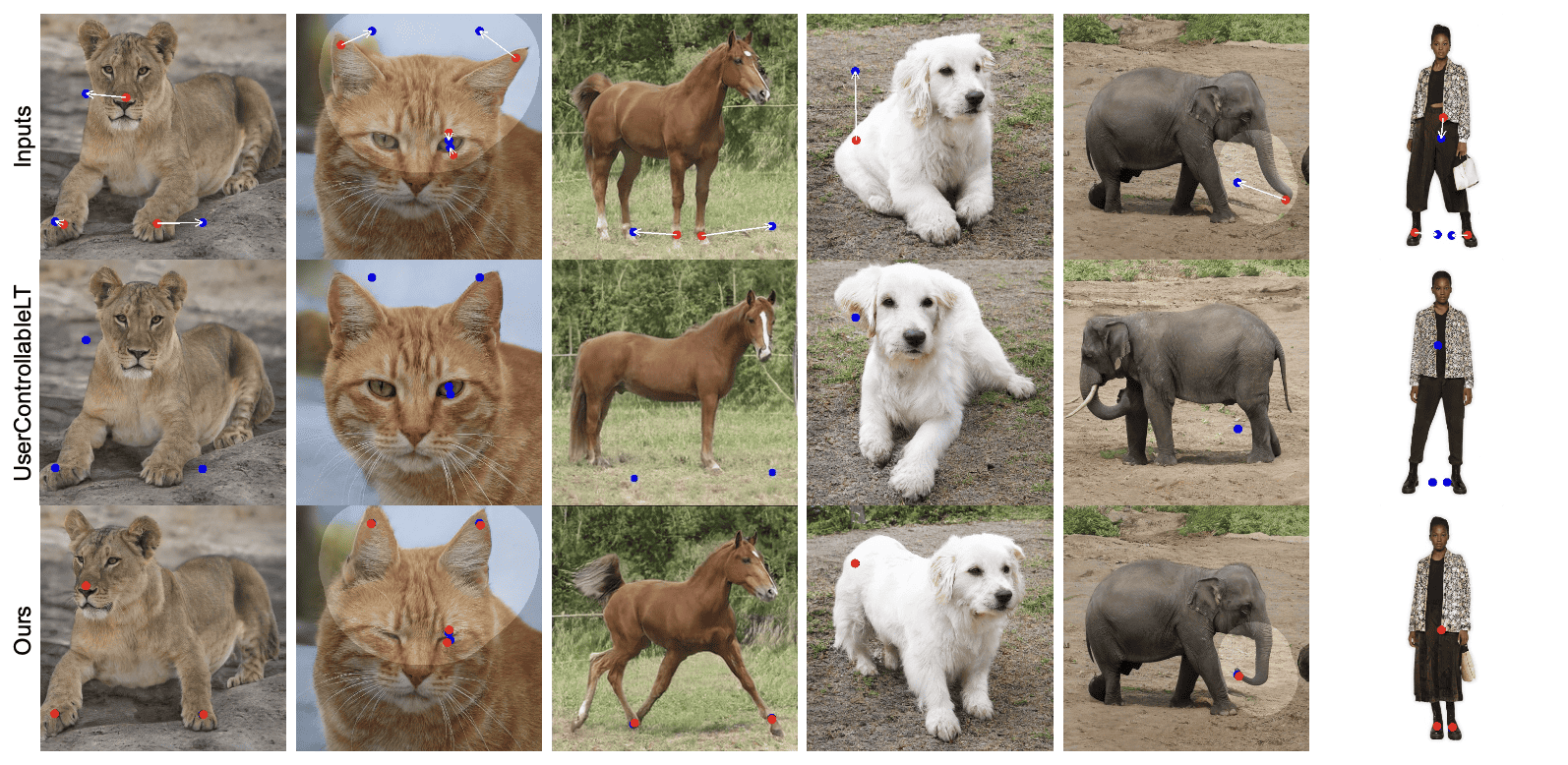
For those still recovering from what Midjourney and Dall-E’s AI-supported image generation brought us, there’s some disturbing news: we haven’t reached the limits of artificial intelligence yet. The ability of AI tools to manipulate images continues to grow. The latest example is shown in a research paper from the Max Planck Institute. For now, it’s “only” a research paper, but a very impressive one, letting users drag elements of a picture to change their appearance.
If you don’t get the picture yet, here’s an example:
Not only can you change the dimensions of a car or manipulate a smile into a frown with a simple click and drag, but you can rotate a picture’s subject as if it were a 3D model — changing the direction someone is facing, for example. Another option is to adjust the reflections on a lake or the height of a mountain with a few clicks.
DragGAN
Creating visual content that fits user needs often requires precise and flexible control over attributes such as pose, shape, expression, and layout of the generated objects. Traditional methods for controlling Generative Adversarial Networks (GANs) rely on manually annotated data or prior 3D models. However, these approaches often lack precision, flexibility, and generality. In response to these shortcomings, Max Planck Institute introduces DragGAN, a novel approach that allows users to interactively “drag” any points in an image to target locations.
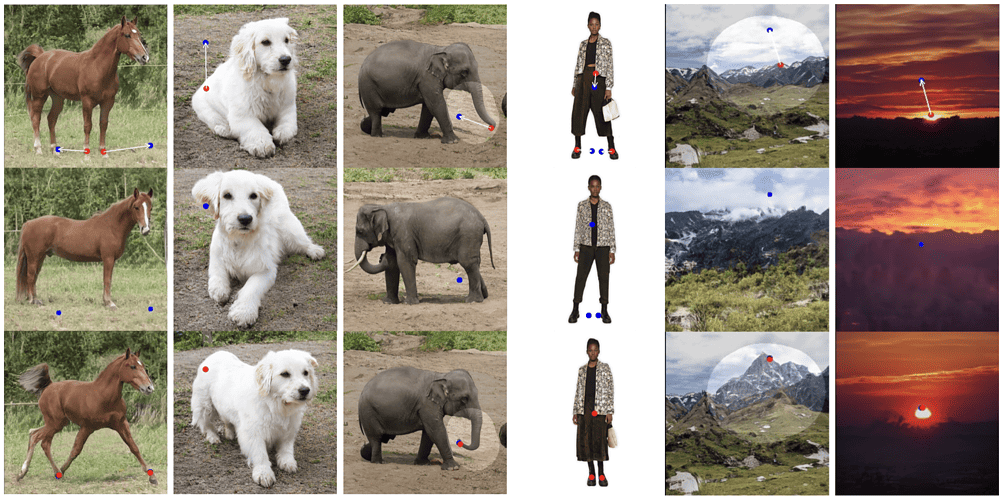
DragGAN comprises two main components: a feature-based motion supervision and a new point-tracking approach. The motion supervision allows for user-guided movement of handle points in the image towards target positions. The point-tracking approach leverages distinctive generator features to keep track of the handle points’ locations as they are moved. As a result, users can deform images with precision, influencing attributes such as pose, shape, expression, and layout across various categories like animals, cars, humans, and landscapes.
Flexible, precise, generic
This method presents a more flexible, precise, and generic way of controlling GANs, allowing users to select any number of handle points and corresponding target points on an image. The goal is to move these handle points to reach their respective targets. This approach allows the user to control a range of spatial attributes, regardless of object categories. This sets DragGAN apart from previous methods that often fail to generalize to new object categories or offer limited control over spatial attributes.
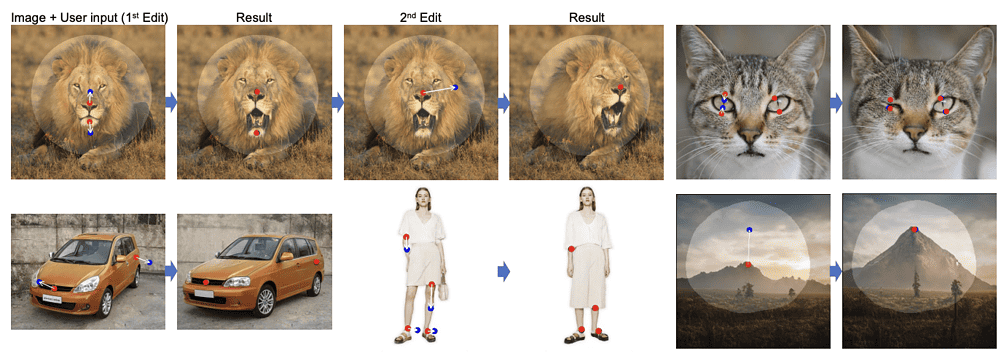
The approach offered by DragGAN does not rely on any other networks like RAFT, making it efficient for manipulation tasks. The researchers claim it takes only a few seconds on an RTX 3090 GPU in most cases. This allows for live, interactive editing sessions, enabling quick iteration of different layouts until the desired output is achieved.
Through an extensive evaluation of diverse datasets, DragGAN has demonstrated its capability to move user-defined handle points to target locations, achieving diverse manipulation effects across many object categories. A key feature of DragGAN is its capacity for user input of a binary mask, indicating the movable region in an image. This allows for more nuanced control of manipulations, helping reduce ambiguity and maintain certain regions fixed.
Limitations and misuse
However, the researchers also warn DragGAN has its limitations. Although it does possess some extrapolation capabilities, the quality of editing can still be affected by the diversity of training data. Moreover, handle points in texture-less regions sometimes suffer from drift in tracking. Despite these limitations, DragGAN offers an effective, interactive approach to image editing.
The paper’s authors note the potential for misuse of the technology, as it can be used to create images of a real person with a fake pose, expression, or shape. They stress the importance of respecting personality rights and privacy regulations in any application of their approach.
In conclusion, DragGAN represents a significant advancement in the field of GAN-based image manipulation. The method utilizes a pre-trained GAN to generate images that closely follow user input while remaining realistic. By using an optimization of latent codes and a point tracking procedure, DragGAN provides pixel-precise image deformations and interactive performance. The authors hope to extend this point-based editing to 3D generative models in the future.