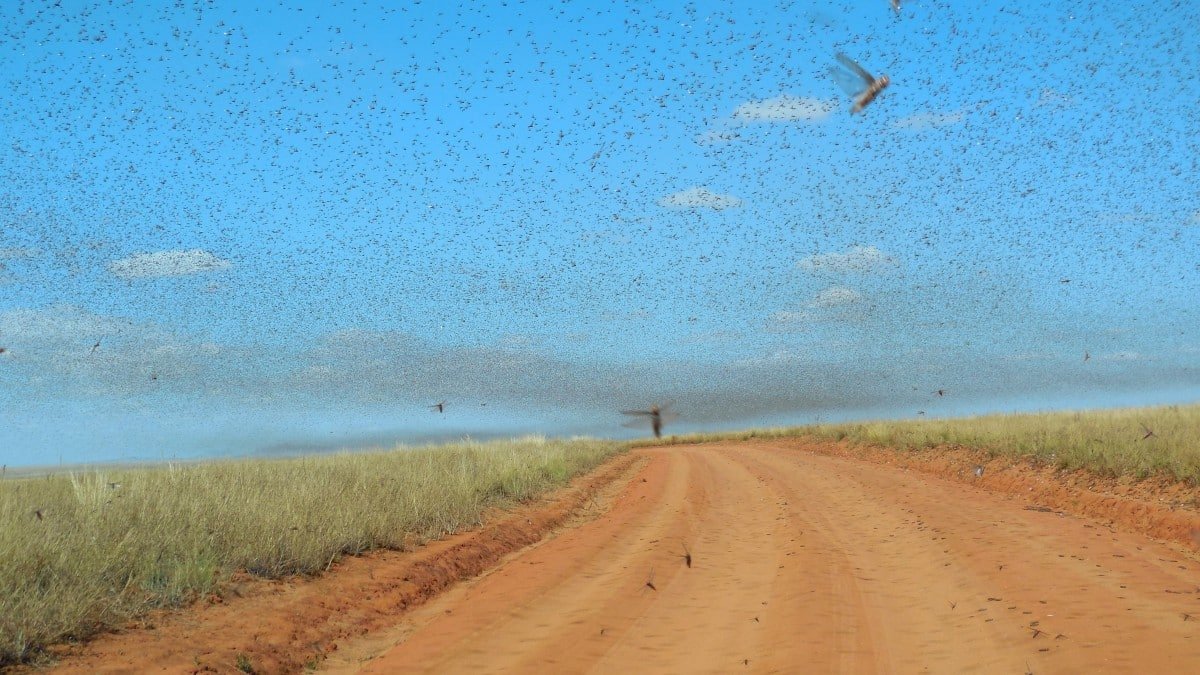
Physicists at the University of Innsbruck (Austria) are investigating the swarm behaviour of living beings using artificial intelligence. Previously, this was done with algorithms that were based on assumptions.
Artificial intelligence enables an unbiased perspective on the swarm behaviour of living beings. The new approach doesn’t simply show a pre-programmed reaction to an event. It can individually adapt its behaviour over time to previous experiences. “If a decision turns out to be correct afterwards, the machine is rewarded and next time is inclined to make this decision again in the same situation,” says Katja Ried, a member of the project team, explaining the approach from machine learning. She is conducting research together with the quantum physicist Hans Briegel from the Institute of Theoretical Physics at the University of Innsbruck and Thomas Müller from the Department of Philosophy at the University of Konstanz (Germany).
Projective simulation
Briegel has already developed the projective simulation learning model used in previous research projects and tested it in various areas – for example in the search for optimized experiments in quantum laboratories and the design of creative machines. In this study, the method was applied to migratory locusts. A single swarm can consist of more than a billion animals and cause considerable economic and humanitarian damage in the affected countries.
Migratory locusts swarm out to find new food again and again. These collective movements were simulated in the algorithms. The empirics provide experimental studies with locusts.
Learning ability of locusts
To what extent locusts can learn or adapt their behaviour in the swarm is still unclear. Other swarm animals, such as honey bees, have a distinct ability to learn. These were successfully trained in the laboratory to approach flowers of different colours at different times of the day. If one models this behaviour adaptation with the help of the learning agents, the information processing of bees can be reconstructed.
The model can also be transferred to robots. If a swarm of robots is sent off with a certain target, its behaviour can be simulated and trained in the model.
“Models of learning agents could greatly improve our understanding of the origins of collective movements,” said Briegel.
Interdisciplinary approach
In the current research project, the new model is now to be combined with findings from biology and ecology to investigate collective movement in more complex environments. The aim is to jointly investigate fundamental questions about the individual behaviour of grasshoppers and their causes in the laboratory. For example, it will be possible to explain how a grasshopper behaves when it is touched by another grasshopper on its side or when other grasshoppers move in a certain way in its environment, explains Ried. These findings lead to the refinement of the theoretical model.
The method for modelling collective movements was published in the journal PloS one: Modelling collective motion based on the principle of agency: General framework and the case of marching locusts
