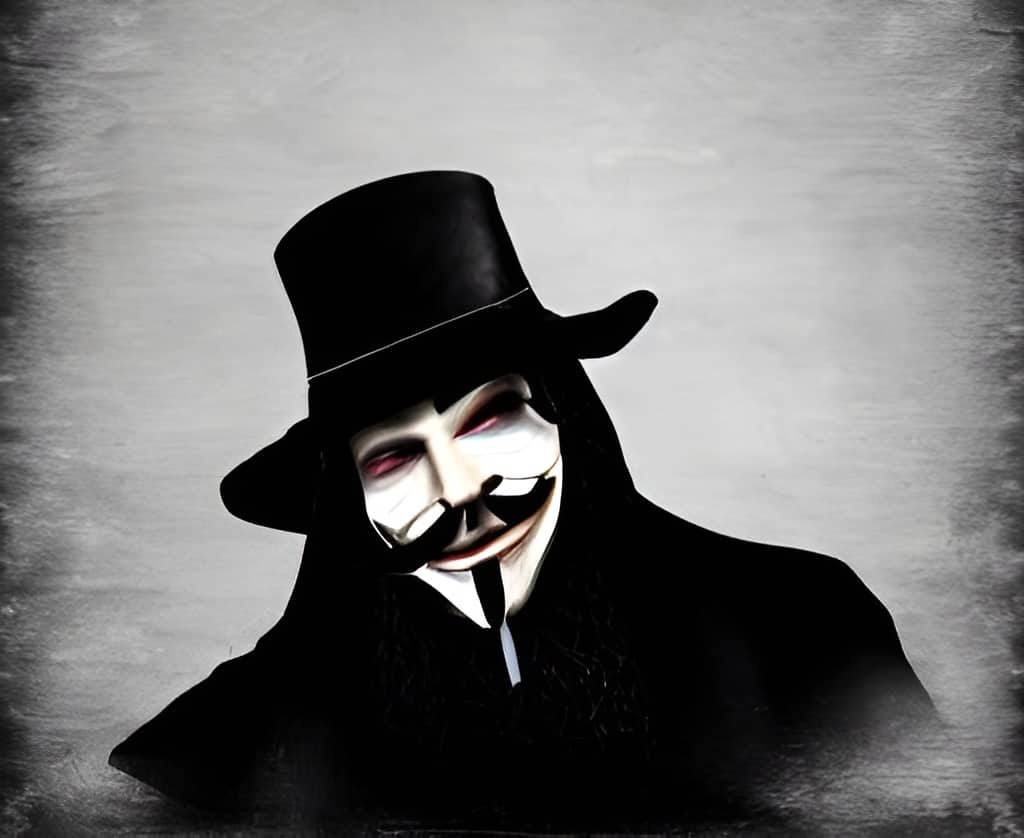
Researchers have developed DarkBERT, a groundbreaking language model specifically designed to tackle the linguistic complexities of the Dark Web. Unlike the Surface Web, the Dark Webโs language use is strikingly distinct, demanding innovative approaches to textual analysis. DarkBERT, pre-trained on Dark Web data, outshines current language models in multiple use cases, serving as a crucial resource for future Dark Web research. The model creators meticulously filtered and compiled text data to address the Dark Webโs extreme lexical and structural diversity, ensuring a proper representation of the domain.
Understanding the Dark Web Domain
The Dark Web is a subset of the internet that is not indexed by search engines, making it inaccessible without specialised applications like Tor. Often associated with illegal activities, the Dark Web has attracted significant interest from researchers and security experts seeking to understand its workings and develop cybersecurity measures. One key method employed in this field is natural language processing (NLP), which is used to gather evidence-based knowledge and generate cyber threat intelligence (CTI).
However, existing NLP models such as BERT, which are predominantly trained on Surface Web content, have limitations when it comes to Dark Web research. The linguistic characteristics of the Dark Web differ significantly from those of the Surface Web, rendering BERT-based models less effective in this domain. To address these limitations and provide a more powerful NLP tool, a team of researchers from KAIST in South Korea and S2W Inc. developed DarkBERT, a new language model pre-trained on a Dark Web corpus.
Creating the DarkBERT Model
Developing DarkBERT required a thorough understanding of the Dark Webโs linguistic landscape and the careful selection of appropriate text data. The researchers needed to filter and compile the text data used to train DarkBERT to combat the extreme lexical and structural diversity of the Dark Web that may hinder the creation of an accurate representation of the domain. In the pre-filtering researchers also made sure no privacy sensitive data was used.
Once the appropriate text data was compiled, the researchers proceeded with the pre-training of the DarkBERT model.
Evaluating DarkBERTโs Performance
In order to validate the benefits of having a Dark Web domain-specific model, the researchers compared the performance of DarkBERT with its vanilla counterpart and other widely used language models. The evaluation process demonstrated that DarkBERT outperforms current language models in detecting underground activities, making it a valuable resource for future research on the Dark Web.
DarkBERTโs superior performance in this domain can be attributed to its targeted pre-training on Dark Web data, which allows it to better understand and process the linguistic nuances unique to this environment. By providing more accurate and relevant insights, DarkBERT has the potential to greatly enhance cybersecurity measures and facilitate a deeper understanding of the Dark Webโs workings.
Implications and Future Research
DarkBERTโs development marks a significant milestone in the field of Dark Web research and cybersecurity, as it offers a more effective NLP tool tailored to the unique language use within this domain. By outperforming current language models, DarkBERT can provide valuable insights for researchers and security experts investigating the Dark Web, ultimately contributing to the development of more effective cybersecurity strategies.
As the model continues to be refined and its performance evaluated, it is likely that DarkBERT will become an indispensable resource for researchers and security professionals alike. Its success in addressing the linguistic challenges of the Dark Web not only highlights the importance of domain-specific language models but also paves the way for future advancements in this rapidly evolving field.