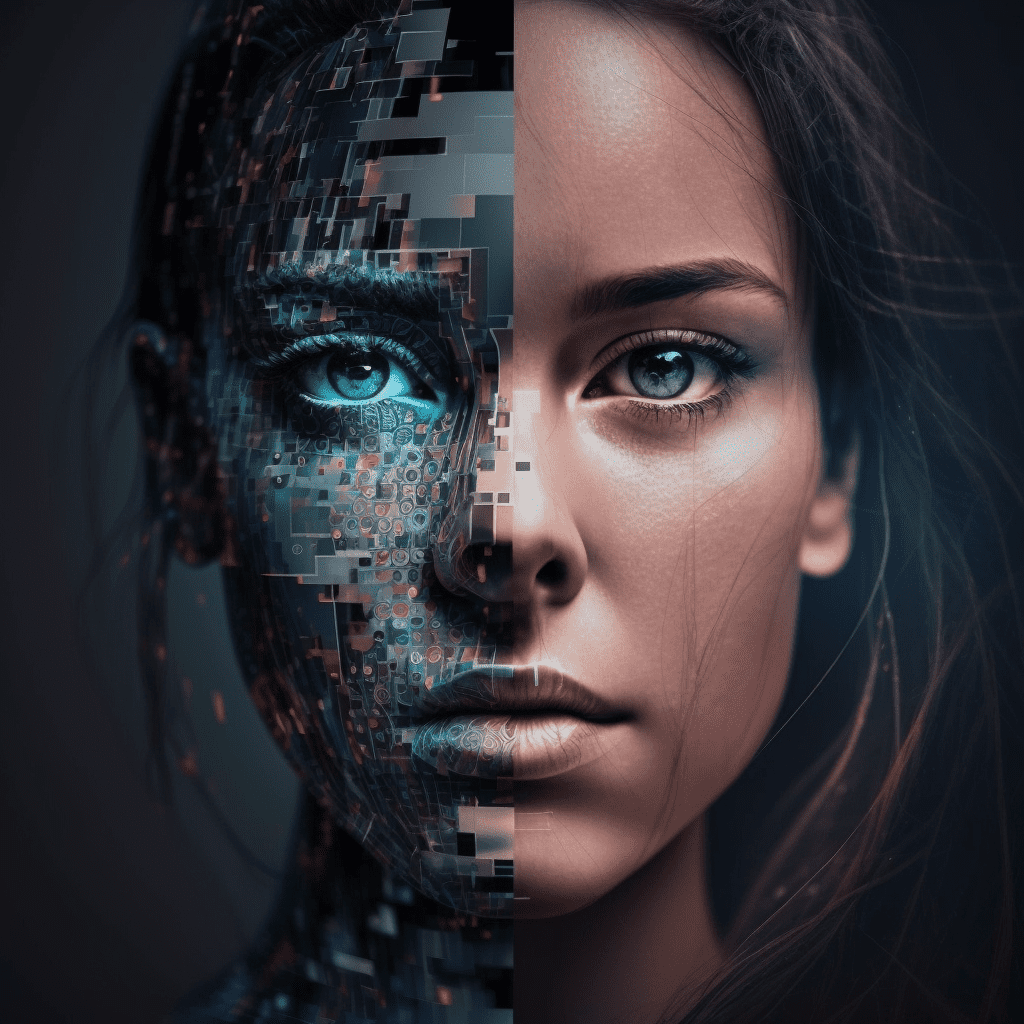
Dutch researchers have devised a groundbreaking technique, merging brain-like neurons with Forward-Propagation Through Time (FPTT), to train large-scale spiking neural networks with enhanced speed and energy efficiency. Published in Nature Machine Intelligence, their method can train networks with over 6 million neurons, expanding applications in wearable AI, speech recognition, and augmented reality. By using neuromorphic hardware chips, these networks maintain user privacy while allowing learning directly from data.
A Dutch breakthrough in neural networks
Bojian Yin, Federico Corradi, and Sander M. Bohté from the Dutch National Research Institute for Mathematics and Computer Science (CWI) have achieved a significant breakthrough in artificial intelligence. Collaborating with researchers from Eindhoven University of Technology and research partner Holst Centre, the team has demonstrated a novel approach to training large-scale spiking neural networks by combining brain-like neurons with Forward-Propagation Through Time (FPTT).
Spiking neural networks mimic the exchange of electrical pulses in the brain. These networks, implemented in chips known as neuromorphic hardware, bring AI programs directly to users’ devices while maintaining privacy. This is particularly relevant in speech recognition for toys and appliances, as well as local surveillance.
Overcoming limitations in neural networks
Traditional artificial neural networks, while being the backbone of the current AI revolution, face limitations in terms of efficiency, memory requirements, and learning capabilities compared to the human brain. Spiking neural networks, on the other hand, more closely mimic the properties of biological neurons, offering benefits in privacy, robustness, and responsiveness. These networks communicate by exchanging electrical pulses, similar to the neurons in our nervous system, and do so sparingly, resulting in more energy efficiency.
However, the learning aspect of these networks – adapting and adjusting to new information – poses significant challenges. The researchers aimed to address these limitations and develop a system that better mirrors the way the human brain learns, processing and adapting to new information in real-time. The FPTT learning method combined with novel liquid time-constant spiking neurons resolves these limitations, enabling online learning of exceedingly long sequences while outperforming current online methods and approaching or outperforming offline methods on temporal classification tasks.
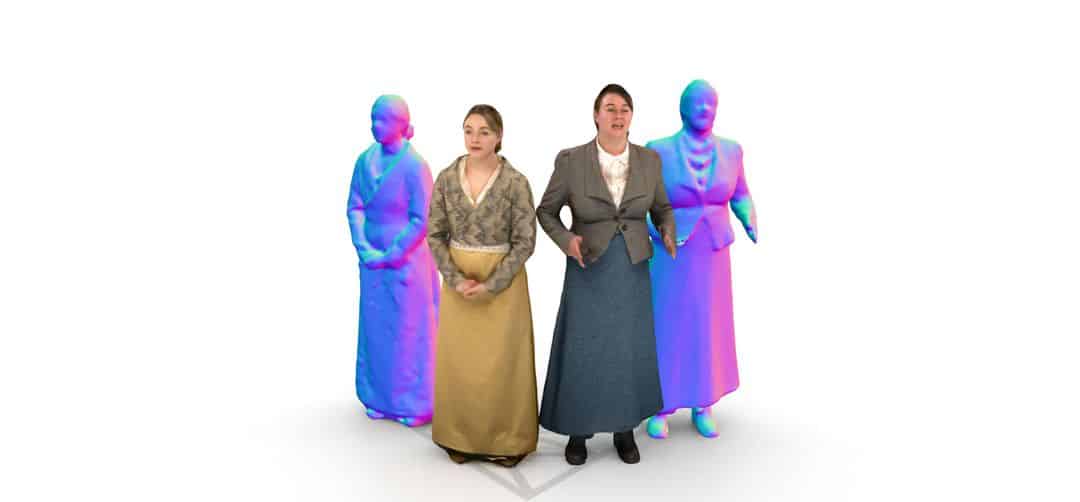
The research by Bohté and his team demonstrates that neural networks could previously only be trained with up to 10,000 neurons. However, with this new approach, networks can be trained with more than 6 million neurons, such as the SPYv4. The team’s goal was to develop something closer to the way our brain learns, adapting and learning in real-time without storing and processing all previous information.
Speed and energy efficiency in real-life applications
By combining brain-like neurons and FPTT for faster neural networks, researchers have opened the door to a wide array of potential applications, including wearable AI, speech recognition, and augmented reality. This new approach allows for much larger spiking neural networks to be created, resulting in improved speed and energy efficiency.
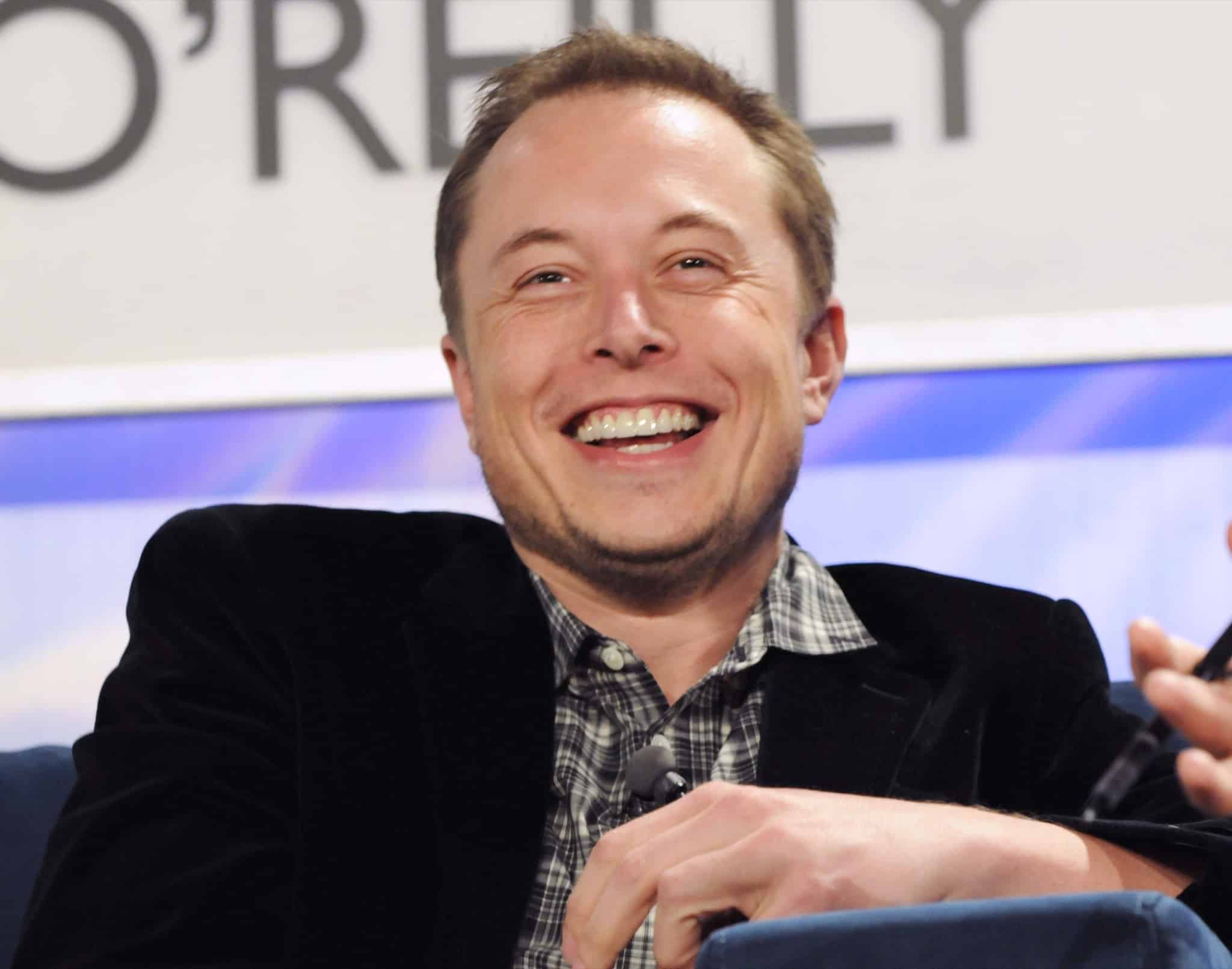
Furthermore, the use of neuromorphic hardware chips enables these programs to run at very low power while maintaining user privacy. This aspect is especially important for speech recognition applications in toys and appliances, as well as local surveillance systems. The algorithm’s efficiency and robustness also make it possible to directly train deep and performant spiking neural networks for joint object localization and recognition, demonstrating the ability to train large-scale dynamic and complex spiking neural network architectures.
The future of neural networks
As researchers continue to explore the potential of combining brain-like neurons and FPTT for faster neural networks, it’s clear that this innovative approach has significantly improved speed and energy efficiency in AI systems. Industries such as healthcare and transportation could greatly benefit from these advancements, making machine learning more efficient and energy-efficient in a wide range of applications.
While quantitative data or comparisons illustrating the improvement in speed and performance achieved by this new approach compared to traditional neural networks are not readily available, the research’s implications for the future of AI are undeniable. This Dutch breakthrough could pave the way for more advanced, efficient, and powerful artificial intelligence systems, transforming industries and our daily lives alike.