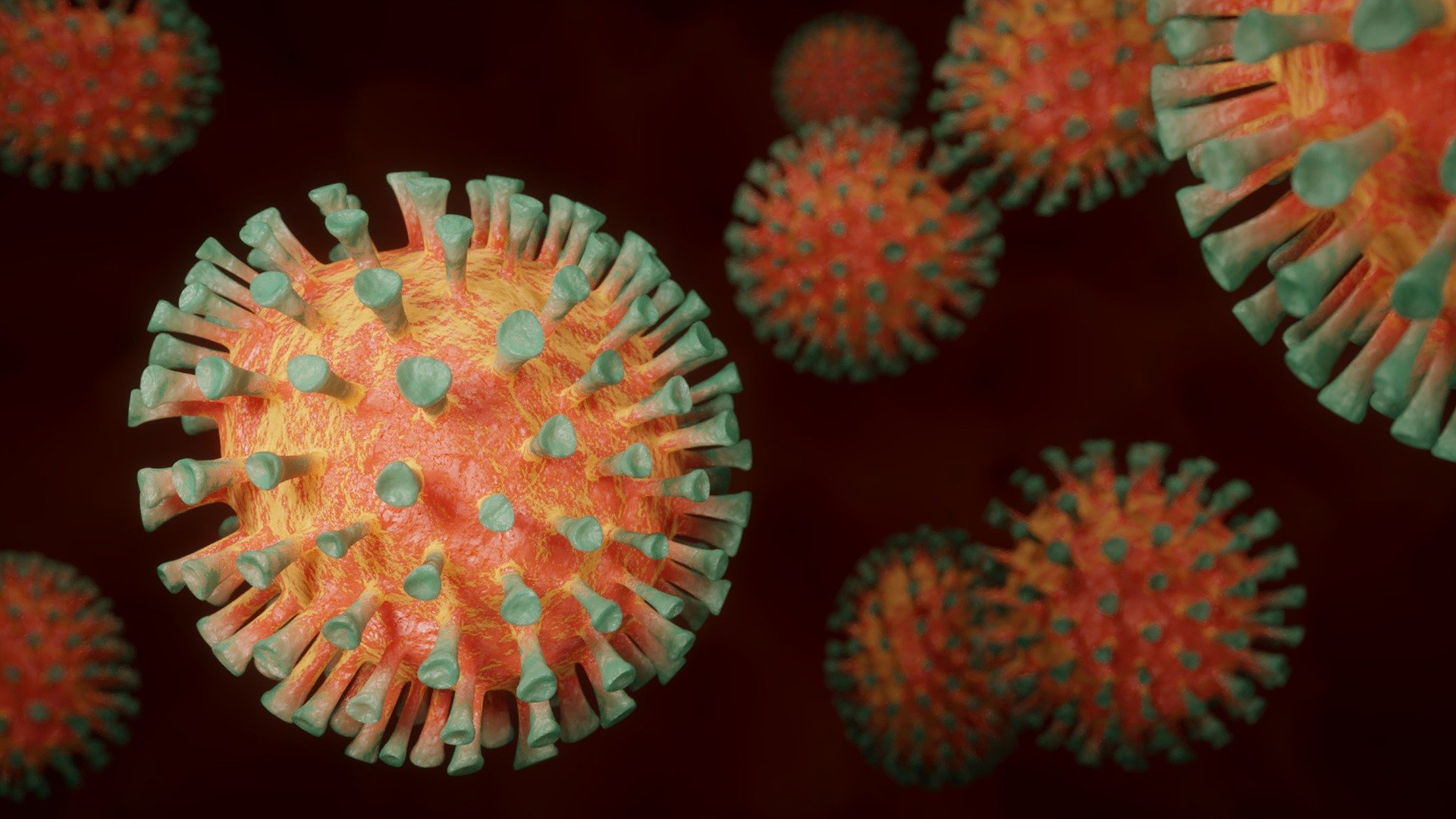
Scientists at the Gwangju Institute of Science and Technology have developed a groundbreaking tool, DeepGT, which combines a Gires-Tournois immunosensor with deep learning algorithms, offering enhanced accuracy in counting viral particles. The framework aims to provide rapid and precise diagnostics, even at low virus concentrations. DeepGT refines visual artefacts and colour deviations, offering substantial improvements over traditional rule-based algorithms. This innovation could revolutionise the early screening and triage of emerging viruses, reducing both time and cost in diagnostics. The teams findings have been published in Nano Today.
- DeepGT combines a Gires-Tournois immunosensor with deep learning to enhance virus detection and quantification accuracy.
- The Gires-Tournois immunosensor uses colorimetric sensing, while deep learning refines results, even at low virus concentrations.
- This innovation revolutionizes early virus screening, reducing time and cost for diagnostics and management.
Understanding the Gires-Tournois immunosensor
The Gires-Tournois immunosensor, or GT biosensor, is a novel tool developed for the detection of viruses, including SARS-CoV-2, the strain responsible for COVID-19. The biosensor employs a trilayered thin-film configuration and a colorimetric sensing method. In simpler terms, it uses light and colour to detect the presence of viruses. This biosensor is named after its creators, French physicists François Gires and Pierre Tournois, who first introduced the concept in the 1960s. The GT biosensor is essentially a photonic resonator that increases bioparticle visibility in bright-field microscopy, a technique that shines white light through a sample.
Bright-field microscopy, however, has limitations. It can struggle with low contrast, limited magnification, and low optical resolution. To overcome these challenges, the GT biosensor enhances visibility and maximises chromatic contrast. But the GT biosensor alone cannot accurately quantify nanoscale bioparticles. The solution? Deep learning.
The role of deep learning in viral detection
Deep learning is a subset of machine learning, a type of artificial intelligence that uses algorithms to mimic the way humans learn. In the context of virus detection, the scientists trained a deep learning algorithm, aptly named DeepGT, using optical and scanning electron micrographs of the biosensor surface.
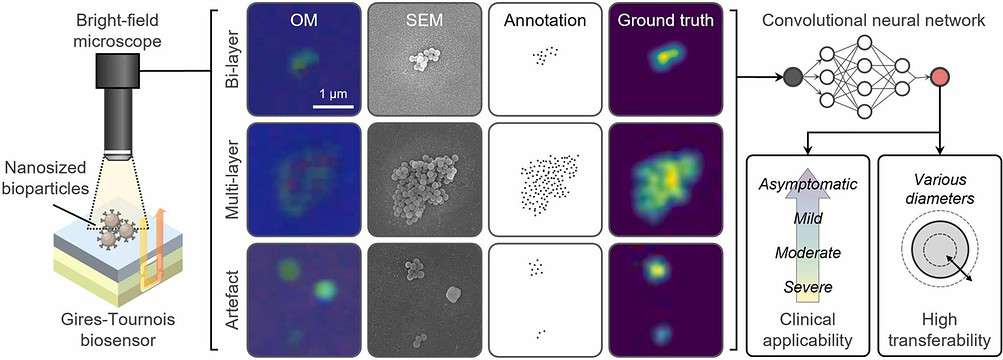
DeepGT refines artifacts and colour deviations, offering significant improvements over rule-based algorithms. The deep learning algorithm was able to accurately detect viral particles even at low concentrations, with a mean absolute error of 2.37. This is a significant improvement over traditional methods, which had a mean absolute error of 13.47. Moreover, the biosensing system can also determine the severity of the infection based on the viral load. This technology provides a practical, cost-effective solution for rapid virus detection and management.
The impact of DeepGT
Deep learning techniques have transformed the way we look at microscopy and viral detection. By combining the Gires-Tournois immunosensor with deep learning algorithms, the scientists have developed a tool that offers higher accuracy in virus detection and quantification. This is crucial for early screening, triage, and personalised treatment of viral infections, such as COVID-19.
The DeepGT platform not only offers a faster and more accurate alternative to conventional testing methods such as qPCR, but it also has the potential to detect a variety of nanosized viral pathogens, including Ebola, Zika, and monkeypox viruses. With its promising results, DeepGT could revolutionise the way we approach diagnostics and therapeutics in the fight against viral diseases.