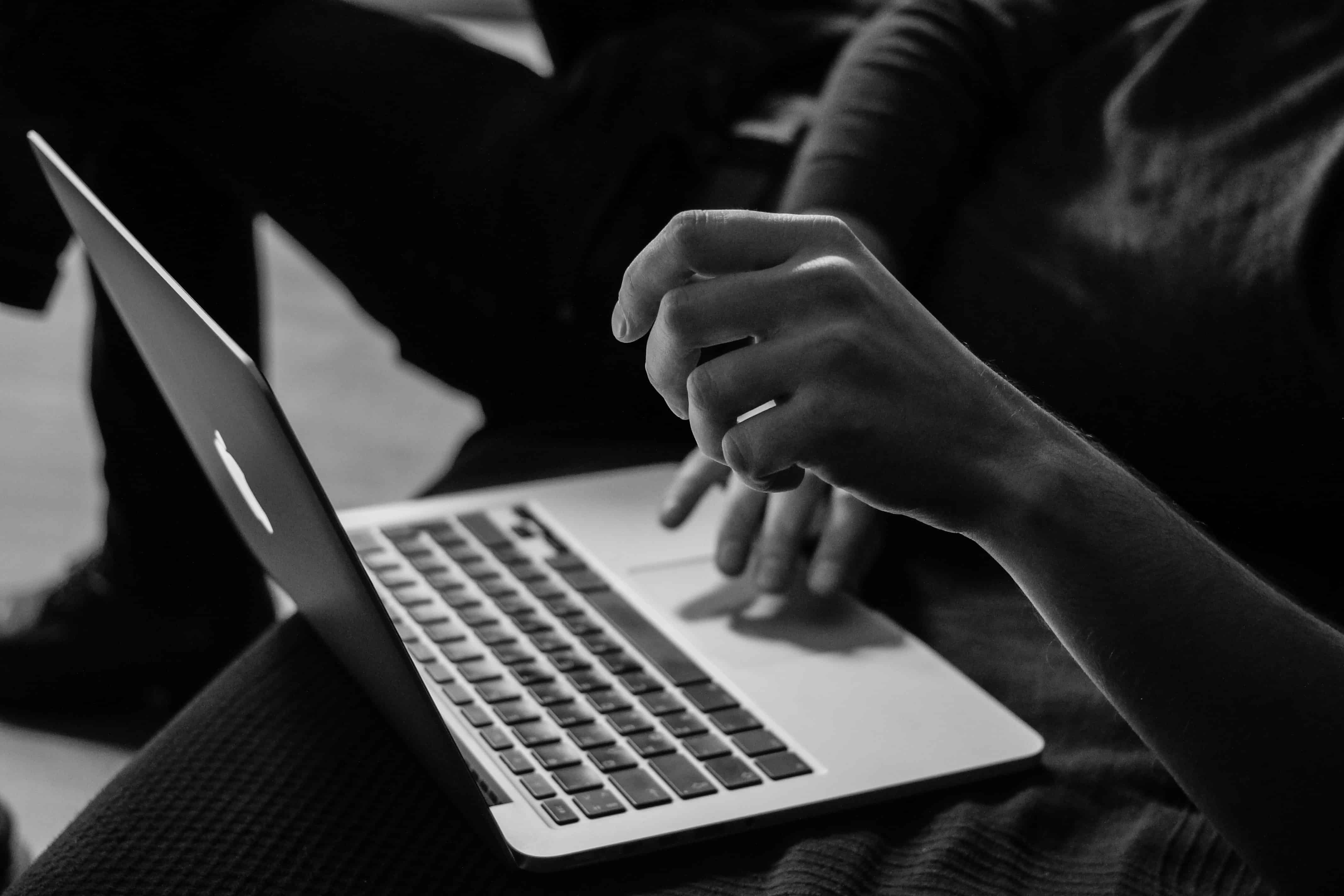
Dealing with customer requests, issues and complaints has always been a crucial part of building and maintaining a thriving business. Arguably, in some sectors, it is the most important part. We can afford to say that this is and has been common knowledge for a long time and we certainly did not need Artificial Intelligence to understand how satisfactory provider-customer relationships in business settings facilitate successful commerce. Nevertheless, a field which in the near future may upset state of the art of business relationships, is that of Generative Artificial Intelligence.
Put like that, it may sound like a buzzword. So, what do we really mean when we talk about Generative AI in business and business improvement? Heath Ramsey, vice-president at US software development firm ServiceNow, has discussed the matter in a presentation he gave this week at Eindhoven’s High Tech Campus.
A three-step model
He explained that the technology – which could be defined as a model that can produce text, images and other pieces of media in response to a prompt – may bring us towards a more and more automated brand of communication and company structure. Ramsey believes that the matter of what he defines as the “customer problem in generative AI” can be summarised in three key steps, which look towards the automation of some part of the hierarchical structure of a company.
The process starts with intake, where an AI model takes in a prompt given by a user. Then, there is comprehension, the step entailing efficient understanding on the machine’s part. In other words, the AI needs to be able to recognize, in sufficient detail, what the prompt is about. Lastly, response: refining a pertinent, case-specific reply to the original prompt.
According to Ramsey, in turn, these three components, in turn, should be used to drive the business outcomes of automation, acceleration and augmentation. In this context, acceleration means that AI should facilitate companies’ path towards self-sufficiency and stability, while augmentation refers to AI’s ability to adapt and transform in short periods of time, following business necessities, as Forbes explains.
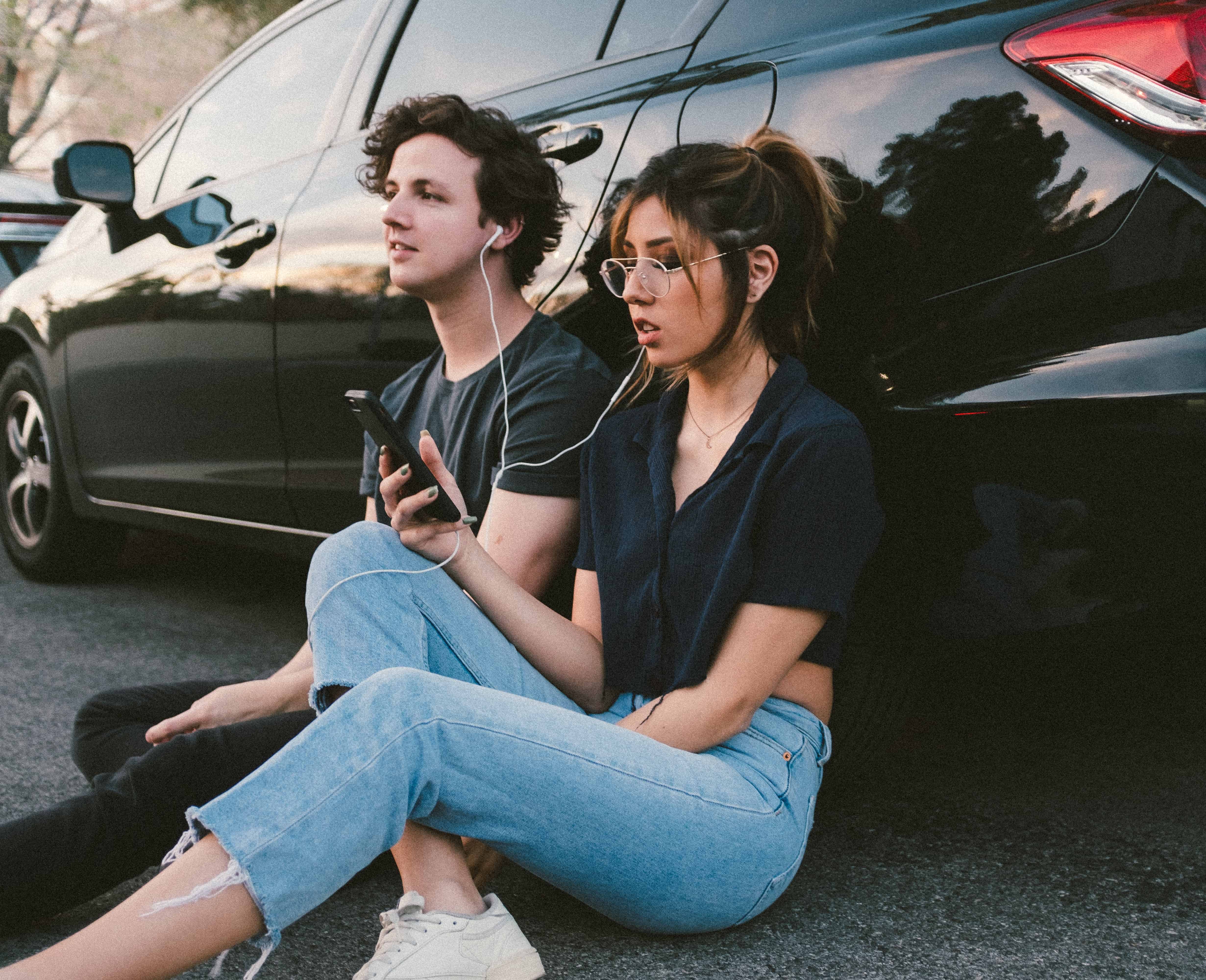
Generative AI’s fair share of challenges
Now, it would be amazing if everything could run in such a simple fashion, but this is not the case. The challenges to consider are many and rather complex. First of all, the usual problem is money: single transactions operated by AI are currently relatively pricey. The “number on the tag” can vary, but generally costs constitute an issue. Secondly, now more than ever people are becoming increasingly conscious and concerned about data retention. Where does data that is fed to AI go? Is it trackable? Is it secure? Along with brand reputation, possible issues of public trust towards machines, poor response quality and misalignment with workflows, these are areas of interest that cannot be ignored.
From general to specific
Also, there is a conversation around the topic of generalizability. Let’s suppose for instance, that a customer has to cancel a strictly non-refundable stay at a hotel because of a terrible, unpredictable event, like the sudden death of a loved one. In the case of bilateral human interaction, a human could decide to apply an exception to the policy and grant a refund to the customer, out of pure compassion. Likely, this “sub-optimal” or “against-policy” (from a strict business standpoint) decision might bring optimal results in the long run, with people awarding trust to the hotel. So, how would it be possible for an AI to assess a situation and decide when to apply exceptions to generalized policies?
“It all comes down to augmentation.” said Ramsey, explaining that an AI, with a better fine-tuning of the response output capabilities is, in time, able to make correct decisions in a good number of instances. However, there arises a consequential problem. If exceptions to policies can be made, humans may find exploitative patterns within the functioning of a service.
According to Ramsey, in business this is a risk that has to be taken into account. “You can train models to respond in certain ways and then you need to understand how great is the loss linked to the exploitation,” he says. In other words, when exploitative patterns from users emerge, a company needs to evaluate if the effective losses are greater than the costs of dedicating humans to handle the exceptions. “Cost versus customer experience is an important factor to consider.”
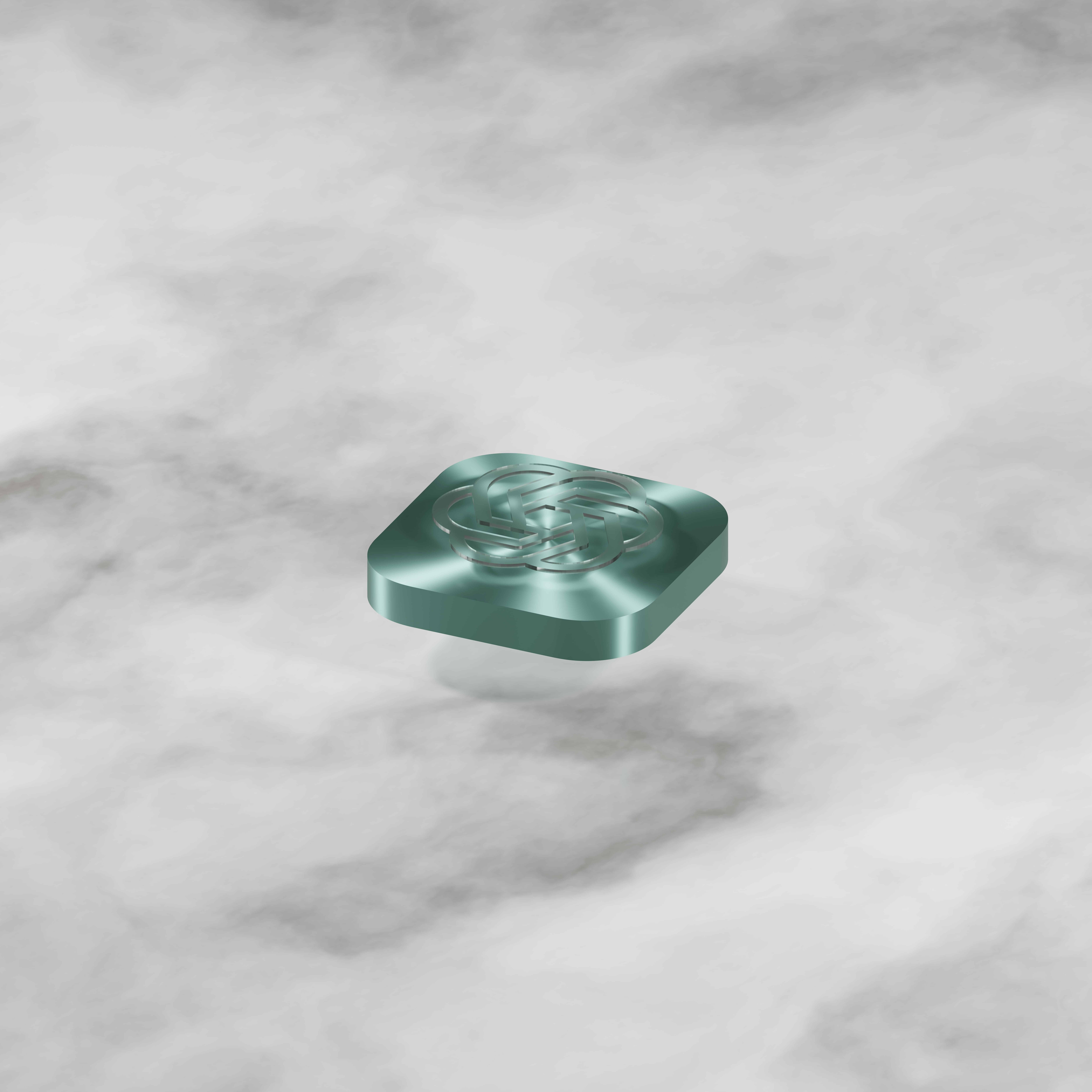
Effective governance and awareness
However, for the time being, full automation of the workflow in business that entails direct customer – worker interaction is not feasible: “Maybe around 60 percent or 70 percent could be doable.”, Ramsey continued, “There is still a need for human beings to be able to get in the loop and supervise what cannot always work with automation”. Moreover, extensive knowledge on AI systems is necessary and paramount: “We need control over the machine, in the sense that we need to always understand and know what the machine is doing.”
Hence, finally, it all goes back to legislative policies, which experts are now bringing to the centre stage of the discussion around AI, considering all of its applications. Effective governance is the name of the game; and Ramsey believes it would be wise to start implementing clear policies right now, even though we might not yet feel a sense of worrying urgency to do so. “We’re going to get to a point where people in business are going to partner with third party AIs. This leads to concerns as data will be leaving the organizations’ control. In terms of governance, we need to be proactive.”