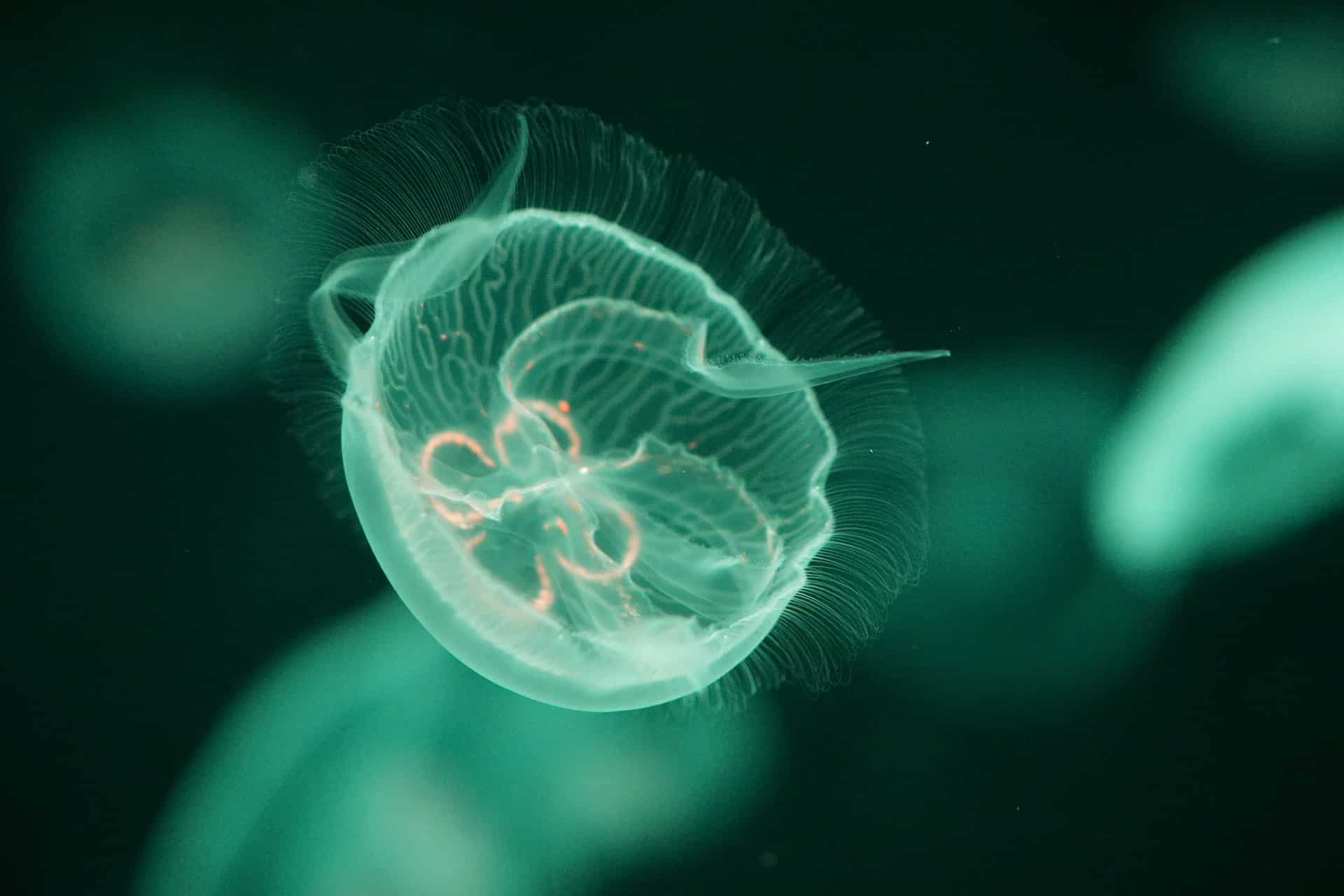
Researchers have discovered that the Caribbean box jellyfish, despite lacking a central brain, can learn through association. This cognitive capacity, previously believed to require a centralised nervous system, has been observed when the jellyfish associate bumping into something with a visual cue to avoid future collisions. The experiments carried out were designed to mimic the creature’s natural environment, resulting in biologically meaningful observations. Further study of the jellyfish’s small ‘eye-brain’ complexes, known as rhopalia, could shed light on the evolutionary origins of learning. These insights may have profound implications for non-biological systems, potentially aiding the development of pattern-recognising robots and contributing to advancements in artificial intelligence and machine learning.
- Jellyfish challenge traditional understanding of learning without a central brain, sparking evolutionary insights.
- Their decentralized neural networks offer AI inspiration for distributed learning systems and underwater robotics.
- Research into jellyfish learning mechanisms may enhance adaptable AI algorithms and expand machine learning possibilities.
Jellyfish: An unconventional study subject
Jellyfish, part of the ancient Cnidaria phylum, are among the simplest forms of life. Unlike most other animals, they lack a centralized brain and instead possess a diffuse network of neurons, known as a nerve net, dispersed throughout their bodies. This primitive nervous system, despite its simplicity, allows jellyfish to perform a variety of complex behaviours such as feeding, mating and avoiding obstacles. The Caribbean box jellyfish, a species barely a centimetre long, has recently stunned researchers by demonstrating associative learning abilities.
These findings challenge long-held views about cognition and the necessity of a brain for learning. The box jellyfish, and possibly other cnidarians such as sea anemones, are capable of associative learning, a cognitive process previously thought to require a centralized nervous system. This revelation opens up exciting new perspectives on the evolution of learning and cognition, suggesting that these capabilities might have developed much earlier in the tree of life than previously believed.
Implications for artificial intelligence and neural networks
Understanding the learning mechanisms of a creature as simple as a jellyfish could have profound implications for artificial intelligence (AI) and neural network research. The jellyfish’s decentralized nervous system, in which each ‘eye-brain’ complex, or rhopalium, can function and learn independently, offers a unique model for distributed learning systems.
The study of moon jellyfish neural networks has already provided insights into the regulation of movement and the resilience of the nervous system, even when parts of the creature are impaired. Researchers at the University of Bonn have developed a mathematical model to explore how nerve cell excitation results in the jellyfish’s efficient movements. This research could contribute to improving the autonomous control of underwater robots based on jellyfish swimming principles, and the principles could also be applied more broadly in the field of robotics.
From biological to non-biological learning systems
The study of box jellyfish learning mechanisms could also influence the development of non-biological learning systems. By understanding how a simple organism like a jellyfish learns and adapts its behaviour, we might be able to design AI systems that can learn from the environment and adapt in a similar way. This could lead to more robust and adaptable AI systems that can operate in complex and unpredictable environments.
The implications of this research extend beyond biology and into technology, particularly artificial intelligence and machine learning. The insights gained from the jellyfish’s learning mechanisms could help develop new algorithms that learn from non-traditional sources, expanding the possibilities for AI and machine learning.