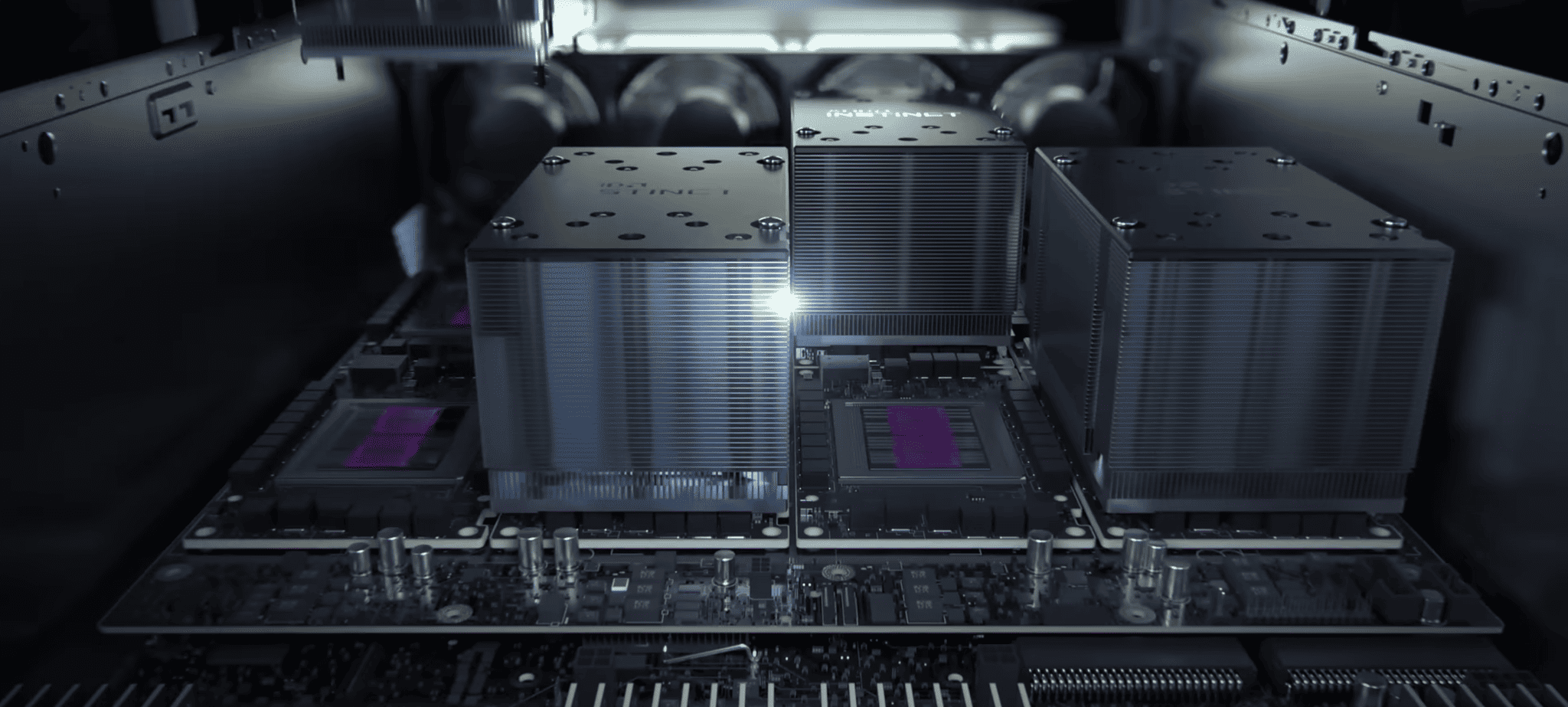
The future of AI chips
The future of AI hardware looks promising, with tech giants and startups alike investing heavily in AI chip development. However, the road ahead remains complex and challenging. OpenAI, currently leading in the AI field, is exploring the development of its own AI chips. The company is considering acquiring an AI chip manufacturer or designing chips internally, which could disrupt the market and reshape the competitive landscape.
The development and deployment of AI chips are not without challenges. The AI chip business is challenging and risky, and the impact of Google, Amazon, AMD, NVIDIA and potential new entrants like OpenAI will be determined by their ability to advance breakthroughs in various sectors, their strategic alliances and partnerships, and their ability to navigate supply and demand dynamics in the global chip market.