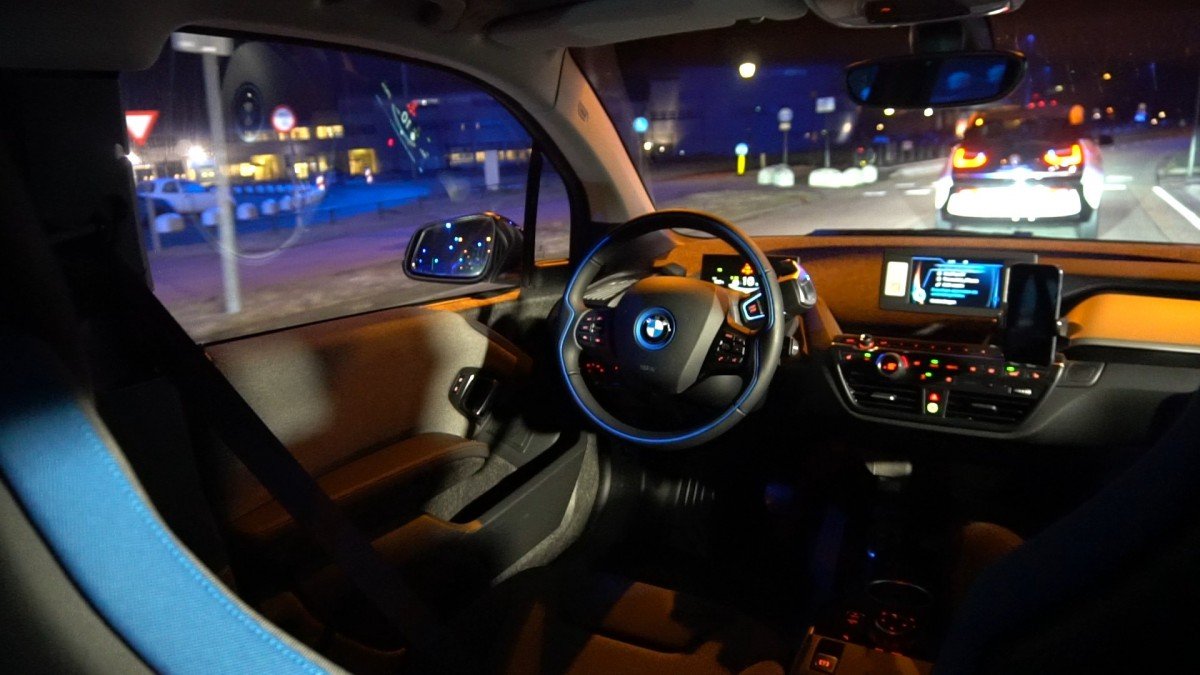
Dutch and Spanish computer scientists have discovered how systems that use artificial intelligence (AI) learn in practice. In many systems that are based on so-called ‘deep learning’, it was not clear how that learning process actually took place. The researchers have now managed to figure out how an image recognition system learns about its environment. Then they simplified that learning system by forcing it to focus on less important information as well. AI systems for image recognition are of great importance for autonomous driving cars, for a start.
The systems in question, according to a press release from the University of Groningen (RUG), are Convolutional Neural Networks (CCNs). These are a biology-inspired form of deep learning in AI. This system learns to recognize images thanks to the interaction of thousands of ‘neurons’. These mimic the workings of the brain. How these CNNs work was not understood up until now, says Estefania Talavera Martinez. She is a lecturer-researcher at the Bernoulli Institute for Mathematics, Computer Science and Artificial Intelligence at the RUG.
Food
She herself makes use of CNNs in her research on human behavior and uses them to analyze images taken with a handheld camera. This is how she carries out studies on how people react to food. She therefore wanted the system to be able to recognize different situations in which people come into contact with food. ” In the process, I noticed that the system made errors when it came to properly identifying the environment in certain pictures, and I wanted to know why that happened.”
Using heat maps, she analyzed which parts of the images were used by the CNNs to recognize the situation. “That led to the hypothesis that the system was not using enough details from the image,” she explains. For example, if an AI system has learned to associate a mug with kitchens, it will misclassify a living room or office, where mugs are also used. The solution Talavera Martinez came up with for this, along with her Spanish colleagues David Morales (University of Granada) and Beatriz Remeseiro (University of Oviedo), was to distract the system from its primary targets.
Blurry images
They trained CNNs using standard images of aircraft or cars. By using heat maps, they were able to work out which parts had been used for classification. They then blurred these parts of the image, which was followed by a second round of training. “This forces the system to use other parts of the image in order to recognise things. And by including this additional information, a better classification has been created.” This new training method is much simpler, according to the researchers, and takes less computational time as well.
Main photo: For autonomous driving cars, a perfectly working image recognition system is indispensable
The research was published in the scientific journal Neural Computing and Applications.
Also interesting: AI discovers what makes the sound of a Stradivari
Read our dossier on artificial intelligence.