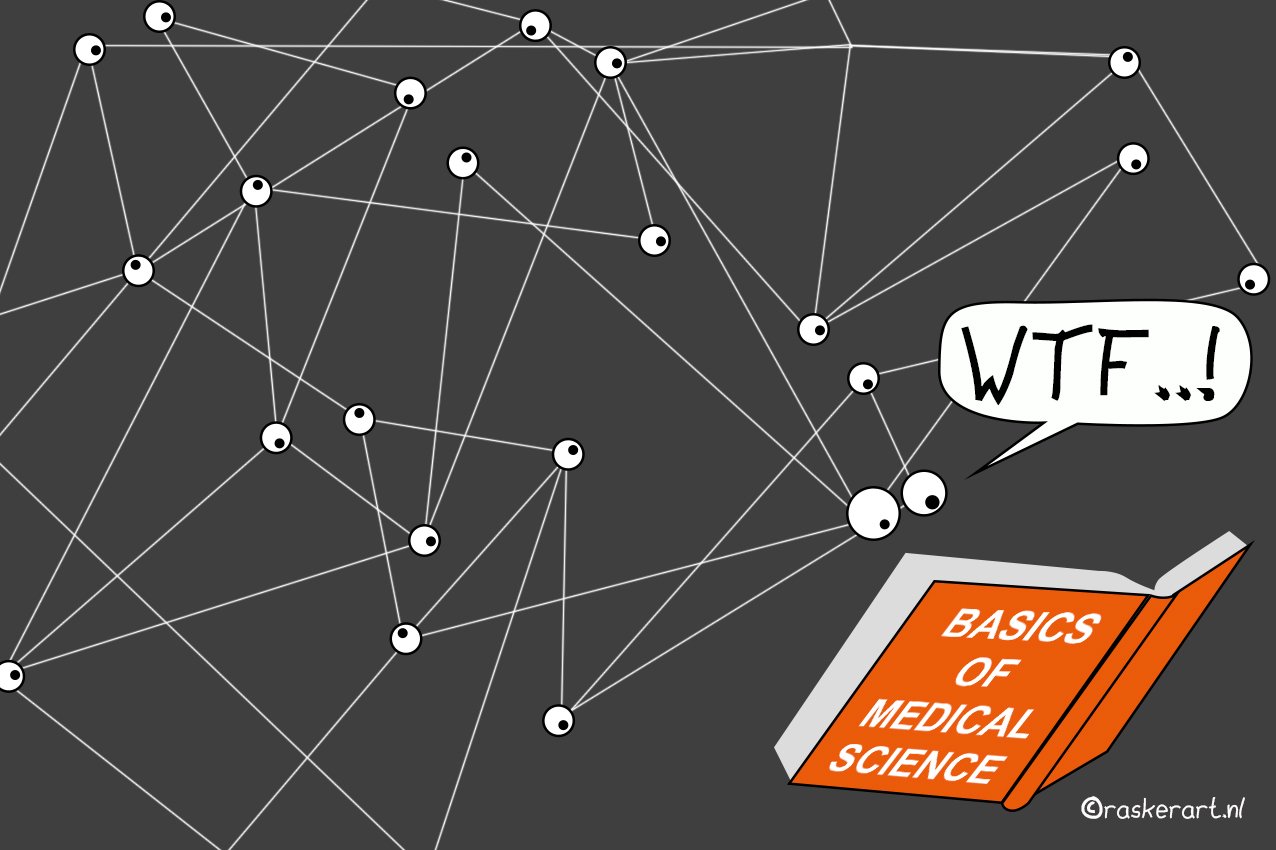
All around us we see AI applications becoming more and more common. Recommendations from Spotify and Netflix, virtual assistants who arrange everything for you or watches that wake you up once you are fully rested. The use of AI in healthcare has also increased in recent years. Last week we wrote about an algorithm that can recognize COVID-19 on the basis of lung scans.
Yet only 16 percent of European healthcare institutions use some form of AI. That is why IO is talking to three AI experts in healthcare here in the Netherlands to find out why healthcare is lagging behind other sectors and what is needed to change this.
Esmee Stoop is a data scientist at the Leiden University Medical Center (LUMC) and as a technician, she teaches doctors and nurses about AI. Marieke van Buchem is a Ph.D. student at LUMC and is researching a variety of AI applications. She is also connected to the CAIRELab, where she and the team there pool AI and clinical knowledge. We also get to speak with Cristina González-Gonzalo. She is doing her final year of Ph.D. research at A-eye Research and the Radboudumc. Among other things, she developed an algorithm that shows doctors how an AI system arrives at a diagnosis.
Algorithms are not going to replace doctors
First of all, algorithms are not going to take over the work of doctors. All three scientists agree on that. González-Gonzalo has to laugh: “That’s often a fear of doctors, but I don’t see that happening any time soon. What’s more, no one wants to hear from a robot that they have cancer; the relationship between patient and doctor remains important. AI is going to make the work of doctors easier. They will have more time for complex cases that way.”
Van Buchem also agrees with this: “A doctor looks at the whole picture. They have wisdom and experience. An algorithm can support them in that, but it’s not magic. Yes, they are complex sums that recognize patterns that we don’t see. But a neural network can’t read a medical book. That kind of human expertise remains indispensable.”
Stoop offers an example straightaway of what this support for young doctors will look like: “Novice radiologists who have doubts about a diagnosis can run scans through a database. They are then shown similar images to compare with the scan they have doubts about. They can learn from this without taking any expertise away from themselves. The algorithm is a buddy and not a supervisor who takes over everything.”
AI can take over repetitive tasks
By using AI across a range of areas of healthcare, around 1.8 billion hours can be saved each year in Europe. That represents about 500 thousand full-time jobs. An enormous amount for a sector that is suffering from a structural shortage. According to van Buchem, healthcare workers sometimes spend as much as 40 percent of their time on administrative tasks. “Writing up a patient report is something that keeps on coming back. AI can easily take over this task. We are now developing a model that gives a kind of preliminary outline for a report. We record conversations and train the model with speech recognition in order to do this. Right now, this takes a lot of time because you have to point out everything manually, but in the end, it will save time. In the US, where they already use it, this saves a doctor a few hours per week,” Van Buchem explains.
And there are even more advantages to this kind of automation: “The current system can make doctors think in terms of pigeonholes. They have to enter almost everything into checklists. Healthcare is very complex. Making a diagnosis or treatment plan involves more than just filling in boxes. This removes any nuance. But if you leave that pigeonholing to an AI, the doctor can focus on a patient again and bring back those nuances.”
What else is needed for AI systems in healthcare?
There is no lack of research and projects here. But what are AI systems missing to be able to make a real breakthrough in hospitals? All three data experts know for certain that this starts with objective datasets. Van Buchem: “Datasets are not always objective. If a doctor has a patient’s blood tested in a lab, they do so with a diagnosis in mind. So these data are already saying something and won’t necessarily present an objective picture. That’s why it’s good to always be critical and ask yourself in advance why specific data has been collected.”
Scans already provide a more objective image, says Stoop. “Such a scan is a direct representation of an organ, for example. You can let AI models do all sorts of things with it. But there is no gold standard. If you ask ten radiologists what they see, they won’t all say the same thing.”
Push AI to keep on searching
It should also be possible to determine these various findings by doctors with an AI model. But at the moment, an algorithm stops searching once it has pinpointed just enough information to arrive at a diagnosis. When in fact you want the system to keep on searching, just like a radiologist does in order to get a more complete picture.
González-Gonzalo has come up with a solution: “At present, the task of an Al is completed when the system recognizes a disease. While it’s often the case that it’s not so black and white. But by pushing the system to look further, several other areas can be tapped into. This provides you with more insight,” she goes on to explain. The system can indicate – with a percentage for example – how certain the AI is of the diagnosis. “Doctors will soon see whether additional examinations are needed or whether they need to double-check something,” according to González-Gonzalo.
Transparent and easy-to-explain models
AI will only really take off if models are capable of explaining how they come to a decision. This so-called black box has already opened up for simpler algorithms. Van Buchem: “A lot of people think that AI is incapable of explaining itself. But there are algorithms that can do this perfectly well. For instance, in the IC, AI specifies which values it uses to determine the duration of a stay in hospital.”
The model that González-Gonzalo developed to recognize eye diseases on scans also includes doctors in the decision-making process. “The system returns from the output to the input via various images. In these pictures, doctors can see what the algorithm has paid attention to in order to reach a conclusion.”
But more complex AI can make this process less transparent, Stoop acknowledges. “A complicated algorithm makes an incredible number of different connections. So many relationships that we as humans can’t even see”. An example of one such complex algorithm attempts to diagnose hereditary eye diseases by linking genetic data to eye scans.
González-Gonzalo: “We train a dataset in various ways to link the gene associated with a hereditary disease to an eye scan,” explains González-Gonzalo. How a system manages to make such a link is almost impossible for people to understand. Nevertheless, we are trying to think of a way in which AI systems may be able to make such a link understandable. This will give healthcare workers more confidence in how AI works. Which will also help towards the wider application of AI in healthcare.”